Post Translational Modification Prediction Methodologies
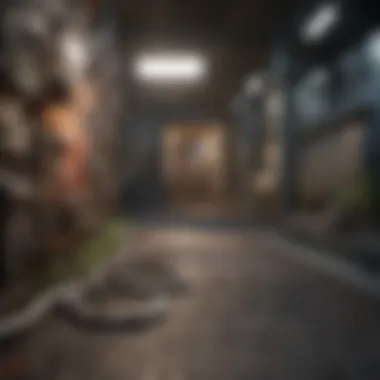
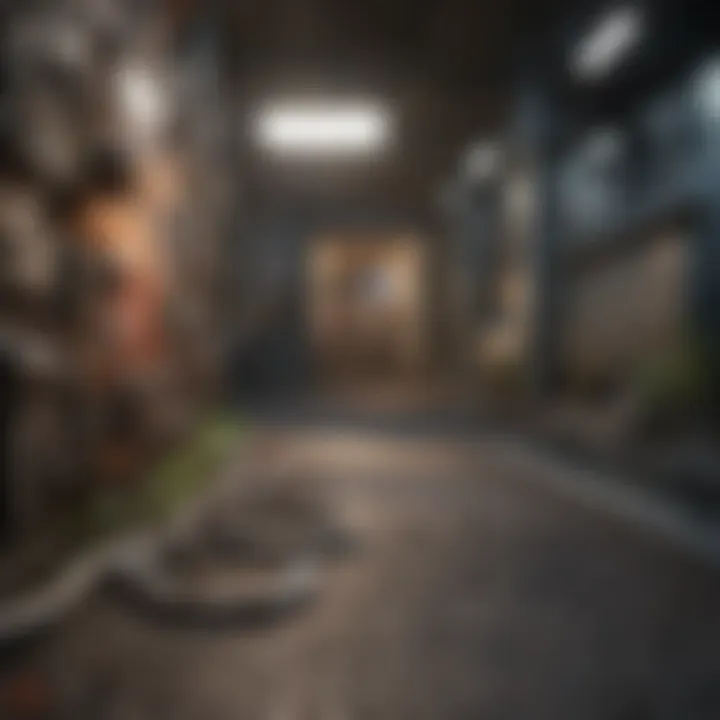
Intro
Post-translational modifications (PTMs) are critical biochemical processes that occur after a protein's synthesis. These modifications can significantly alter a protein’s function, stability, and localization. Understanding PTMs is essential for elucidating cellular mechanisms and developing therapeutic strategies. This article will explore various methodologies employed in PTM prediction and their implications in biological research and drug development.
Research Overview
Methodological Approaches
The prediction of PTMs involves a multitude of methodologies that can broadly be classified into computational and experimental techniques.
Computational Techniques
Computational methods leverage large datasets and algorithms to predict PTMs from genetic sequences. Common approaches include:
- Machine learning techniques, which employ statistical learning to identify patterns associated with PTMs.
- Sequence alignment methods, which compare protein sequences against known modification sites.
- Structural bioinformatics, where three-dimensional protein structures are analyzed to infer potential PTM sites.
These tools often utilize databases such as UniProt for validation and cross-referencing, enhancing their predictive capabilities.
Experimental Approaches
Experimental methods, while often time-consuming and costly, provide invaluable validation for computational predictions. Techniques such as mass spectrometry are integral in identifying and quantifying PTMs in vivo.
Significance and Implications
The significance of accurately predicting PTMs cannot be overstated. These modifications play a fundamental role in modulating various cellular processes, including signal transduction, cellular differentiation, and many other regulatory functions. An in-depth understanding of PTMs allows researchers to:
- Develop targeted therapies by recognizing how specific modifications impact protein function.
- Enhance drug development processes by identifying PTM-related biomarkers.
Accurate prediction methods are critical for these applications, offering a robust foundation for future scientific inquiry.
Current Trends in Science
Innovative Techniques and Tools
Recent advancements in technology have led to the emergence of innovative techniques for PTM prediction.
