The Integration of AI in Agriculture: Transforming Farming Practices
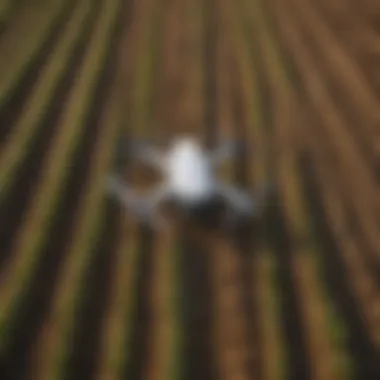
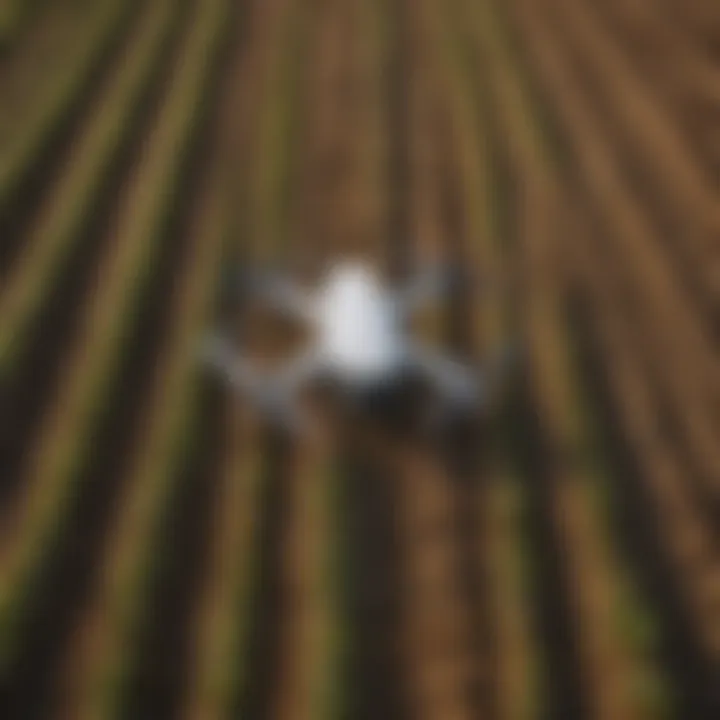
Intro
The landscape of agriculture is undergoing a significant transformation, driven by the integration of artificial intelligence (AI) technologies. As the global population expands, there is an increasing demand for efficient food production. To meet this demand, farmers and researchers are exploring innovative solutions that not only improve productivity but also enhance sustainability. AI presents a unique opportunity to optimize farming practices through advanced data analytics, machine learning, and automation.
The role of AI in agriculture is not merely about improving yields. It encompasses a broader spectrum that includes environmental stewardship and ethical considerations. This article will delve into how AI technologies are reshaping farming practices today and what implications these advancements carry for the future of agriculture. From precision farming to automated machinery, the various applications of AI will be examined, shedding light on both the benefits and the challenges involved in adopting these technologies.
Foreword to AI in Agriculture
The integration of artificial intelligence in agriculture is a transformative development, reshaping how farming practices are conducted. This shift is essential as it reflects the ongoing pursuit of sustainability, efficiency, and improved productivity. In a world facing increasing food demand and environmental concerns, the application of AI technologies offers a promising solution. Understanding this topic allows for insight into how modern agriculture can adapt and thrive amidst these challenges.
Artificial intelligence encompasses various technologies, including machine learning, robotics, and data analytics, which can be harnessed to address agricultural needs. Through its implementation, farmers can make informed decisions that optimize resource use and enhance yields. Furthermore, there is a growing recognition of the role that AI can play in addressing issues such as climate change, pest management, and soil health. Thus, exploring the integration of AI in agriculture is pertinent for anyone engaged in the field, providing valuable perspectives on modern farming practices.
