Exploring Plant Biology through Artificial Intelligence
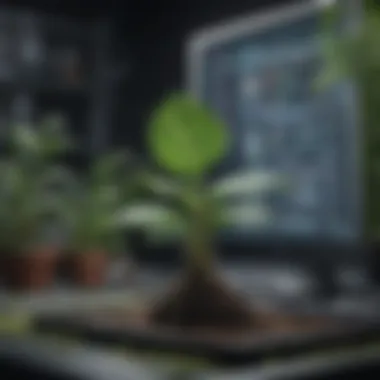
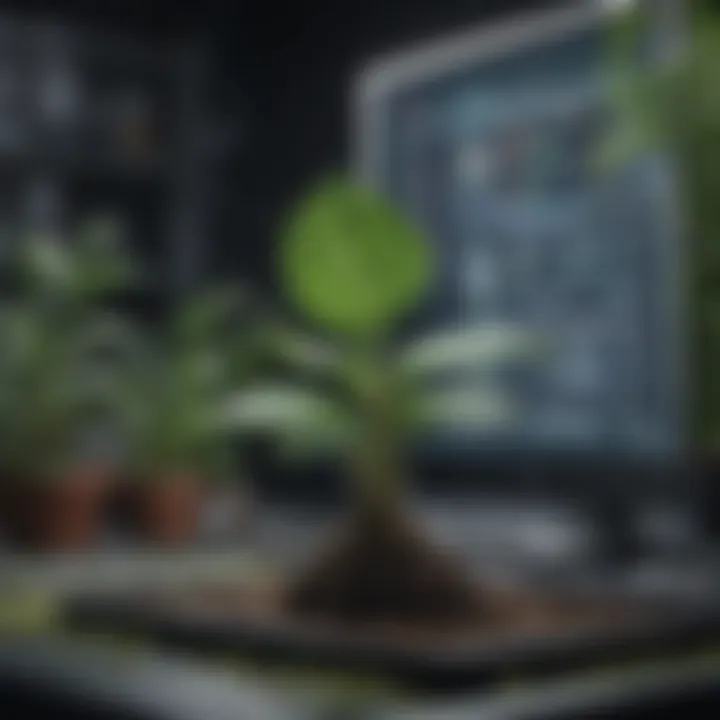
Intro
The integration of artificial intelligence with plant biology provides profound opportunities for both sectors. There is a promising collaboration forming that reshapes agricultural practices and enhances our understanding of plant systems. As we delve deeper into the technological advancements presented by AI, it becomes increasingly important to discuss how these advancements impact plant sciences. This exploration reveals key interactions between the two fields that can lead to sustainable practices and improved crop yields.
Understanding the underlying connections is crucial not only for researchers but also for educators and professionals who are interested in applying these technologies. This initial exploration aims to set the groundwork for understanding the complexities involved in merging plant biology and artificial intelligence.
Research Overview
Research into the amalgamation of plant biology and AI signifies a progressive shift toward addressing food security and environmental challenges. This section presents a comprehensive look at the methodological approaches used in recent research and the significance of these findings.
Methodological Approaches
Various methodologies are being employed to explore the intersection of these fields. Techniques include:
- Data Analytics: The use of big data analytics permits in-depth examination of plant health, growth patterns, and genetics.
- Machine Learning: By employing machine learning algorithms, researchers can predict plant responses to environmental changes.
- Remote Sensing: Drones and satellites equipped with advanced sensors allow for monitoring of plant health across vast agricultural lands.
These methodologies provide valuable insights into improving the efficiency of agricultural practices. They pave the way for a more data-driven understanding of botanic processes.
Significance and Implications
The implications of AI in plant biology are significant. AI not only aids in refining agricultural practices but also promotes sustainability. Some notable implications include:
- Enhanced crop management through predictive analytics.
- Reduced resource consumption, thus lowering the ecological footprint of farming.
- Better understanding of plant behavior, which can lead to innovations in crop breeding.
