Advancements in Protein Folding Prediction and Their Impact
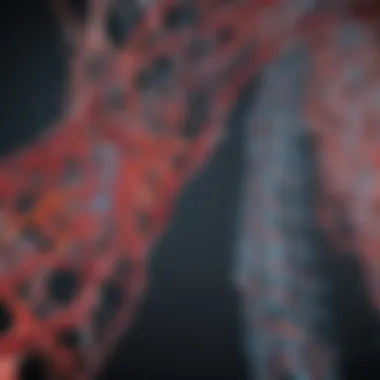
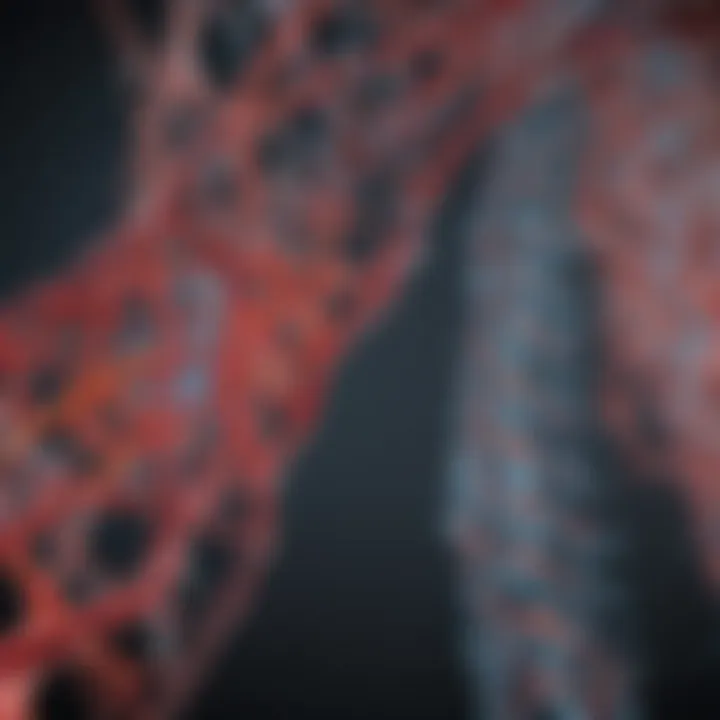
Intro
The discipline of protein folding prediction garners attention for its critical role in understanding biological processes. Proteins, being essential biomolecules, must fold into specific three-dimensional structures to carry out their functions. Abnormalities in protein folding can lead to numerous diseases, underlining the importance of accurate prediction methods.
Recent advancements in computational biology have enabled scientists to harness different methodologies for predicting how proteins will fold. These developments not only illuminate the mechanisms behind protein behavior but also pave the way for innovative applications in healthcare, biotechnology, and pharmacology. This article aims to explore the advancements in protein folding prediction, emphasizing its implications for various fields and the future directions necessary for addressing existing challenges.
Prelude to Protein Folding
Protein folding is a fundamental process in molecular biology, where a polypeptide chain folds into its functional three-dimensional structure. This structure is crucial for the protein's functionality, affecting various biological processes. Understanding how proteins fold is essential for multiple scientific disciplines, including biochemistry, genetics, and medicine. The implications of accurate protein folding prediction extend beyond academic curiosity; they touch upon practical applications in drug design, disease treatment, and synthetic biology.
Definition and Importance
The term protein folding refers to the physical process by which a linear chain of amino acids folds into a specific shape. This shape is determined by various factors, such as the sequence of amino acids and environmental conditions. Proteins are not functional in their linear form. Instead, they need to fold properly. When they do not fold correctly, they can lead to diseases such as Alzheimer's or cystic fibrosis. Therefore, predicting how proteins will fold is vital in both research and clinical settings. Accurate folding predictions can assist in the design of new drugs, targeting specific proteins that contribute to disease. Moreover, this understanding can pave the way for novel biotechnological applications, which can lead to significant advancements in various industries.
Historical Perspectives
The study of protein folding began long before sophisticated computational methods emerged. Early work in the 1950s set the stage for understanding tertiary structures. Scientists like Linus Pauling made groundbreaking contributions, helping to unveil the secondary structures of proteins, such as alpha-helices and beta-sheets. In the following decades, advancements in X-ray crystallography allowed researchers to visualize the folded structures of proteins directly for the first time.