Defining Artificial Intelligence in the Context of Farming
Artificial intelligence in agriculture refers to the use of advanced technologies to analyze data and automate processes. It enables farmers to achieve tasks such as monitoring crop health, predicting yields, and optimizing supply chains. AI systems analyze complex datasets, from weather patterns to soil conditions, to provide actionable insights. This is invaluable in minimizing waste, enhancing profits, and promoting sustainable practices.
Key elements of AI in agriculture include:
- Machine learning: Systems that can learn from data, identifying patterns and making predictions.
- Robotics: Automated machines that conduct tasks with high precision and speed.
- Data analytics: Techniques that process data for informed decision-making.
Understanding these components clarifies how AI is revolutionizing farming. As farmers adopt these technologies, they position themselves at the forefront of agricultural innovation.
Historical Overview of Technology in Agriculture
The history of technology in agriculture spans thousands of years, with transformative advancements shaping practices over time. Early innovations included the plow and irrigation systems which significantly enhanced productivity. Over the centuries, additional inventions, such as mechanized harvesting and fertilizers, pushed the boundaries of what was possible.
In recent decades, the advent of computers and the internet marked an important evolution. These developments allowed for advanced data collection, leading to the emergence of precision agriculture. This approach utilizes data-driven methods to monitor and manage field variability in crops. The introduction of satellite technology and aerial imagery further enhanced farmers’ abilities to assess and manage their fields more effectively.
Today, the landscape is changing again with AI technologies. These innovations enable farmers to analyze vast amounts of data quickly, providing insights previously unattainable. The progression from manual techniques to sophisticated AI tools highlights an ongoing commitment to improving agricultural efficiency and sustainability. Understanding this history contextualizes the current trends and the future of farming.
Current Applications of AI in Agriculture
The integration of AI in agriculture represents a significant advancement in modern farming practices. AI technologies are not just enhancing operational efficiency but also transforming the very landscape of agricultural possibilities. This section delves into the current applications of AI, emphasizing their critical roles and contributions to farming today.
Precision Agriculture Technologies
Precision agriculture is a key application of AI that aims to optimize field-level management regarding crop farming. Utilizing technologies like GPS, sensors, and IoT, farmers can gather substantial amounts of data about their fields. Machine learning algorithms analyze this data to help in making informed decisions.
- Benefits include tailored solutions for specific sections of a field, improving yield through variable rate technology.
- Farmers can apply resources—like seeds, water, and fertilizers—more efficiently.
- This approach not only increases productivity but also minimizes waste and reduces costs.
Research has shown that fields managed with precision agriculture can yield significantly higher returns compared to traditional farming methods. An important aspect to consider is that while the upfront investment may be high, the long-term savings and increased outputs justify this cost.
AI-Driven Crop Monitoring Tools
The ability to monitor crops accurately is paramount for aspiring high yields and quality produce. AI-driven crop monitoring tools, using drones and satellite imagery, allow farmers to keep a close eye on crop health and development. These tools analyze images and data to detect issues early, such as pest infestations and irrigation problems.
- Remote sensing technologies can identify unhealthy plants, prompting quicker responses before larger infestations occur.
- Regular monitoring allows for improved crop management and resource allocation.