- Bioinformatics tools like PhosphoSitePlus and ModificationAtlas are now standard resources in the field, providing comprehensive data on known PTMs.
- Advances in deep learning have improved accuracy in predicting PTMs, enabling more robust analysis of complex datasets.
Interdisciplinary Connections
The study of PTMs is inherently interdisciplinary. Collaborations between biologists, chemists, and computer scientists foster novel approaches to PTM prediction. This convergence of disciplines enhances understanding and drives innovation in drug design and therapeutic applications.
"The integration of interdisciplinary methods is central to advancing PTM research, linking data analytics with biological applications."
The future of PTM prediction is poised for continued growth, driven by technological innovations and interdisciplinary collaboration.
Prolusion to Post Translational Modifications
The significance of post-translational modifications (PTMs) inhabits a central role in the realm of biological sciences. Understanding PTMs is crucial because they dictate a wide array of cellular functions. This understanding plays a pivotal role in research and clinical applications. Knowing how proteins can be modified after translation allows scientists to examine the complex mechanisms that regulate protein activity, stability, and interactions in cells.
PTMs represent a dynamic layer of regulation that sometimes determines whether proteins will function correctly or malfunction, leading to diseases. This complexity reveals the need for methodological approaches to predict PTMs accurately. Advanced prediction models are increasingly essential, particularly in drug discovery and disease research. As such, the exploration of PTM prediction methodologies is vital to further comprehend their implications. Understanding these can also unravel fundamental aspects of molecular biology, advancing both the academic and practical landscape.
Definition of Post Translational Modifications
Post-translational modifications refer to the chemical changes that proteins undergo after translation by ribosomes. These modifications are crucial because they can dramatically impact a protein's function and behavior within a biological system. PTMs can include the addition of specific groups to the protein structure, which can alter its activity, interaction with other molecules, and even its location within cells.
Types of Post Translational Modifications
There are several types of PTMs, each with unique characteristics and functionalities. Understanding these types helps in making sense of their diverse roles in cellular mechanisms.
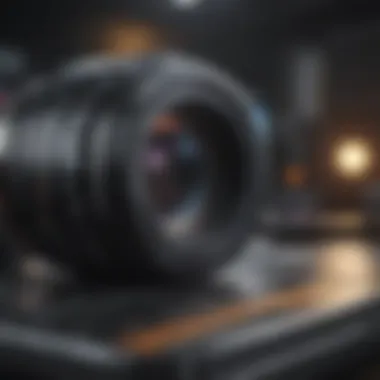
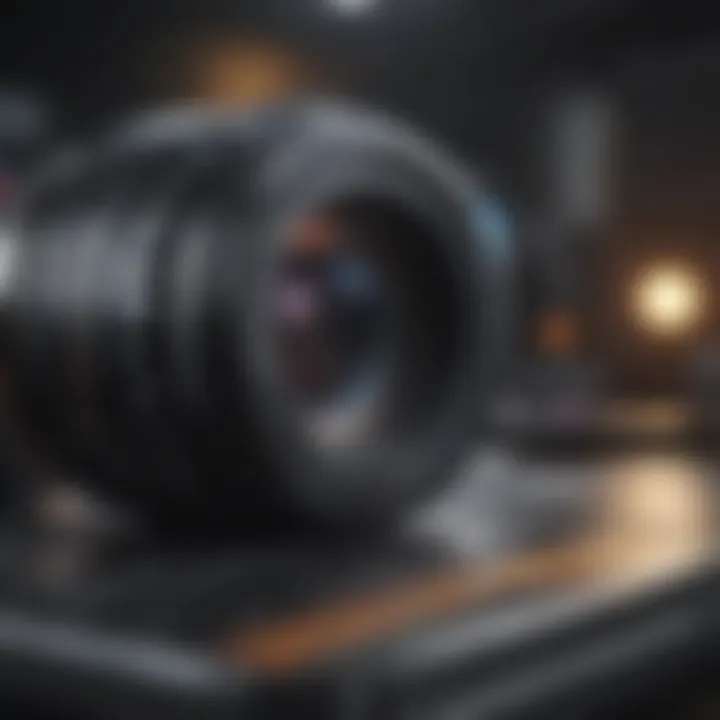
Phosphorylation
Phosphorylation is a common PTM, involving the addition of a phosphate group to an amino acid residue, typically serine, threonine, or tyrosine. It is crucial for the modulation of protein function, often acting as a switch that can turn on or off enzymatic activity. This type of modification plays a vital role in signal transduction pathways and cell regulation. Phosphorylation's significant advantage is its reversibility, allowing for rapid cellular responses to environmental cues.