As technology progressed, so did models of protein folding. The emergence of computational biology in the late 20th century transformed the field. Researchers began to employ various algorithmic approaches to simulate protein folding. This shift produced a deeper understanding of protein dynamics and structure formation. These historical milestones showcase the evolution of protein folding research, revealing a journey filled with discovery and innovation. Looking back, the initial studies paved the way for a future that incorporates both experimental and computational approaches to unravel the complexities of protein folding.
The Mechanism of Protein Folding
Protein folding is a fundamental process that involves the transition of a polypeptide chain from a linear structure into a three-dimensional form. Understanding this mechanism is essential because the functionality of a protein is intrinsically linked to its folded shape. Misfolded proteins can lead to a variety of diseases, such as Alzheimer's or Parkinson's, which makes this area of study vital in both health and disease contexts. Advances in our understanding of protein folding mechanisms open the door to better therapeutic options and enhance our grasp on biological processes.
Primary to Tertiary Structure
The protein structure is typically described in four levels: primary, secondary, tertiary, and quaternary. The primary structure refers to the sequence of amino acids. Once synthesized, the polypeptide undergoes folding, driven by various interactions at the molecular level.
The secondary structure involves local folding into shapes like alpha helices and beta sheets due to hydrogen bonding. However, the tertiary structure represents the overall 3D arrangement, determined by interactions among the side chains of amino acids. These interactions include ionic bonds, hydrophobic interactions, and van der Waals forces. The transition from secondary to tertiary structure is complex and influenced by many factors, including pH, temperature, and the presence of chaperones, which assist in the folding process.
Understanding how these structures form is essential for predicting how proteins behave in biological systems and offers valuable insights for the development of drugs.
Key Factors in Folding
Several key factors influence the protein folding process:
- Hydrophobic Effects: Non-polar regions of amino acids clump together to avoid water, driving the folding towards a more energetically favorable state.
- Chaperone Proteins: These proteins assist in the proper folding of other proteins, preventing aggregation and misfolding.
- Post-translational Modifications: Chemical modifications after protein synthesis can affect folding and stability, impacting function.
- Temperature and pH Levels: Both can influence the stability of different folding states, often leading to denaturation if conditions are extreme.
- Genetic Factors: Mutations in the genetic code can lead to changes in the amino acid sequence, which may disrupt proper folding.
"Protein folding is not just a problem of form but a question of functionality. Many diseases can be traced back to misfolded proteins that fail to perform their intended roles."
Addressing these factors in research can significantly enhance understanding and prediction of protein structures, ultimately facilitating applications across drug discovery and disease treatment regimes.
Significance of Accurate Prediction
The accurate prediction of protein folding is a critical endeavor within molecular biology and biochemistry. Understanding how proteins fold impacts various disciplines, including drug discovery, disease understanding, and biotechnology innovations. Each of these areas benefits from improved predictive accuracy, leading to significant advancements.
Implications in Drug Discovery
In the realm of drug discovery, protein folding prediction plays an essential role. The structure of proteins dictates their function, and many diseases result from misfolded proteins. Targeting these proteins accurately can lead to the development of better therapeutic agents.