These advancements contribute to sustainable agricultural systems, which are vital in the face of global challenges like climate change and population growth.
Current Trends in Science
Keeping abreast of the latest trends is crucial in understanding the evolving nature of plant biology and AI. This section outlines innovative techniques and interdisciplinary connections fueling the two fields.
Innovative Techniques and Tools
Recent progress includes:
- Robotic Harvesting: Robots are being developed to identify and harvest ripe crops autonomously, streamlining food production.
- AI-driven Breeding Programs: Analyzing genetic data informs breeding efforts, aiming for disease-resistant plants.
- Blockchain for Transparency: Using blockchain technology enhances the traceability of food products while involving AI in predicting market demands.
These techniques exemplify the innovative spirit of current research and its focus on using technology for better outcomes.
Interdisciplinary Connections
The intersection of plant biology and AI inevitably involves collaboration across various fields. Connections can be found in:
- Bioinformatics: Merging biological data with computational tools enhances research capabilities.
- Environmental Science: AI aids in analyzing ecological data, supporting sustainable practices.
- Agronomy: Integrative techniques improve soil and crop management strategies.
These interdisciplinary connections foster a rich environment for research and development that benefits not only plant sciences but also broader environmental goals.
"The future of agriculture lies at the intersection of technology and nature, where artificial intelligence acts as a bridge to sustainability."
Prolusion to Plant AI
The integration of artificial intelligence in plant biology is of significant relevance today. With the increasing challenges faced by the agricultural sector, such as climate change, pest infestations, and the demand for higher yields, the need for innovative solutions is pressing. Plant AI represents a convergence of technology and botanical science. This field leverages machine learning, data analysis, and other AI technologies to optimize agricultural practices and enhance our understanding of plant behavior.
By employing advanced algorithms, researchers aim to unlock insights from vast datasets, which can lead to improved crop management strategies. Additionally, Plant AI has the potential to foster sustainable methods in agriculture. As this interdisciplinary field continues to grow, it brings forth not only solutions but also a range of considerations, including ethical implications and the integration of technology within existing agricultural systems.
Definition of Plant AI
Plant AI refers to the application of artificial intelligence techniques in the study and management of plants. This includes using machine learning, computer vision, and data analytics to understand plant behavior, optimize growth conditions, and predict agricultural trends. By employing AI, researchers can analyze complex variables that affect plant health and productivity. Hybridizing plant biology with AI technologies provides a platform for enhancing traditional practices, driving innovations in farming techniques.
Historical Context
The relationship between technology and agriculture is not new. Historically, agricultural practices have evolved through innovations such as the plow or irrigation systems. However, the advent of digital technologies marks a significant turning point. In recent decades, concepts like precision farming have emerged, driven by the need for efficiency and sustainability. The introduction of AI into this realm is a natural progression. Early implementations included basic data analysis, which has since transformed into sophisticated machine learning models. These advancements allow for real-time monitoring and adaptive management of crops. As a result, understanding the historical timeline of this integration aids in appreciating its future trajectory.