Glycosylation
Glycosylation is the process of adding sugar moieties to proteins. This modification is essential for stability, signaling, and recognition processes. Glycosylated proteins often exhibit increased half-lives in circulation and can improve cellular interactions. The unique feature of glycosylation is its role in cell communication and immune responses. However, the complexity of glycan structures can pose challenges in precise prediction and analysis.
Ubiquitination
Ubiquitination involves the attachment of ubiquitin, a small protein, to a target protein. This modification typically tags proteins for degradation, thus regulating their levels within the cell. Ubiquitination's importance lies within its roles in cell cycle control, DNA repair, and response to stress. However, the intricacies of ubiquitin signaling pathways can complicate the prediction of these modifications due to context-dependent effects.
Methylation
Methylation refers to the addition of methyl groups to specific amino acids. This modification often impacts gene expression regulation and protein interactions. Methylation can influence histone proteins and thus modify chromatin structure, which impacts gene accessibility. A key characteristic of methylation is its potential to serve as an epigenetic mechanism. However, the study of methylation is often challenged by the multitude of residues that can be modified.
Acetylation
Acetylation is the addition of acetyl groups, typically to lysine residues. This modification influences protein stability, activity, and interactions. Acetylation can also alter the charge of proteins, affecting their interaction with other molecules and cellular compartments. It is especially notable for its role in transcription regulation. Yet, like other PTMs, its predictive modeling can face difficulties due to the complexity of cellular environments.
Sumoylation
Sumoylation is a less understood but significant PTM involving the conjugation of small ubiquitin-like modifiers. This modification can impact nuclear transport, transcription, and protein stability. Its distinctive role in regulating diverse cellular functions makes it essential in research. One challenge is its relatively lower prevalence compared to other modifications, sometimes hindering comprehensive predictions.
Significance of PTMs in Biological Systems
Understanding the roles of PTMs in biological systems is essential for grasping cellular functionality. PTMs are intrinsically linked to numerous biological processes, such as cell signaling, immune responses, and development. Their complexity requires robust prediction methodologies, as inaccuracies can lead to misunderstandings about protein function and subsequent implications in disease contexts. The ongoing research into PTMs carries significant potential in molecular biology, biotechnology, and therapeutic development.
The Process of Post Translational Modification
The process of post-translational modification (PTM) is critical within cellular biology. Understanding how proteins undergo modifications after they are synthesized is essential for decoding how proteins function in various biological pathways. PTMs can alter protein structure, activity, stability, and interaction with other cellular components. Therefore, grasping the intricacies of this process is integral for predicting outcomes in biological systems.
Enzymatic Mechanisms of PTM
Enzymatic mechanisms are fundamental to the generation of post-translational modifications. Enzymes like kinases, glycosyltransferases, methyltransferases, and ligases act on specific substrates to introduce or remove molecular groups. For example, kinases add phosphate groups in a process called phosphorylation, while glycosyltransferases attach carbohydrate moieties to proteins, resulting in glycosylation.
These enzymatic reactions are highly specific. They depend on the protein structure, local environment, and availability of the substrate. Importantly, the enzymes can be regulated by various factors, such as cellular signals or changes in the environment, which adds a layer of complexity to PTM processes. Understanding these mechanisms provides insights into how modifications influence cellular functions and disease states.
Factors Influencing PTM Events
Several factors influence the frequency and type of post-translational modifications that occur.