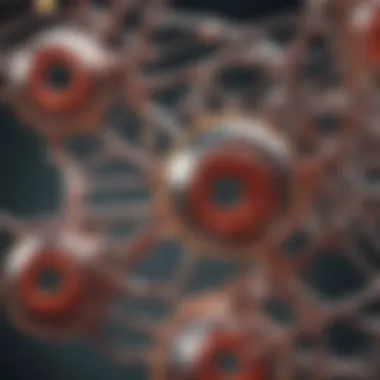
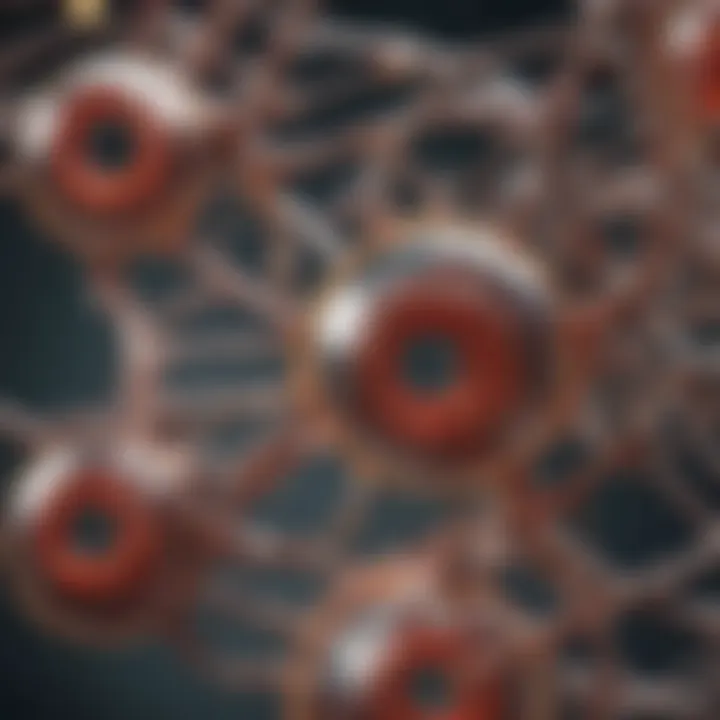
- Identifying Targets: Accurate predictions allow researchers to identify specific protein targets for drug development. This helps streamline the process of finding effective treatments for diseases like cancer or Alzheimer's.
- Antibody Development: For therapeutic antibodies, knowing the precise folding of the target protein can aid in designing antibodies that can effectively bind to the intended target, enhancing treatment efficacy.
- Reducing Costs: Predictive accuracy reduces the time and cost associated with trial-and-error approaches in drug development. By knowing how a protein is likely to fold, scientists can make informed decisions early in the development process.
- Tailored Medicines: The ability to predict protein structure allows for personalized medicine approaches, where treatment can be customized according to an individual's protein expression profiles.
Role in Understanding Diseases
Accurate protein folding predictions have profound implications for understanding diseases. Misfolded proteins are often at the core of pathological conditions, so comprehending their structure can illuminate the mechanisms of various diseases.
- Pathogenesis Insight: Accurate predictions help uncover how specific folding patterns can lead to disease states. For example, in neurodegenerative diseases, like Parkinson's, understanding how proteins misfold can reveal crucial insights into disease onset and progression.
- Biomarker Identification: Proteins often serve as biomarkers for diseases. Accurate predictions can assist in identifying which proteins may serve as reliable markers, thus aiding in early diagnosis and treatment strategies.
- Therapeutic Strategies: Understanding the structures of misfolded proteins can lead to the development of therapies aimed at correcting these misfolds or preventing their formation altogether.
In summary, the significance of accurate protein folding predictions cannot be overstated. It plays a transformative role in modern molecular biology, particularly in drug discovery and disease understanding. As research progresses, the integration of advanced techniques will likely lead to even greater insights.
Computational Approaches to Protein Folding Prediction
Computational approaches are vital for understanding and predicting protein folding. These methods offer insights that are often unattainable through experimental approaches alone. In the realm of molecular biology, accurate protein folding prediction can drastically alter the landscape of research and therapeutic development. As proteins play crucial roles in nearly all biological processes, predicting their structure based on amino acid sequences is of paramount importance.
Through computational methods, researchers can simulate folding processes, analyze protein stability, and explore dynamic behavior over time. The integration of computational techniques with experimental data enhances the overall understanding of protein conformational states and their implications in various biological activities. In practical terms, these approaches are not just theoretical; they have direct applications in drug discovery, disease modeling, and biotechnology advancements.
Molecular Dynamics Simulations
Molecular dynamics simulations are a powerful tool in the computational biology toolkit. They allow for the observation of atomic movements in proteins over time. By using classical mechanics, molecular dynamics provides a detailed view of how proteins fold and refold under various conditions. The fundamental principle involves calculating the forces on each atom within the protein to predict its movement.
This method generates trajectory data that reveals the time-dependent behavior of proteins, depicting how they transition through various conformational states. The effectiveness of these simulations relies on accurate force fields, which define how atoms interact based on their positions and distances. Advantages include the ability to visualize folding pathways, identify stable conformations, and analyze fluctuations in structure under different environmental conditions.