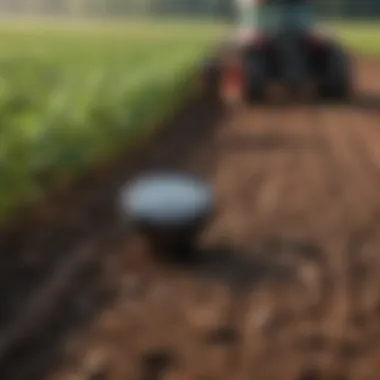
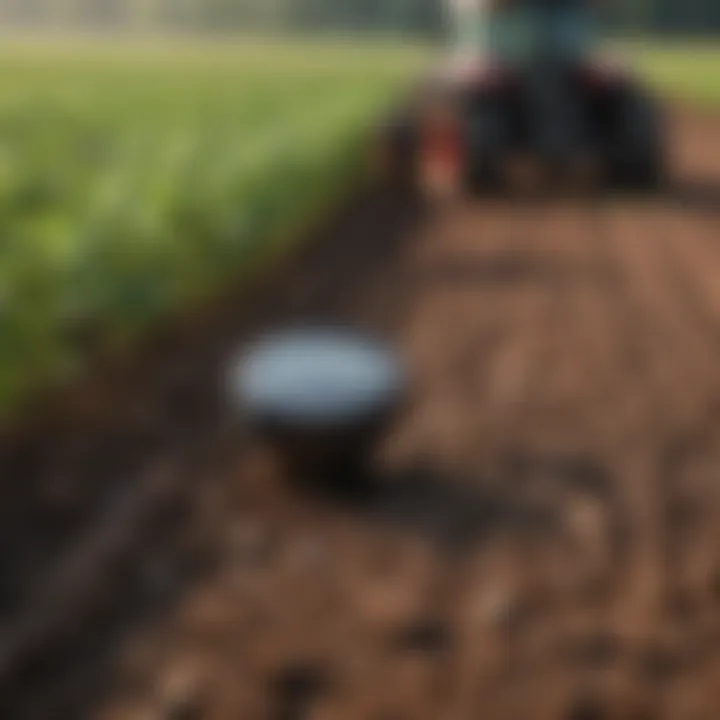
These advancements help minimize losses and optimize production cycles. Predictive analytics applied in monitoring tools guide farmers on when to plant or harvest, thus making operations more efficient and time-sensitive.
Automated Machinery and Robotics
Automation in agriculture refers to the use of AI-powered machinery to perform various farming tasks. This includes planting, weeding, and harvesting. Automated systems replace traditional labor with machines that can work consistently and efficiently.
- Examples of these machines include autonomous tractors and robotic harvesters designed to operate with minimal human intervention.
- Using robotic systems not only reduces labor costs but also addresses labor shortages common in many agricultural regions.
Automation paves the way for a more efficient and less labor-intensive agricultural process. As technology evolves, these machines grow smarter, learning from data and improving their operations over time.
"The use of AI in agriculture does not eliminate the need for farmers but empowers them to make more informed decisions and manage their resources better."
Benefits of AI Integration in Farming
Understanding the benefits of AI integration in farming is critical for grasping how these technologies facilitate modern agriculture. AI applications are not merely enhancements; they are transformative forces that can redefine how we approach farming. The importance of applying artificial intelligence in agriculture transcends individual benefits. It encompasses more effective decision-making, increased productivity, and improved sustainability.
AI-powered tools analyze vast data sets, enabling farmers to make informed choices that can greatly enhance their operations. Given the rising demand for food globally, applying AI becomes essential in maximizing crop yields while maintaining sustainable practices. Below are several specific benefits derived from AI integration in farming.
Enhancing Crop Yields and Efficiency
AI technologies are pivotal in improving crop yields and operational efficiency. By employing various algorithms and machine learning models, farmers can forecast weather patterns, soil conditions, and other crucial production factors with greater accuracy.
Data from sensors and drones allows farmers to monitor crops in real time. For instance, platforms like Climate FieldView gather field data and provide insights that help optimize planting schedules and harvest timing. Farmers using this technology see measurable increases in yield.
Moreover, precision agriculture, which relies on AI, allows for tailored input application. Instead of applying fertilizers or water uniformly, targeted methods can be employed. This leads to higher productivity and reduces unnecessary expenditure in resources.
Reducing Waste and Resource Use
AI plays a significant role in minimizing waste and optimizing resource utilization. Using AI-driven irrigation systems can lead to water savings by analyzing soil moisture and weather forecasts. By applying water only when necessary, these systems reduce wastage significantly.
For instance, smart irrigation systems from companies like RainMachine utilize real-time data to adjust water application by predicting moisture needs. Additionally, AI tools can optimize the use of fertilizers, ensuring they are applied in the right amounts and at the right times. This not only minimizes the cost of resources but also limits environmental impact, promoting sustainability.