- Cellular Environment: The biochemical environment within a cell can dictate PTM processes. Conditions such as pH levels, ion concentrations, and the presence of other molecules can either promote or inhibit specific modifications.
- Cellular Signals: Signaling pathways affect PTM events significantly. For instance, hormonal signals can activate kinase pathways, resulting in increased phosphorylation of target proteins. This dynamic regulation is essential for responding to physiological changes.
- Protein Structure: The protein's three-dimensional structure plays a vital role. Certain regions of a protein might be more accessible to enzymes and hence more likely to undergo PTM. Moreover, any conformational changes induced by interactions with other proteins may expose new sites for modification.
- Genetic Regulation: Gene expression levels determine enzyme availability and activity. Variations in transcription can lead to differences in enzyme concentration within cells, subsequently impacting PTM efficiency.
Understanding these factors improves our understanding of the mechanics behind PTM and aids in developing predictive models, which are crucial in various applications like drug development and biomarker discovery.
Challenges in PTM Prediction
Post-translational modifications (PTMs) significantly influence protein functionality and their interaction within biological systems. However, predicting these modifications presents a myriad of challenges. Recognizing and addressing these challenges is crucial for advancing the field of PTM research, facilitating better understanding of biological processes and aiding in drug discovery.
Complexity of PTM Sites
PTM sites within proteins vary greatly in their sequence and structural characteristics. The complexity arises from the unique nature of each site, influenced by factors like amino acid composition, potential interaction sites, and local structural features. Each modification can occur at multiple locations on a single protein, resulting in a vast array of possible modifications. For example, phosphorylation can happen at serine, threonine, or tyrosine residues, amplifying the prediction difficulty. Moreover, certain modifications can exhibit crosstalk, where one modification influences the likelihood or effect of another occurring. The intricate relationships between these modifications necessitate sophisticated models that can accurately predict and interpret these biological nuances.
Data Availability and Quality
The predictions of PTMs heavily rely on the availability of high-quality experimental data. This data is often scant and unevenly distributed across different types of modifications and organisms. Some modifications, such as glycosylation, are more extensively studied than others like sumoylation, resulting in data bias. Additionally, databases such as UniProt and PhosphoSitePlus provide essential information but may suffer from incomplete or outdated entries. The challenge lies not only in accessing sufficient data but also in evaluating its accuracy. Erroneous annotations can lead to misleading predictions, compounding the difficulties faced in identifying true PTM events.
Computational Limitations
Computational approaches form the backbone of PTM prediction methodologies. However, these methods face inherent limitations. Traditional algorithms may struggle with the complexity of protein structures and the subtlety of molecular interactions involved in PTM processes. For instance, many machine learning models require substantial training datasets, which may not be available for every type of PTM or organism. Additionally, factors such as overfitting and underfitting impact the models' performance, often leading to unreliable predictions. Newer techniques, such as deep learning, have shown promise but come with their own set of challenges, including the need for vast computational resources and the intricacies of model interpretability. Ultimately, overcoming these computational limitations is essential for enhancing prediction accuracy and broadening our understanding of PTM biology.