Homology Modeling
Homology modeling also plays an essential role in protein folding prediction. This method is based on the assumption that proteins with similar sequences will have similar structures. By utilizing known structures of homologous proteins, researchers can construct models for proteins where experimental data may be lacking.
The process begins by identifying a template structure with a significant sequence alignment. Following alignment, the model can be generated through a series of steps, such as loop modeling and side-chain placement. One notable benefit of homology modeling is its cost-efficiency compared to experimental structure determination. However, its accuracy depends heavily on the quality of the template protein.
Ab Initio Methods
Ab initio methods represent a different computational strategy for predicting protein structures. Unlike homology modeling, which relies on existing structures, ab initio methods predict folding based solely on the amino acid sequence. This approach is often complex and requires substantial computational power due to the vast conformational space explored.
Ab initio techniques involve energy minimization and molecular simulations, which seek to identify the lowest energy conformation that a protein could adopt. Among the challenges faced are the immense number of possible structures that a protein could theoretically take, making it difficult to ascertain the most stable one. However, advances in algorithms and computing capabilities are making ab initio methods increasingly viable and valuable in the protein folding prediction domain.
In summary, computational approaches like molecular dynamics simulations, homology modeling, and ab initio methods contribute significantly to the understanding of protein folding. They provide tools that not only allow for detailed analysis but also guide experimentalists in their research. As these methods continue to evolve, their implications for molecular biology, drug discovery, and disease understanding will inevitably expand.
Machine Learning and AI in Protein Folding
The intersection of machine learning, artificial intelligence, and protein folding has opened new avenues for research and application. Traditional methods for predicting protein structures have limitations, making it crucial to explore innovative techniques. AI and machine learning provide a robust framework to analyze complex data efficiently, leading to more accurate folding predictions which can significantly impact fields like drug discovery and personalized medicine.
Preamble to Machine Learning Techniques
Machine learning encompasses a variety of algorithms that enable systems to learn from data and make predictions or decisions without explicit programming. Various techniques can enhance protein folding predictions, aiding in identifying patterns and trends in biological data. Among these techniques, supervised learning, unsupervised learning, and reinforcement learning play essential roles.
- Supervised Learning: This approach utilizes labeled datasets, where the model learns from examples. For instance, when predicting the structures of proteins, the model trains on known protein structures.
- Unsupervised Learning: This technique involves grouping unlabelled data to find hidden patterns. It can be significant in identifying clusters of similar protein structures.
- Reinforcement Learning: In this model, an agent learns to make decisions by receiving rewards or penalties based on its actions. This method has potential in optimizing folding pathways.
Machine learning techniques provide a powerful toolkit for tackling the complex challenges of protein folding prediction, offering new perspectives and accelerating discoveries in the field.
Deep Learning Models

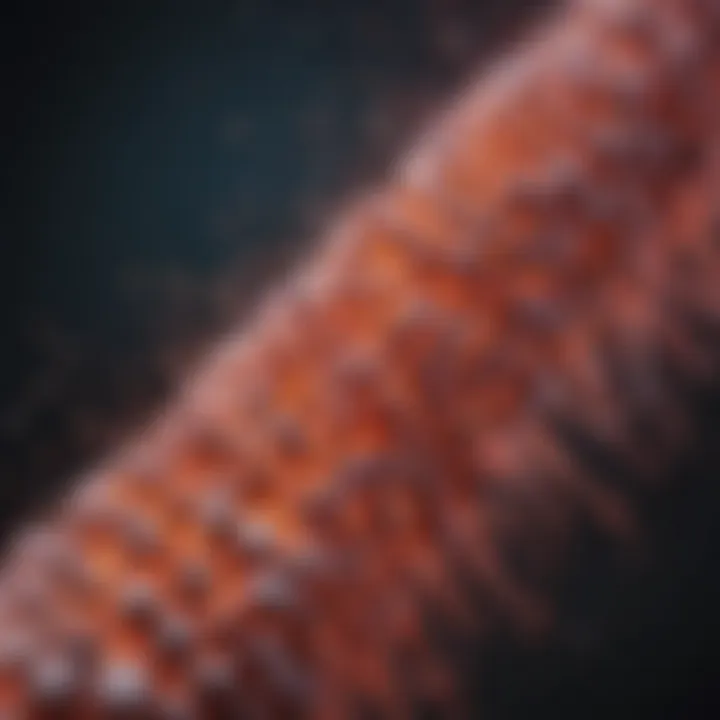
Deep learning, a subset of machine learning, employs neural networks with many layers to process data with high complexity. This approach is particularly advantageous in protein folding prediction due to its ability to learn features automatically from raw data.