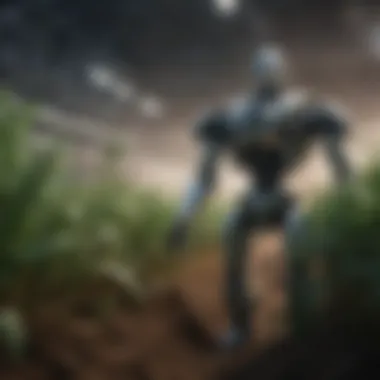
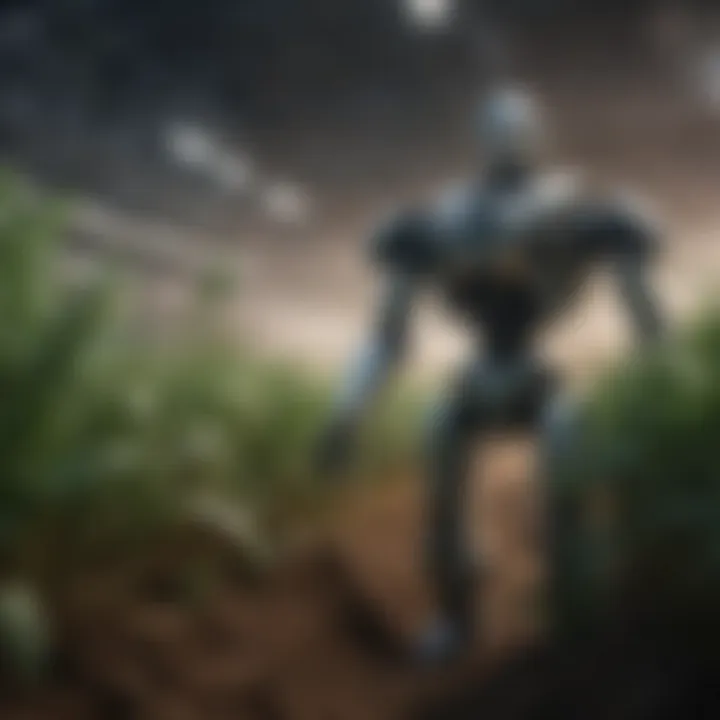
Fundamentals of Plant Biology
Understanding the foundational aspects of plant biology is crucial in the context of integrating artificial intelligence into agricultural practices. Plant biology encompasses various processes that drive plant growth, communication, and adaptation. By exploring these fundamentals, we can appreciate how AI can enhance these natural processes, ultimately improving agricultural productivity and sustainability. This comprehension not only signifies the scientific interactions at play but also underscores the potential technological advancements that can arise from this intersection.
Photosynthesis and Plant Growth
Photosynthesis is the process by which plants convert sunlight into energy. This occurs mainly in the chloroplasts, where chlorophyll absorbs light energy and uses it to transform carbon dioxide and water into glucose and oxygen. Understanding photosynthesis is essential as it is the primary energy source for plant growth and development.
Factors influencing photosynthesis include light intensity, carbon dioxide concentration, and temperature. Studies have shown that optimizing these factors can improve crop yield. Artificial intelligence can play a pivotal role in monitoring these conditions. For instance, AI algorithms can analyze data from sensors to manage light exposure, thereby maximizing the photosynthesis rate.
"Efficient management of photosynthesis can lead to sustainable agricultural practices and ensure food security in the face of climate change."
Plant Communication
Plants communicate with one another and their environment in less obvious ways compared to animals. They use chemical signals, like volatile organic compounds, to attract pollinators or warn neighboring plants of threats, such as pests. This biological communication facilitates cooperative strategies that enhance survival and growth.
Artificial intelligence can aid in understanding these complex signaling mechanisms. Machine learning algorithms can analyze patterns in plant behavior and communication, providing insights into how plants interact with each other and their environment. By harnessing this data, we can develop better crop management practices, simplify pest control, and enhance plant health.
Genetic Variation and Adaptation
Genetic variation is the raw material for evolution in plant species. It contributes to the adaptability of plants in changing environments. Understanding how plants adapt to specific climates or soil conditions can help optimize their growth in various environments. Genetic diversity ensures that some plants can withstand diseases or shifts in climate while others cannot.
AI can assist in analyzing large genomic datasets. Through advanced data analysis, researchers can identify beneficial traits in plants for breeding programs. Breeding strategies informed by AI could rapidly accelerate improvements in crop resilience, especially in the face of global challenges such as climate change and food security.
Artificial Intelligence Technologies
The domain of artificial intelligence technologies plays a pivotal role in integrating the fields of plant biology and artificial intelligence. It provides various tools and methodologies that enhance our ability to analyze and interpret complex biological data, streamline agricultural practices, and improve overall productivity. In this context, AI serves not just as a collection of algorithms, but as a fundamental component that can reshape how we understand and interact with plant systems.