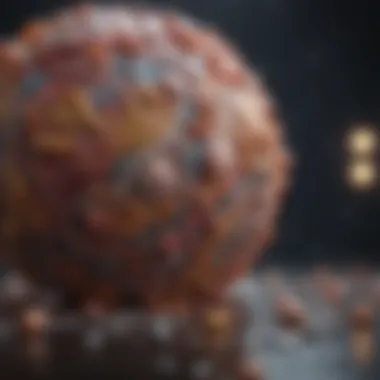
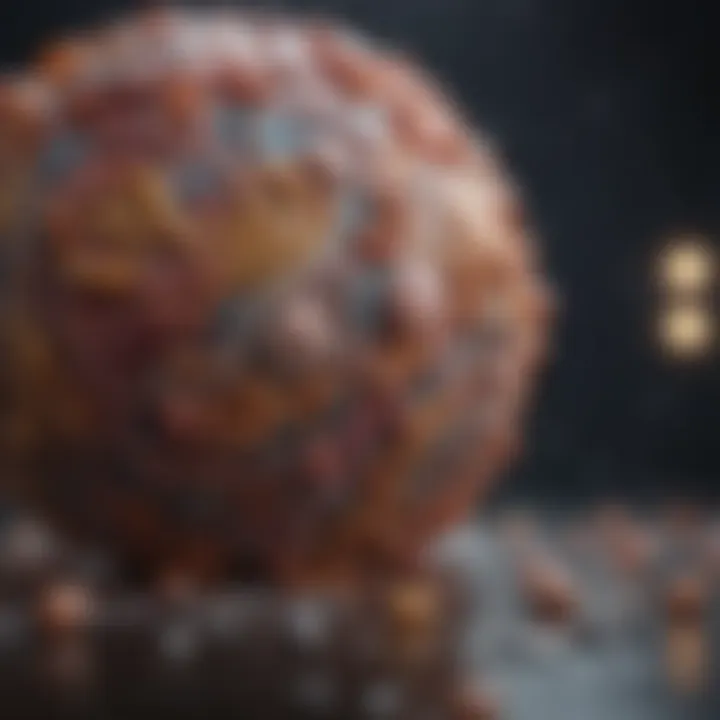
"Understanding the challenges in PTM prediction is the first step towards better methodologies and improved outcomes in biological research and therapeutic applications."
In summary, the challenges in PTM prediction stem from the inherent complexity of modification sites, the variability and quality of available data, and the limitations of current computational models. Tackling these challenges will require a concerted effort from researchers to innovate methodologies and leverage interdisciplinary approaches.
Predictive Models for Post Translational Modifications
Predictive models for post-translational modifications (PTMs) are critical in the advancement of our knowledge about the dynamics of protein functions and interactions. By utilizing these models, researchers can gain insights that can significantly impact various fields such as molecular biology, pharmacology, and biotechnology. As the sheer variety of PTMs and their effects become clearer, the need for reliable prediction methods becomes paramount. These methods not only enhance our understanding of biological processes but also inform therapeutic development and diagnostics.
Machine Learning Approaches
Machine learning offers robust methodologies for PTM prediction. These algorithms learn patterns from existing data, enabling them to make predictions on new and unseen sequences. Various machine learning techniques, such as support vector machines or random forests, have demonstrated success in identifying potential PTM sites based on sequence features.
A few advantages of machine learning in this area include:
- Increased accuracy in prediction.
- Capability to analyze large datasets efficiently.
- Flexibility to incorporate diverse data types, such as structural and sequence information.
Despite these strengths, challenges remain. The quality of the training data directly affects the model’s performance. Moreover, the biologically relevant features may often be complex and not easily codified.
Deep Learning in PTM Prediction
Deep learning represents a significant enhancement over traditional machine learning methods. With the ability to process vast amounts of data, deep learning models can uncover more complex patterns relevant to PTMs. These models rely on neural networks that automatically learn feature representations from raw data, facilitating deeper insight into the underlying sequences and structures.
Notable benefits include:
- Improved predictive capability for intricate PTM sites.
- Enhanced performance when dealing with high-dimensional data.
- Ability to integrate various types of information, including sequence, structural, and contextual cues.
However, deep learning approaches require substantial computational resources and carefully curated datasets. Researchers must navigate challenges related to overfitting and ensure the generalizability of the models.
Combined Predictive Strategies
Combining different predictive strategies enhances the accuracy and reliability of PTM prediction. Integrating machine learning with deep learning leverages the strengths of both methods. This strategy can entail using machine learning models to filter data and highlight valuable features for deep learning networks.
Advantages of combined approaches are:
- Comprehensive model performance that capitalizes on multiple data dimensions.
- Enhanced ability to interpret results, as different models contribute diverse perspectives.
- Improved efficiency in identifying functional PTMs through multi-faceted analysis.