Convolutional neural networks (CNNs) and recurrent neural networks (RNNs) are two prominent deep learning architectures used in this context.
- Convolutional Neural Networks: CNNs excel at processing grid-like data, such as images. In protein folding, CNNs can analyze spatial relationships between amino acids, identifying key structural features influencing folding.
- Recurrent Neural Networks: RNNs are effective for sequential data. They can capture dependencies in the sequence of amino acids, essential for understanding the folding process as proteins undergo transitions.
Implementing deep learning models has produced promising results, demonstrating significant improvements in prediction accuracy and efficiency. As research evolves, these models continue to refine their capabilities, paving the way for revolutionary advances in protein folding.
Notable Algorithms
Several notable algorithms have been instrumental in advancing protein folding predictions through machine learning and AI. Each algorithm has unique strengths suited for various aspects of the protein folding process.
- AlphaFold: Developed by DeepMind, AlphaFold utilizes deep learning to achieve unprecedented accuracy in predicting protein structures. It incorporates both supervised and unsupervised learning methods and has dramatically influenced the protein folding landscape.
- Rosetta: While initially a traditional computational modeling tool, it has integrated machine learning to enhance its predictive accuracy. Rosetta’s strength lies in modeling complex interactions at atomic resolution.
- PSIPRED: This is a secondary structure prediction method that employs neural networks to predict the arrangement of protein segments. It is valuable for providing insights that aid in understanding the folding process.
These algorithms showcase the versatility and potential of machine learning and AI in advancing protein folding prediction, underscoring the necessity of continuous research and development in this rapidly evolving field.
Noteworthy Advances in Protein Folding Prediction
The field of protein folding prediction has witnessed remarkable advances in recent years, underscoring its significance in our understanding of biological processes. These gains are not merely academic; they have tangible applications that can impact healthcare, drug design, and our grasp of molecular diseases. Evaluating the noteworthy advances in this area reveals both the complexity of challenges faced and the innovative tools employed to overcome them.
Recent Breakthroughs and Their Implications
Recent breakthroughs in protein folding prediction have primarily emerged from the interplay of artificial intelligence and computational biology. One prominent example is AlphaFold, developed by DeepMind. This deep learning model made headlines by achieving unprecedented accuracy in predicting protein structures. The implications are vast. Accurate folding predictions can drastically reduce the time and costs associated with experimental methods, such as X-ray crystallography or nuclear magnetic resonance.
Another significant advance is the development of new algorithms that utilize graph-based neural networks. These networks model proteins as graphs, where nodes represent amino acids and edges represent interactions between them. This perspective allows for a more nuanced understanding of folding pathways and stability.
Additionally, recent studies show how integrating evolutionary data improves prediction accuracy. By analyzing homologous proteins across species, researchers can identify conserved folding patterns that enhance model training. This integration of various data sources represents a formidable leap in our predictive capabilities.
"The intersection of AI and molecular biology stands to transform our understanding of life at the molecular level."
Case Studies of Successful Predictions
The practical application of these advances can be illustrated through various case studies. One notable case involves the prediction of the structure of the SARS-CoV-2 spike protein. Researchers utilized advanced models to accurately predict how this protein folds, which was crucial for vaccine development efforts. The successful prediction aided scientists in understanding the mechanisms of viral entry into human cells and facilitated the rapid design of effective vaccines, such as the Pfizer-BioNTech and Moderna mRNA vaccines.
Another case is the determination of the structure of human prion protein, linked to neurodegenerative diseases. Through computational techniques, scientists unraveled its folded structure, providing insights into disease pathology and possible therapeutic targets.
Successful protein folding predictions have also been observed in the enzyme domain. For example, the folding model generated for a specific enzyme allowed researchers to identify mutations that could enhance enzyme activity. These examples showcase not only the technical prowess of predictive algorithms but also their potential to inform practical biological and medical applications.