Improving Pest and Disease Management
Another crucial benefit of AI in farming is its ability to improve pest and disease management. AI technologies such as image recognition and data analysis can identify pests or disease outbreaks before they become widespread.
For example, Plantix is an app utilizing machine learning to detect plant diseases through images taken by farmers. This allows for swift action, which is critical for limiting crop damage. By analyzing historical data alongside real-time observations, AI systems can predict pest migration patterns, enabling farmers to implement proactive management strategies.
These measures lead to healthier crops, ultimately resulting in higher yields and lower input costs. As research continues to explore the synergy between AI and crop protection strategies, it becomes increasingly clear that the integration of these technologies in agriculture is a game changer.
Challenges in Implementing AI in Agriculture
The integration of artificial intelligence into agriculture offers promising advances but does not come without its challenges. Understanding these challenges is crucial for both the successful implementation of AI technologies and the overall enhancement of agricultural practices. It is essential to acknowledge these barriers to develop effective strategies that allow farms to adapt and thrive in an increasingly digital landscape.
Technical Barriers and Limitations
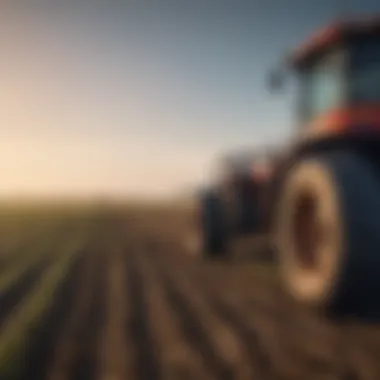
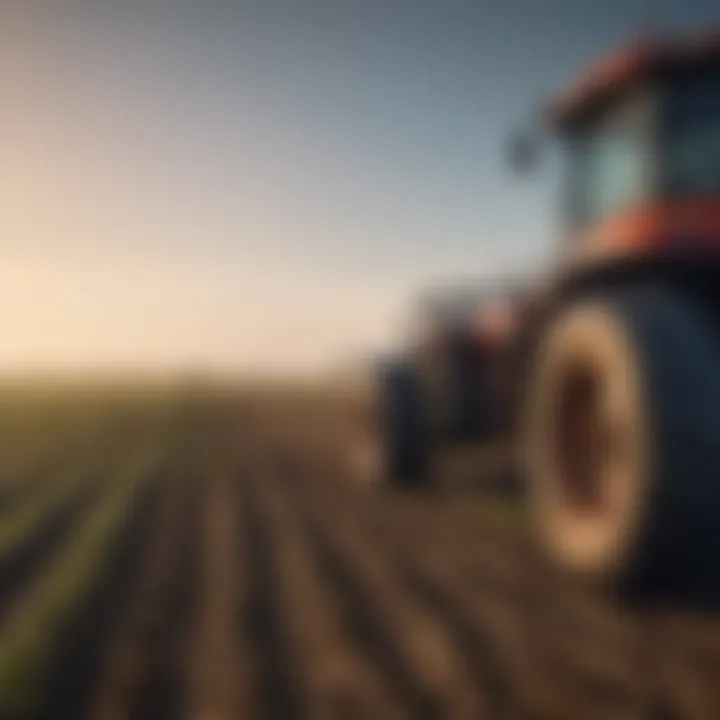
AI technologies require a solid infrastructure to work effectively. This can include specialized hardware, software systems, and skilled personnel. Many farmers lack the necessary access to high-speed internet or advanced computational devices, limiting their ability to utilize AI fully. For instance, precision agriculture systems that rely on data collection can be hindered by poor connectivity in rural areas.
Moreover, there is a need for robust data analytics capabilities. Farmers might possess data regarding soil health or crop yield, but without proper tools, this information remains underutilized. In addition, the complexity of integrating new technologies with existing farming equipment is often a concern. As a result, the steep learning curve and initial setup costs can deter farmers from embracing AI solutions.