Collaborative predictive modeling approaches that merge insights from experimental data, computational predictions, and biological knowledge can result in more reliable outcomes. By continually refining these methodologies, the field can address the complexities associated with PTMs more effectively.
Validation of Post Translational Modification Predictions
The validation of post-translational modification (PTM) predictions is a critical step in ensuring the accuracy and reliability of computational methods. Validating these predictions allows researchers to accurately link PTMs to their biological ramifications, establishing a clearer understanding of protein function and regulation. This section covers the various methodologies used for validation and their significance in the broader context of PTM research.
When considering validation, it is essential to evaluate both experimental and computational techniques. This dual approach ensures robust results and meaningful conclusions. Experimental validation provides empirical support for predictive models, while database comparisons help to contextualize findings within existing literature. The importance of these activities cannot be understated, as they provide confidence in the applicability of PTM prediction models in real-world scenarios.
Experimental Approaches to Validate Predictions
Mass Spectrometry
Mass spectrometry is a widely utilized analytical tool in the validation of post-translational modification predictions. Its capability to accurately identify and quantify proteins and their modifications makes it indispensable in proteomics. The key characteristic of mass spectrometry lies in its precision and sensitivity, allowing for the detection of low-abundance proteins and minor modifications. Researchers often regard it as a beneficial choice in this field due to its established methodology and reproducibility.
A unique feature of mass spectrometry is its ability to differentiate between various structural variants of proteins. However, it does have some disadvantages. The requirement for complex sample preparation and the need for sophisticated equipment can limit its accessibility for some laboratories. Nonetheless, its advantages often outweigh these limitations, making it a cornerstone in PTM validation.
Western Blotting
Western blotting serves as another experimental approach to validate PTM predictions. It is crucial for verifying the presence and abundance of specific proteins and modified forms thereof. The key characteristic of this technique is its ability to provide clear results through the immunodetection of proteins. Moreover, it is a common method in laboratories, which makes it readily accessible.
Western blotting's unique feature is its specificity, as it employs antibodies that can selectively bind to target proteins or modified versions. While it can yield definitive answers concerning the presence of PTMs, it is not as sensitive as mass spectrometry. Thus, it might miss low-abundance modifications. Despite this, it remains a valuable tool alongside other techniques.
Immunoprecipitation
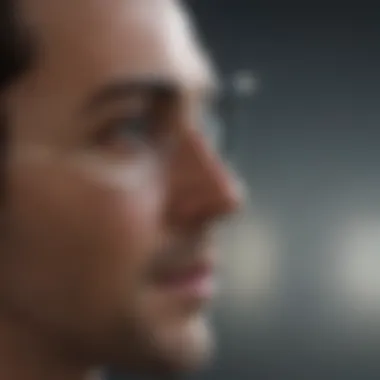
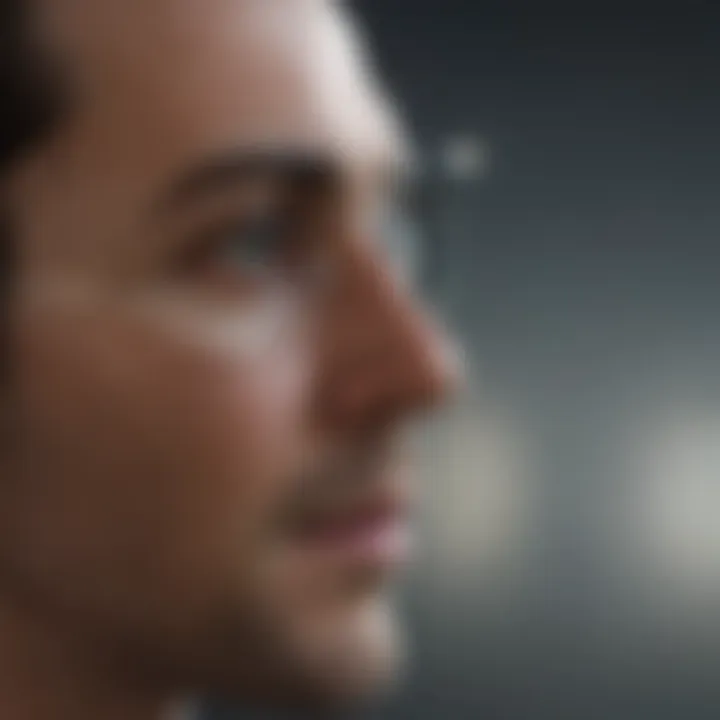
Immunoprecipitation is critical for validating PTM predictions as it allows for the specific isolation of target proteins from complex mixtures. By using antibodies to capture the protein of interest, researchers can study the associated modifications in detail. The key characteristic here is its ability to enrich for specific proteins, enhancing detection sensitivity and understanding of functional protein interactions.