These case studies highlight the dynamic interplay between recent advances in protein folding prediction and their far-reaching implications, reinforcing the need for continued research and refinement of these computational methods.
Challenges in Protein Folding Prediction
The journey of understanding protein folding prediction is filled with numerous challenges. These challenges arise from the inherent complexity of protein structures and the limitations of current predictive models. Grasping these obstacles is vital for researchers and practitioners in the field because they directly influence the advancement of methods and the accuracy of predictions.
Complexity of Protein Structures
Proteins are intricate macromolecules composed of long chains of amino acids. The unique sequence of these amino acids dictates how the protein will fold into its three-dimensional structure. This folding process is not straightforward; it involves various interactions such as hydrogen bonds, ionic interactions, and hydrophobic effects. These interactions increase the overall complexity. Additionally, proteins can exist in multiple conformations, which can complicate accurate modeling of their folded states.
To illustrate the challenges in capturing this complexity, consider that a typical protein can have thousands of atoms. Simulating every possible interaction and pathway for such a structure requires immense computational power. Even with advances in technology, limited computational capacity continues to hinder the predictive capabilities.
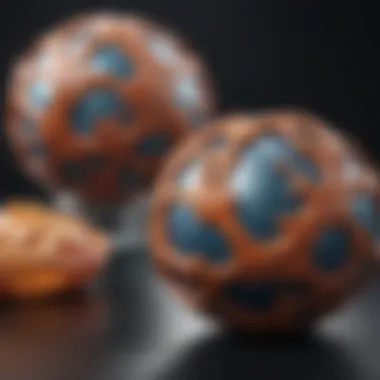
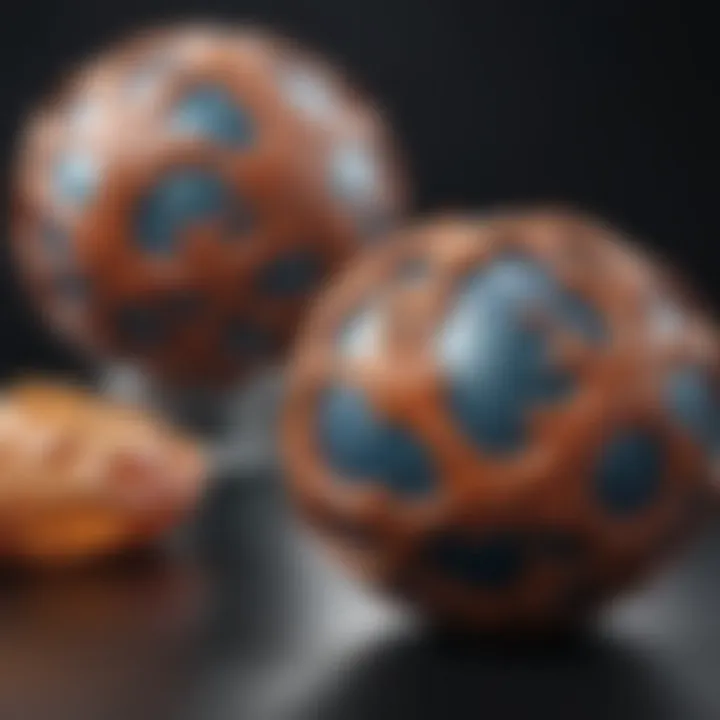
Limitations of Current Models
Current predictive models face several limitations that affect their ability to accurately forecast protein folding. Many traditional methods, such as molecular dynamics simulations, can take significant time to yield results. As a consequence, they are often incapable of simulating larger proteins within a reasonable timeframe. For instance, while molecular dynamics is essential, it may fail to account for the full range of protein dynamics.
Furthermore, algorithms based on homology modeling rely on existing data from known structures. This method assumes that similar sequences will fold similarly, yet this is not always the case. The vast diversity of protein structures makes this approach occasionally unreliable.
Another limitation is observed in ab initio methods, which attempt to predict folding based solely on physical principles, without prior knowledge of the structure. Although innovative, these methods often struggle with complexity and computational demands.
Understanding the challenges in protein folding prediction not only informs researchers about the current limits but also aids in finding innovative solutions to overcome these obstacles.
In summary, the complexity of protein structures combined with the limitations of current predictive models presents significant challenges in protein folding prediction. Addressing these issues is crucial for advancing the field and developing more reliable models for future research.