By utilizing AI technologies, researchers and practitioners can make informed decisions based on data-driven insights. This leads to significant benefits such as increased efficiency, reduced costs, and enhanced sustainability in agricultural practices. It is important to acknowledge that the integration of these technologies requires careful consideration regarding data management, system compatibility, and the potential ethical implications surrounding their use.
Machine Learning in Agriculture
Machine learning emerges as a cornerstone of AI technologies in agriculture. This approach leverages algorithms that can learn from and make predictions based on data. The application of machine learning can be seen in various areas, including:
- Predictive modeling for crop yields
- Automated pest detection and management
- Soil quality assessment and recommendation
With the help of machine learning, farmers can identify efficient practices and mitigate risks more effectively. The ability to analyze large datasets enables them to adapt to changing conditions, leading to improved resilience in agricultural practices. However, the effectiveness of machine learning is contingent on the quality of data used. Poor data can lead to misguided conclusions and ineffective strategies.
Data Analysis and Plant Phenotyping
Data analysis is crucial for understanding plant phenotyping, which involves measuring and analyzing physical and biochemical traits. Advanced statistical methods, powered by AI, facilitate the extraction of meaningful insights from vast datasets. Scientists can evaluate traits such as growth rates, disease resistance, and stress responses. Some key elements include:
- High-throughput phenotyping systems
- Real-time data collection techniques
- Integration of environmental data for comprehensive analysis
These systems enable researchers to identify which plant traits are most beneficial for specific environments and how to cultivate them effectively. As a result, the development of new plant varieties can be expedited, ultimately enhancing food security and environmental sustainability.
Computer Vision Applications
Computer vision technologies are making significant strides in plant research and agriculture. They allow for the analysis of visual data from plants using high-resolution images and video. Key applications include:
- Disease Detection: Early identification of plant diseases through image recognition algorithms.
- Growth Monitoring: Tracking plant growth patterns over time to assess health and development.
- Yield Estimation: Estimating potential crop yields based on visual assessments of plant health.
The constant advancement in computer vision capabilities enables precise monitoring of crops. Farmers can act swiftly upon detection of issues, potentially saving entire harvests. However, successful implementation demands not only sophisticated technology but also proper training of personnel handling these systems.
With the right tools and insights, artificial intelligence can revolutionize our approach to agriculture, emphasizing the importance of data-driven methodologies.
Applications of Plant AI
The integration of artificial intelligence within agricultural practices is transforming various segments of plant biology. These applications provide numerous benefits, ranging from optimizing plant growth to enabling efficient resource management. By utilizing machine learning, data analysis, and predictive modeling, plant AI creates smart solutions for contemporary agricultural challenges. This section covers three key applications: precision agriculture, disease prediction and management, and crop yield optimization.
Precision Agriculture
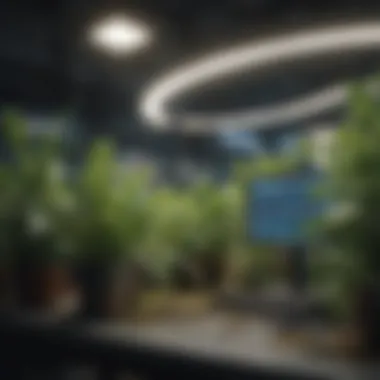
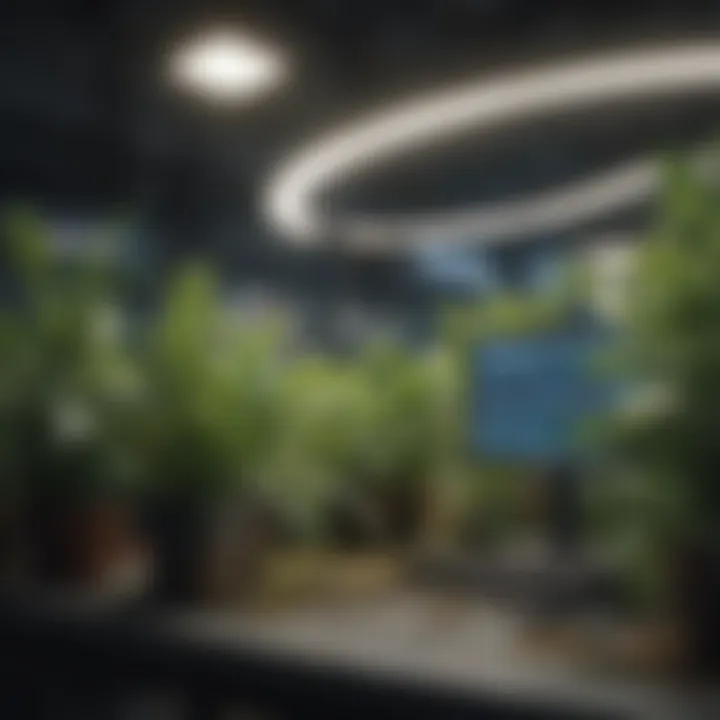