A notable advantage of immunoprecipitation is its ability to work with various sample types and conditions, including cellular environments. However, like other methods, it has drawbacks, such as the dependency on high-quality antibodies, which may not always be available. Nonetheless, when carried out properly, it offers significant insights into the PTM landscape.
Comparative Analysis with Existing Databases
Comparative analysis with existing databases enables researchers to validate and contextualize their predictions. Publicly available resources like UniProt or PhosphoSitePlus create a wealth of information that can serve as a reference point. By cross-referencing predictions against these databases, researchers can identify any discrepancies and refine predictive models. Furthermore, this process highlights the ongoing evolvement in PTM research, encouraging the integration of new findings into predictive frameworks.
Applications of PTM Prediction in Research
The potential applications of post-translational modification (PTM) prediction extend far beyond basic biology. Understanding how PTMs function and their implications in various research areas is crucial for advancing our knowledge of cellular mechanisms and developing new therapies. Various disciplines benefit from these predictions, from molecular biology to pharmacology. This section focuses on three significant applications: understanding disease mechanisms, drug development and therapeutic targets, and biomarker discovery.
Understanding Disease Mechanisms
PTMs are integral to the regulation of cellular processes. Dysregulation of PTMs can lead to numerous diseases, including cancer, neurodegenerative disorders, and metabolic syndromes. By accurately predicting PTMs, researchers can gain insights into how these modifications contribute to disease pathogenesis.
For instance, the phosphorylation status of specific proteins may alter cellular signaling pathways, leading to uncontrolled cell growth in cancer. Understanding these modifications can highlight potential pathways that are disrupted in specific diseases. Once a PTM is identified, subsequent studies can focus on characterizing its effects on protein function. This line of research may guide the identification of new therapeutic strategies aimed at restoring normal function or mitigating disease effects.
Drug Development and Therapeutic Targets
In the realm of drug development, PTM predictions enable researchers to identify novel therapeutic targets. Drugs can be designed to specifically modulate PTMs, enhancing or inhibiting specific pathways. Understanding the PTM landscape of target proteins allows drug developers to focus on modifications that are crucial for their activity or stability. This targeted approach not only improves efficacy but also minimizes off-target effects, a common issue in drug development.
For example, drugs like Imatinib have been designed to target specific tyrosine kinases altered by PTMs in chronic myeloid leukemia. Continuing advancements in predictive modeling can streamline the discovery of other targeted therapies for various diseases, transforming the landscape of personalized medicine.
Biomarker Discovery
Another critical application of PTM prediction is in biomarker discovery. Biomarkers are measurable indicators of biological processes, often used in diagnostics. PTMs can serve as indicators of disease state or progression. For instance, changes in glycosylation patterns have been linked to various cancers, making them potential biomarkers for early detection or treatment response.
PTM predictions can help identify specific modifications that could serve as effective biomarkers. As research progresses, these findings may translate into clinical applications, improving diagnostic tools and personalized treatment modalities.
In summary, the applications of PTM prediction in research are vast and varied. Understanding disease mechanisms through PTM insights can enhance basic biological research. Moreover, the capability to target modifications offers significant potential for drug development. Lastly, leveraging PTMs could unveil novel biomarkers, further bridging the gap between research and clinical practice. The implications of accurate prediction echo across disciplines and open pathways to innovative solutions in healthcare.