Future Directions in Protein Folding Research
The field of protein folding research is advancing rapidly, necessitating a strong focus on future directions. Understanding what these advancements entail is not merely an academic exercise; it has tangible implications for healthcare, biotechnology, and molecular biology as a whole. The interplay of innovative technologies and an interdisciplinary approach can yield significant progress in addressing the complexities inherent in protein folding prediction.
Emerging Technologies and Methods
Recent innovations in computational biology have transformed predictive models of protein folding. Technologies such as quantum computing and enhanced molecular dynamics simulations are at the forefront of these advancements. Quantum computing, for example, offers the capacity to analyze protein structures on a scale previously unattainable. By leveraging qubits, researchers can perform calculations that would take classical computers an impractical amount of time.
In addition, techniques like cryo-electron microscopy (cryo-EM) provide high-resolution structural information. This clarity is crucial for improving existing models and can be integrated with artificial intelligence tools that refine predictions based on historical data. Likewise, tools that implement alpha-fold methods showcase how deep learning can derive patterns from immense datasets.
These methods, among others, not only enhance predictive power but also increase the accuracy of forecasts about protein interactions and stability, thereby influencing drug design and disease modeling.
Integration of Interdisciplinary Approaches
As the complexity of protein folding turms evident, integrating different disciplines becomes vital. Fields such as bioinformatics, structural biology, and machine learning must converge to address existing challenges. For instance, combining insights from biophysics and computational algorithms can improve the robustness of predictions. This multidisciplinary collaboration can lead to a better understanding of folding pathways and misfolding consequences, an area of study crucial for neurodegenerative diseases.
Furthermore, collaboration between academia and industry can facilitate real-world applications. By sharing resources and data, both sectors can accelerate the development of novel therapies. Partnerships can also provide exposure to diverse methodologies and techniques, enriching the research landscape.
"The success of future protein folding research lies in the integration of various disciplines that can synergize for greater outcomes."
Emerging technologies and interdisciplinary collaboration signify a promising horizon in protein folding research. As these elements coalesce, the field stands to benefit from new insights and enhanced methodologies that hold potential for medical advancements and a deeper understanding of biological processes.
Epilogue
The conclusion of this article serves a vital role in synthesizing the previous discussions within the context of protein folding prediction. This intricate field is pivotal not only in molecular biology but also significantly impacts areas like drug discovery and the understanding of various diseases. As we near the end of this exploration, it is crucial to underscore the importance of the findings and their broader implications.
Summary of Key Points
In summary, the major aspects covered include:
- Definition and Importance: Protein folding is fundamental to understanding biological functions, as misfolding can have dire consequences on health.
- Computational Approaches: Various computational techniques such as molecular dynamics simulations, homology modeling, and ab initio methods have transformed the prediction landscape.
- Machine Learning Applications: The utilization of machine learning and artificial intelligence has gained momentum, showcasing how deep learning models enhance prediction accuracy.
- Recent Breakthroughs: Noteworthy advancements highlight the effective application of predictive models in real-world scenarios, including significant case studies.
- Challenges and Limitations: Despite advancements, challenges persist, particularly regarding the complexity of protein structures and limitations in current predictive models.
- Future Directions: Emerging technologies and interdisciplinary approaches suggest promising avenues for further research.
These points underscore the multifaceted nature of protein folding prediction and advocate for a thorough understanding of its intricacies.
Call for Continued Research
The call for continued research in protein folding prediction cannot be overstated. As challenges remain in accurately predicting protein structures, fostering an environment of collaborative research is essential. This includes refining computational models, exploring novel algorithms, and integrating insights from various fields such as biochemistry, pharmacology, and computer science.
Continued exploration will not only yield improvements in prediction accuracy but also enhance our understanding of biological processes and the underlying mechanisms of diseases. The implications of such research extend beyond theoretical frameworks, affecting practical applications in healthcare and biotechnology.
"The future of protein folding prediction necessitates a sustained scholarly effort to overcome the current limitations."
In summary, nurturing an ecosystem that supports innovative research and interdisciplinary collaboration is imperative. By addressing existing gaps and pushing the boundaries of knowledge, we can collectively advance the field into new territories, ultimately benefiting scientific inquiry and society at large.