Precision agriculture represents a paradigm shift in farming. By harnessing AI, farmers can monitor their crops through real-time data collection. Technologies such as satellite imagery and drones collect precise information on soil health, plant health, and weather conditions. These data points are analyzed by AI models that help farmers make informed decisions on irrigation, fertilization, and pest control.
The implementation of precision agriculture leads to significant cost reductions, resource conservation, and enhanced productivity. For example, irrigation can be adjusted based on real-time soil moisture data, preventing overuse of water and improving the overall health of crops.
"Using AI in precision agriculture is not just about technology; it’s about rethinking how we grow food without compromising on quality or quantity."
Disease Prediction and Management
Identifying plant diseases at an early stage is critical for maintaining crop health. AI applications in disease prediction involve analyzing vast datasets, which can include historical data, environmental conditions, and plant health indicators. Machine learning algorithms can detect patterns and predict outbreaks before they occur.
Farmers can leverage these insights to apply targeted treatments, significantly reducing the need for widespread pesticide applications. For instance, AI can improve disease resistance by analyzing genetic information from plants, thus aiding in the development of more resilient crop varieties. This targeted approach minimizesenvironmental impact while safeguarding yields.
Crop Yield Optimization
Optimizing crop yields is paramount for meeting the food demands of a growing population. AI offers advanced analytical capabilities which can forecast yields based on various parameters including weather patterns, soil conditions, and pest pressures. By using data collected from sensors and drones, farmers can adjust their strategies to enhance yield.
Both historical and real-time data contribute to creating models that predict crop performance. Such models enable farmers to implement timely interventions that can result in improved outputs. Crop rotation strategies, soil management techniques, and fertilizer schedules can all be fine-tuned through gleaned insights, ensuring a sustainable and efficient agricultural practice.
In summary, the applications of plant AI pave the way for an innovative agricultural future. These technologies offer precision, predictability, and efficiency, ultimately leading to improved farming practices and enhanced sustainability.
Challenges in Implementing Plant AI
Understanding the challenges in implementing Plant AI is crucial for its effective deployment in agriculture. As the integration of artificial intelligence technologies into plant biology progresses, several obstacles come into play. These issues can affect data handling, system compatibility, and operational scalability. Addressing these challenges can lead to more sustainable agricultural practices, optimizing resource use and improving crop yields.
Data Privacy and Security
Data privacy and security present significant hurdles in the adoption of Plant AI. As agritech companies collect vast amounts of data from farms, consumers and farmers alike worry about how this information is stored and utilized. Sensitive data, including location, crop types, and farm management practices, must be adequately protected.
The potential for data breaches or misuse necessitates strong security measures. Regulations, such as the General Data Protection Regulation (GDPR) in Europe, impose strict requirements on data handling and user consent. Agritech companies must invest in robust cybersecurity frameworks to ensure compliance and build trust with users. Failure to protect data could lead to not only legal implications but also reputational damage.