Economic Constraints for Farmers
Economic realities play a significant role in the adoption of AI technologies in agriculture. Smallholder farmers, in particular, may find it difficult to invest in advanced AI systems. The initial costs of purchasing software and hardware can be a significant obstacle, especially when profit margins are tight. In some cases, farmers may rely on traditional methods that are less costly, even if they lead to lower productivity.
In addition, the variable return on investment associated with AI technologies creates uncertainty for farmers. While AI can lead to increased efficiency eventually, the risk of initial low yields during transition periods can discourage investment. Farmers often prioritize immediate monetary needs over long-term technological investments. This leads to a hesitance to allocate budgets toward advancements that may or may not yield immediate results.
Data Privacy and Security Concerns
As AI systems become more integrated into agricultural practices, concerns about data privacy and security begin to surface. Farmers are increasingly collecting sensitive data about their operations, including crop management techniques, soil conditions, and potentially proprietary information about their farming strategies. This data, if mismanaged or accessed unlawfully, can result in serious breaches of privacy.
Additionally, the reliance on cloud-based solutions for data storage raises questions about security. Cyberattacks targeting agricultural data can disrupt operations and lead to significant financial damages. Understanding how to protect sensitive information while benefiting from data-driven insights becomes essential.
In summary, while AI in agriculture presents opportunities for improvement and efficiency, challenges exist at multiple levels that must be addressed. Identifying these barriers—technical limitations, economic constraints, and data security concerns—will pave the way for a clearer path forward in the integration of AI technologies.
The Future of AI in Agriculture
The future of artificial intelligence in agriculture represents a substantial shift in how farming will be conducted. As we reflect on past developments, it is evident that AI has the potential to enhance productivity, sustainability, and decision-making processes in farming. Understanding the implications of these technologies is critical in shaping a future that prioritizes food security and ethical practices.
Emerging Technologies and Innovations
AI systems are constantly evolving, bringing forth new technologies that influence agriculture. Machine learning algorithms, drones, and sensor technology are becoming integrated into daily farming operations. Drones, for instance, can now survey crop health and provide real-time data on conditions that affect growth. This information allows farmers to make precise decisions about water, fertilizer, and pesticide application.
Moreover, the Internet of Things (IoT) is playing a pivotal role in agriculture. IoT devices enable the monitoring of crops and livestock through connected sensors, which can communicate critical data instantly. This immediacy helps in understanding plant health and in optimizing resources more efficiently.
Potential Impact on Food Security
Food security is a foremost concern globally, and AI holds immense promise in addressing this issue. With a growing global population, the need for increased and stable food production is paramount. Advanced AI technologies can significantly enhance crop yields through better planning and resource management.
By leveraging predictive analytics, AI can forecast potential harvests and market demands. This forecasting can minimize food waste by aligning production with actual consumption trends. Furthermore, AI aids in identifying the best crop varieties and farming practices suitable for specific environments, ensuring that agricultural outputs can keep pace with consumer needs.
Ethical Considerations in AI Deployment
As agriculture becomes increasingly reliant on AI technologies, ethical considerations must be prioritized. Issues such as data privacy, algorithmic bias, and equitable access to technological resources are pertinent. Farmers must be educated on how to protect their data and ensure it is used responsibly.
Concerns also arise regarding the impact of AI on employment. Automation may displace traditional farming jobs, leading to socioeconomic challenges in rural areas. Thus, the integration of AI in agriculture should be managed with policies that ensure fair labor practices and support for those affected.
Case Studies: Successful AI Implementation in Farming
Examining successful case studies of AI implementation in farming is essential for understanding the real-world impact of these technologies. These case studies provide concrete evidence of how AI applications improve efficiency and productivity. They highlight the benefits of specific practices, such as precision agriculture and robotics, and offer insights into the broader implications of AI in agriculture. By analyzing these cases, stakeholders can glean lessons that are applicable to their own operations.