Future Directions in PTM Research
Research focused on post-translational modification (PTM) prediction is evolving at a rapid pace. This evolution brings forth new methodologies and insights that can transform our understanding of cellular processes and disease mechanisms. As the field matures, it is essential to identify emerging technologies and interdisciplinary approaches that will define the future of PTM research. This section highlights the significant elements that will likely shape the next frontier of PTM prediction.
Emerging Technologies and Methodologies
One of the most exciting areas in PTM research is the advent of novel technologies that enhance prediction accuracy. Mass spectrometry continues to be a cornerstone for identifying PTMs, but advancements in proteomics are allowing more precise analyses. Techniques such as high-resolution mass spectrometry and ion mobility spectrometry provide detailed information about the modification states of proteins. These innovations enable researchers to dissect complex biological systems and understand how specific modifications influence protein function.
- CRISPR Technology: The application of CRISPR/Cas9 technologies for genome editing offers a new avenue for studying PTMs. By specifically targeting genes responsible for enzymes that add or remove modifications, researchers can elucidate the role of various PTMs in real-time within living organisms.
- Artificial Intelligence: Moreover, machine learning algorithms are proving invaluable in predicting PTMs from sequence data. These algorithms can analyze vast data sets to identify patterns and correlations that are not apparent through traditional methods. By incorporating biological context, they can offer predictions with a higher degree of confidence.
Interdisciplinary Approaches
The future of PTM research relies heavily on interdisciplinary collaboration. By combining expertise from diverse fields such as computer science, molecular biology, and bioinformatics, researchers can develop more holistic predictions and interpretations.
- Cross-Disciplinary Collaboration: Efforts to merge fields like structural biology and systems biology with computational predicted methods lead to more integrated insights about the cellular roles of PTMs. For instance, understanding protein structure can significantly influence predictive modeling, enhancing the quality of predictions made by computational methods.
- Data Sharing Initiatives: Establishing databases that compile PTM data from various studies also promotes knowledge exchange and collaboration. Initiatives that facilitate shared resources can provide researchers with a more robust dataset to refine prediction algorithms.
Emerging technologies combined with innovative interdisciplinary approaches promise to deepen our understanding of PTMs and their implications in health and disease, guiding future research directions effectively. As the community advances these methodologies, the practical implications will be significant across various biological applications.
The End
In the backdrop of nature’s complex architecture, post-translational modifications (PTMs) substantiate the functional adaptability of proteins. This adaptability is paramount in biological systems. The ability to accurately predict PTMs fosters a deeper understanding of cellular mechanisms and allows for innovation in the fields of medicine and biotechnology. In this article, several elements emerged as critical to the accurate prediction of PTMs.
Summary of Key Concepts
First, the essence of PTMs lies in their capability to modify protein functions through various mechanisms, like phosphorylation or ubiquitination. Each type of modification has distinct effects on protein dynamics. The processes of predicting these modifications incorporate a range of methodologies, from machine learning approaches to experimental validation.
Furthermore, identifying the relationship between PTMs and specific diseases illustrates the clinical relevance of this knowledge. Researchers are leveraging predictive models to unveil how these modifications contribute to pathophysiology, thereby directing therapeutic strategies. Hence, understanding the interplay between PTMs and diseases can lead to effective drug discovery.
The Importance of Accurate PTM Prediction
Accurate PTM prediction is not merely academic; it has real-world implications. It accelerates biomarker discovery, influencing early disease detection and monitoring. Additionally, with the rise of personalized medicine, accurate predictions facilitate tailoring treatments to individual patients.
"Understanding PTMs can lead to breakthroughs in targeting diseases at a molecular level."
Moreover, as computational tools improve, the predictive accuracy will increase. However, the ongoing challenge of data quality and availability must be addressed to ensure that predictions align closely with biological realities. Collaborative efforts among researchers, along with advancements in technology, are essential to overcoming these hurdles.