Integration of AI into Existing Systems
Implementing Plant AI requires seamless integration with existing agricultural systems. Many farms utilize traditional methods and technologies that may not easily accommodate modern AI solutions. This integration can be complicated by varied hardware, software, and data formats.
To develop an effective Plant AI system, agricultural stakeholders must ensure compatibility across these diverse systems. This may involve reengineering current processes and investing in new technologies. Efforts to integrate AI successfully can lead to more efficient workflows and provide farmers with actionable insights. However, the transition pathway can be daunting for many, posing a significant challenge.
Scalability Issues
Scalability is another critical consideration when deploying Plant AI solutions. As different farms have unique configurations, environments, and crop types, creating adaptable AI systems requires flexible frameworks. A solution that is viable for one farm may not be suitable for another due to differences in scale or operational needs.
Scalability also encompasses the ability to process large datasets as AI tools become more common. Data handling must grow in tandem with the increasing amount of information generated by farms. Challenges can arise in maintaining efficient processing capabilities, especially when integrating machine learning algorithms. Ensuring scalability involves not only technology and infrastructure adjustments but also ongoing support and training for users.
"The success of AI in agriculture hinges on solving integration and scalability challenges. Without addressing these elements, the full potential of Plant AI may remain unrealized.”
By tackling these challenges head-on, the agricultural sector can harness the power of Plant AI. This would not only lead to better crop management but also encourage more sustainable practices, ensuring that the benefits of innovative technology are so much greater.
Ethical Considerations
Ethical considerations play a critical role in the integration of artificial intelligence into plant biology. As technology advances, the implications for agricultural practices, environmental sustainability, and societal values become increasingly complex. It is essential to analyze various facets of these ethical challenges to ensure that the benefits of Plant AI are maximized while minimizing potential harms.
Impact on Employment in Agriculture
The advent of AI technologies in agriculture raises questions about the future of employment within this sector. While automation and AI systems can enhance efficiency and productivity, there exists a palpable concern regarding job displacement. Many traditional farming roles may become obsolete as machines and algorithms take over tasks like planting, harvesting, and monitoring crop health.
However, this does not mean that overall employment will decline. Instead, there will likely be a shift in the type of jobs available. New opportunities could arise in technological support, data analysis, and system management. Farmers who adapt to these new technologies may take on roles that require a combination of agricultural knowledge and technical skills, fostering a workforce that is more versatile than ever.
Environmental Consequences
The interplay between AI and environmental sustainability introduces multiple ethical dilemmas. On one hand, AI applications in agriculture can optimize resource use, leading to decreased chemical input and reduced waste. For example, precision agriculture leverages AI to assess soil health and predict irrigation needs, greatly reducing water usage.
On the other hand, increased reliance on technology can lead to unintended consequences. Over-automation may lead to detrimental practices that harm local ecosystems. AI systems can potentially promote monoculture farming, thus reducing biodiversity, which is critical for maintaining resilient agricultural systems.