Examples from Precision Agriculture
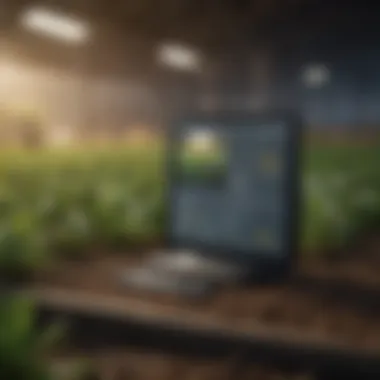
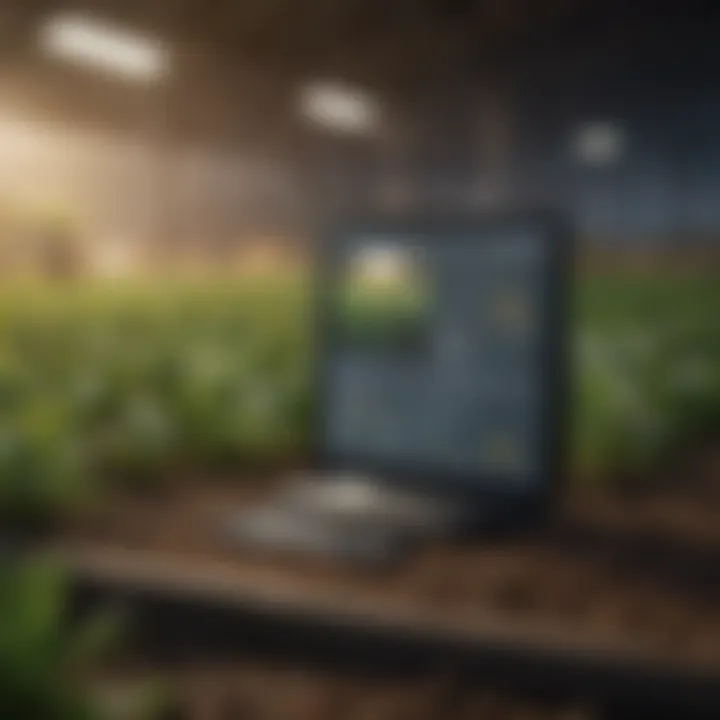
One notable example of AI in precision agriculture is the use of** climate-smart agriculture technologies**. These tools utilize AI algorithms to analyze vast amounts of data regarding weather patterns, soil conditions, and crop health. For instance, a farm in California adopted AI-driven solutions from a company called Savana, integrating satellite imagery and machine learning to monitor crop growth. As a result, the farm improved its yield by 20% while reducing water usage by 30%. This illustrates how AI can contribute to more sustainable practices by enabling farmers to make informed decisions based on real-time data.
Moreover, John Deere, a leader in agricultural machinery, has developed technologies that include AI to provide insights into field conditions. Their machines are equipped with sensors that gather information, which AI processes to optimize planting schedules and fertilizer applications. With this, farmers can maximize their productivity while minimizing waste during the growing season.
Lessons Learned from Robotics Use
The implementation of robotics in agriculture is another area where valuable lessons arise. Harvest CROO Robotics created robots designed to pick strawberries, a task typically done by hand. By using advanced AI systems, the robots can assess the ripeness of strawberries and pick them with precision. This innovation addresses labor shortages in the agricultural sector while maintaining high-quality produce.
However, this implementation teaches important lessons about integration and workforce concerns. While robotics can increase efficiency, it is crucial to address the potential displacement of human labor. Educating workers about new technologies and retraining them for new roles in a technology-driven landscape is key to ensuring a smooth transition.
"AI not only changes what we grow but also how we grow it. It brings efficiency, but we must manage the associated challenges with foresight."
The successes noted in these case studies affirm the transformative power of AI in agriculture. As more farms adopt similar technologies, the sector can witness a shift towards sustainable and efficient farming practices, enabling a balance between productivity and environmental stewardship.