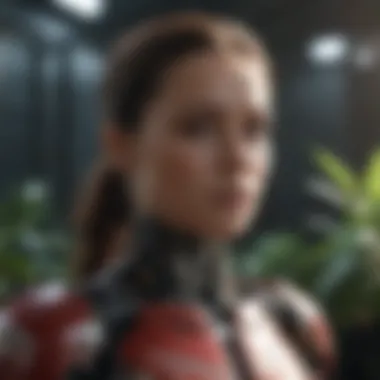
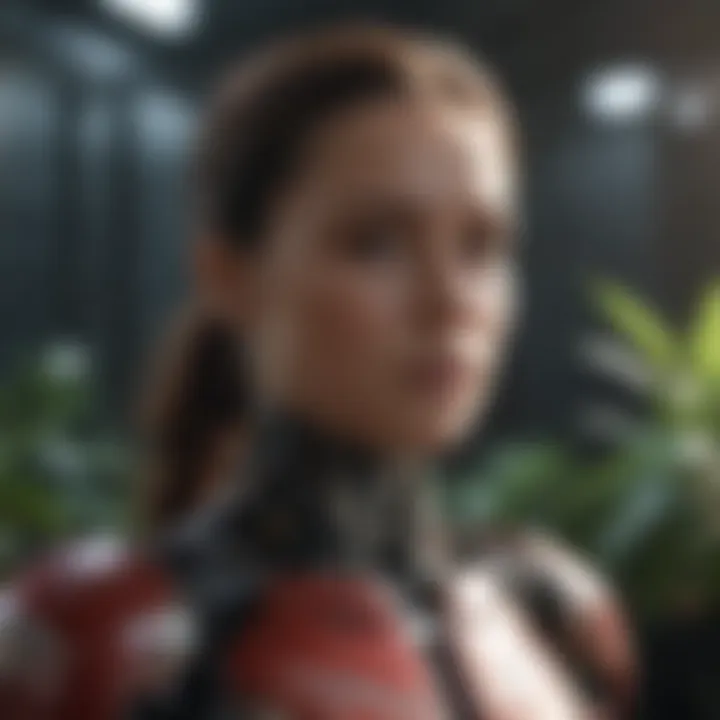
"The ethical landscape of AI in agriculture must be navigated carefully. Balancing efficiency with environmental responsibility is paramount."
Access to Technology
Equitable access to AI technology is another significant ethical consideration. The digital divide remains a pressing issue, particularly in developing regions. Farmers with limited access to cutting-edge technologies may struggle to compete with larger, more tech-savvy agricultural enterprises. This lack of access not only limits their productivity but may also exacerbate socioeconomic inequalities within the agricultural sector.
There is a growing need to address how technology is disseminated and if equitable models can be developed to support small farmers. Initiatives that promote open-source technology and community-based resources can help bridge this gap. By ensuring that all farmers have an opportunity to utilize AI advancements, the industry can move towards a more inclusive and just agricultural future.
Future Directions in Plant AI Research
The future directions in Plant AI research represent a critical juncture for both the fields of botany and technology. As agricultural demands increase globally, integrating AI's capabilities with plant biology can lead to enhanced research outcomes and practical applications. Exploring these advancements can yield significant benefits, such as improved crop resilience, efficient resource management, and a deeper understanding of environmental interactions.
Innovative Technologies on the Horizon
Recent years have seen a surge in innovative technologies that promise to revolutionize how we approach Plant AI. One such development is the use of drones equipped with AI-driven imaging systems. These drones can monitor large agricultural fields and collect detailed data on plant health and soil conditions. This data can be analyzed in real-time, allowing farmers to make informed decisions quickly.
Another promising technology is the application of AI algorithms in predicting plant behavior. For example, models that analyze historical climate data alongside crop performance can help in anticipating yield outcomes. By leveraging such predictive models, farmers can adjust their practices preemptively, potentially mitigating losses.
Interdisciplinary Collaborations
The potential of Plant AI lies significantly within interdisciplinary collaborations. Engaging professionals from diverse backgrounds—such as computer science, environmental science, and agronomy—can lead to richer insights and innovative solutions. For instance, data scientists can partner with plant biologists to develop more sophisticated machine learning algorithms that encompass a broader range of variables, enhancing data accuracy and applicability.
Furthermore, universities and research institutions can foster these collaborations by promoting cross-disciplinary research projects. Such initiatives not only can enhance academic inquiry but also can pave the way for technological advancements that benefit agricultural practices.
Potential for Sustainable Practices
Sustainability remains a pivotal concern in modern agriculture. The intersection of Plant AI and sustainable practices can facilitate significant progress in this area. By employing AI tools, farmers can optimize resource usage, such as water and fertilizer, thereby reducing environmental footprints. Precision agriculture techniques allow for targeted application of inputs, minimizing waste.
Additionally, AI can assist in promoting biodiversity in agricultural settings. By analyzing ecosystems holistically, AI-driven models can help in designing crop rotations and agricultural methods that support ecological balance, leading to more sustainable farming practices in the long term.
In summary, the future of Plant AI is bright with potential. By embracing innovative technologies, fostering interdisciplinary collaboration, and prioritizing sustainability in agricultural practices, the field can advance significantly, ensuring that the benefits of AI extend beyond theoretical applications to create tangible outcomes in food security and environmental protection.
Integrating AI with Traditional Agricultural Methods
The integration of Artificial Intelligence (AI) with traditional agricultural methods is not merely a trend. It emerges as a necessary evolution in addressing global agricultural challenges such as sustainability, productivity, and food security. By combining the insights of AI with the time-tested practices of traditional farming, we can enhance agricultural output while respecting the environment. This section explores specific elements, benefits, and considerations involved in this integration.
Blending Knowledge Systems
Blending AI with traditional knowledge systems offers a unique opportunity to tap into decades or even centuries of agricultural wisdom. Many farmers possess deep understandings of their local ecosystems, passed down through generations. Integrating this qualitative knowledge with AI's quantitative capabilities can yield a more holistic approach to farming.
For instance, farmers can utilize AI models to analyze soil health, climate patterns, and crop performance while grounding these analyses in local historical context. The results could lead to more informed decisions about crop rotation, pest management, and resource allocation. This symbiosis not only empowers farmers with enhanced tools but also respects and preserves valuable indigenous knowledge.
Enhancing Farmer Decision-Making
AI can significantly enhance decision-making for farmers. With the advent of data-driven insights, farmers can optimize various aspects of their operations. Tools like predictive analytics can determine the best times for planting or harvesting based on weather patterns and soil conditions.
Consider the application of Machine Learning algorithms on historical agricultural data. AI can identify trends in crop yield, thus allowing farmers to make timely adjustments to their farming strategies. The increased accuracy in predictions can reduce failures and improve economic outcomes.
Moreover, the deployment of mobile applications powered by AI can provide real-time advice to farmers. With immediate access to data, farmers can respond promptly to changes in weather or pest activities, thereby minimizing potential losses.
Combining traditional agricultural practices with AI not only improves efficiency but also secures the future of farming against unforeseen challenges.
Culmination
The conclusion of this article is essential as it encapsulates the key findings and insights drawn from the exploration of Plant AI. The intersection of plant biology and artificial intelligence represents a promising frontier that has the potential to revolutionize agriculture and enhance our comprehension of plant behavior.
Summary of Key Points
- Definition and Context of Plant AI: Plant AI combines the principles of artificial intelligence with plant biology to analyze, interpret, and optimize plant-related processes. Understanding the historical context gives us appreciation on how this field has developed.
- Fundamentals of Plant Biology: Knowledge of photosynthesis, plant communication, and genetic adaptation is crucial to frame the application of AI technologies in agriculture.
- Technological Applications: Machine learning, data analysis, and computer vision are tools that enable precision agriculture, disease prediction, and crop yield optimization, leading to more efficient farming practices.
- Challenges and Ethical Considerations: Data privacy, system integration, and scalability pose significant barriers that must be addressed. Additionally, the impact on agricultural employment and access to technology raises important ethical concerns.
- Future Directions: There are exciting innovations on the horizon, emphasizing interdisciplinary collaborations that could promote sustainable agricultural practices.
Final Thoughts on Plant AI
The integration of AI in plant biology signifies not only a technological evolution but also a paradigm shift in how we understand and interact with our agricultural systems. As we navigate this evolving landscape, the careful balance between leveraging AI for productivity and preserving ethical standards remains vital. The future of Plant AI will depend on addressing challenges while ensuring accessibility to technology for all stakeholders in the agricultural domain. Ultimately, ongoing research, ethical awareness, and collaboration will shape the future of agriculture, leading to a more sustainable and efficient food production system.
"The fusion of plant biology and artificial intelligence promises to redefine agricultural practices and enhance our understanding of the living world, offering solutions that are crucial for our collective future."
Engaging with these themes provides a roadmap for researchers, educators, and practitioners alike, illuminating the paths toward innovative solutions in the realm of plant science.