Policy and Regulatory Frameworks for AI in Agriculture
The integration of AI in agriculture brings about significant advantages, but it also necessitates a careful examination of policy and regulatory frameworks. Understanding these policies is crucial to harnessing AI's full potential while safeguarding the interests of farmers, consumers, and the environment. A robust policy framework can ensure sustainable development and foster innovation by outlining clear rules and guidelines that govern the use of AI technologies.
Current Regulations Affecting AI Technologies
Currently, regulations affecting AI technologies in agriculture vary across regions. In many cases, these regulations focus on data protection, privacy, and safety standards. AI systems often require vast amounts of data for effective functioning. Thus, regulatory frameworks must ensure that data is collected, stored, and utilized ethically. For instance, GDPR in the European Union sets strict guidelines on personal data usage, impacting how agricultural AI companies operate. Moreover, existing agricultural regulations sometimes need to adapt to include AI considerations, particularly concerning biosecurity and the use of drones.
Additionally, regulations must address liability when AI systems fail or cause harm. This diverts responsibility between technology providers and agricultural producers. Proper clarity in regulations regarding AI tools is essential to mitigate risks, promote trust, and encourage wider adoption of such technologies in the agricultural sector.
Future Policy Directions
Looking ahead, policy directions must evolve alongside technological advancements. Policymakers should focus on flexibility in regulations to accommodate the rapid development of AI technologies. Collaborative approaches among industry stakeholders, including farmers, tech companies, and regulatory bodies, can yield comprehensive frameworks that address challenges while encouraging innovation.
Potential future policies may include:
- Incentives for Sustainable Practices: Governments could provide financial incentives for adoption of AI technologies that enhance sustainability.
- Standardization of Data Usage: Creating a unified data governance framework for AI applications can facilitate easier integration across different systems.
- Research Funding: Supporting research initiatives that explore the implications of AI in agriculture can inform better policy frameworks.
"A well-thought-out regulatory framework is essential for the successful integration of AI technologies in agriculture, balancing innovation with safety and ethical considerations."
Through continued assessment and proactive policy-making, the agricultural sector can effectively navigate the complexities introduced by AI technologies. In doing so, it can not only improve operational efficiency but also ensure long-term resilience against emerging challenges such as climate change.
The End and Recommendations
The integration of artificial intelligence in agriculture has profound implications for the farming industry. This article has highlighted how AI technologies can enhance productivity, optimize resource use, and lead to more sustainable practices. The final insights provide a synthesis of the critical elements discussed, emphasizing the importance of continued exploration in this field.
Summary of Key Insights
From precision agriculture technologies that improve yield predictions to automated machinery that reduces labor costs, AI presents a myriad of opportunities. Key insights include:
- Increased Efficiency: AI tools help in making informed decisions leading to higher yields and lower waste. Farmers can collect and analyze data to optimize their operations.
- Sustainability Focus: Technologies like AI-driven sensors and drones promote sustainable practices by ensuring better resource management and precision application of inputs.
- Economic Implications: While there are economic barriers, the long-term benefits of AI in agriculture can outweigh initial investments, making it viable for many farmers.
- Challenges and Considerations: The successful adoption of AI is accompanied by challenges such as data security and privacy, which need careful consideration.
"Adopting AI technologies in agriculture can not only enhance productivity but also ensure sustainable farming practices for the future."
Future Research Directions
Looking forward, several areas merit attention. Researchers and practitioners should consider exploring:
- Advanced Data Analytics: Investigating new methods in data collection and analysis can lead to better insights into crop health and yield predictions.
- Integration of AI with Traditional Practices: Understanding how AI can complement traditional farming methods will be vital for widespread acceptance and application.
- Policy Development: There is a need for research into regulatory frameworks that support the ethical use of AI in farming. This includes privacy laws surrounding data collected by AI technologies.
- Economic Models: Developing models that assess the economic impact of AI adoption will help farmers make informed decisions on investments.
Through these research directions, stakeholders can better navigate the landscape of AI in agriculture, ensuring the technology contributes positively to the industry. Continued engagement and innovation in this area will be crucial in shaping the future of farming.