RNA Sequencing Procedure: Detailed Overview and Insights
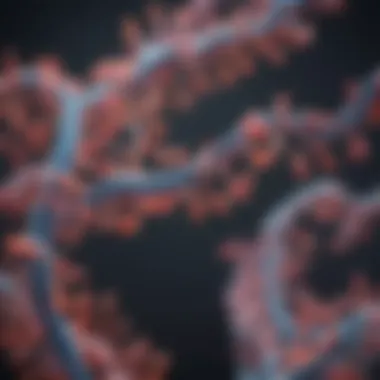
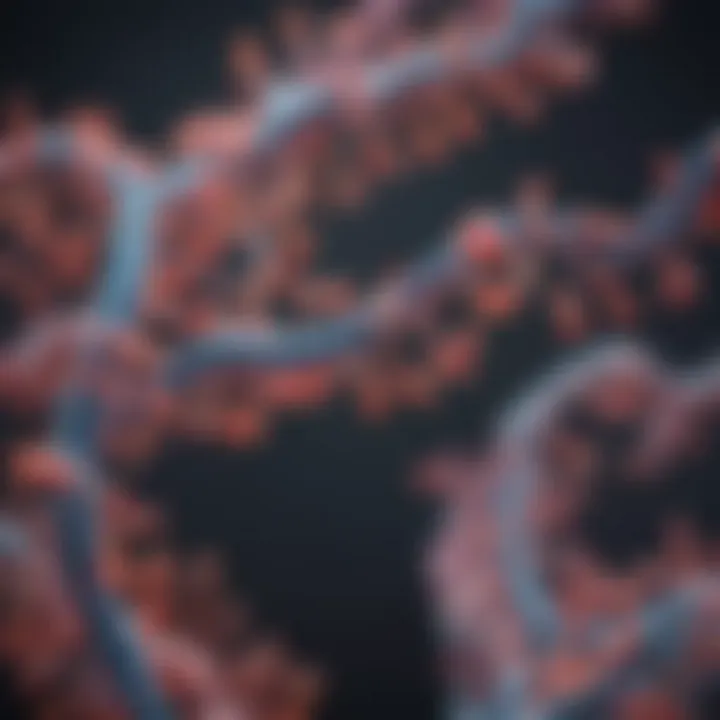
Intro
The landscape of genomic research is constantly evolving, with RNA sequencing emerging as a pivotal technology that profoundly enhances our understanding of gene expression and regulation. RNA sequencing, often shortened to RNA-seq, provides a detailed snapshot of the transcriptome, revealing not only which genes are active but also the levels of activity under various conditions. This methodology has become indispensable in both academic and applied research settings, ranging from basic biological queries to complex medical investigations.
At its core, RNA-seq involves a series of meticulously planned steps that include sample preparation, library construction, sequencing, and data analysis. Each of these phases presents unique challenges and requires specific techniques and tools to ensure accuracy and reliability. The nuances involved can be daunting, especially for those new to the field. Yet, understanding these complexities is crucial for effective application and interpretation of RNA-seq data.
As we unfold the layers of this technology, we will delve into the critical methodologies employed, the implications of findings gleaned from RNA-seq studies, and the future trajectory of research in this dynamic area. Throughout this examination, the aim is to shed light on the practical applications of RNA sequencing and how it tackles current questions in the scientific community. In doing so, we hope to enrich our readers' comprehension and spark interest in the continuing advancements within genomic research.
Understanding RNA Sequencing
RNA sequencing, often known as RNA-seq, is a powerful tool that has revolutionized the field of genomic research. This section serves to clarify what RNA sequencing entails and why it holds such significance in the expanding landscape of molecular biology. Understanding the foundational elements of RNA sequencing equips researchers and scholars with the knowledge essential for diving deeper into its applications and implications.
What is RNA Sequencing?
At its core, RNA sequencing is a technique used to analyze the quantity and sequences of RNA in a biological sample. Unlike DNA, RNA is directly involved in the processes of gene expression and regulation. By capturing RNA levels, scientists can gain insights into which genes are active, under what conditions, and to what extent they contribute to cellular functions.
RNA-seq involves several key steps, starting from isolating RNA from a sample, followed by converting it into complementary DNA (cDNA). This cDNA is then amplified and sequenced. Depending on the technology used, researchers can produce data that reflects the total RNA content or focus on specific subsets, such as mRNA or non-coding RNAs. With modern sequencing techniques, the precision and depth of RNA-seq analyses have come a long way, enabling the detection of low-abundance transcripts and complex transcripts like long non-coding RNAs.
For clarity, here are a few fundamental points about RNA sequencing:
- High Throughput: RNA-seq can process millions of fragments simultaneously, making it much faster than traditional methods.
- Sensitivity: It can detect low-expressed genes, which is crucial in understanding subtle biological changes.
- Versatility: Applicable across various sample types, from tissue biopsies to cell lines, RNA-seq can cater to diverse research needs.
Importance in Genomic Research
The relevance of RNA sequencing in genomic research cannot be overstated. As researchers continue to unravel the complexities of cellular activities and gene expression, RNA-seq emerges as an indispensable tool. It offers several benefits:
- Dynamic Expression Profiling: Unlike static snapshots provided by outdated techniques, RNA-seq captures the dynamic range of gene expression. It allows researchers to observe how genes are expressed differently across various conditions or developmental stages, providing contextual understanding to genomic data.
- Discovery of Novel Transcripts: RNA-seq has opened doors to identifying previously undocumented transcripts and alternative splicing events, helping to decode the complexities of the transcriptome.
- Disease Mechanisms Unlocked: In the realm of disease research, RNA-seq plays a vital role in identifying biomarkers and therapeutic targets, particularly in cancer and genetic disorders. By highlighting differentially expressed genes in diseased versus healthy tissues, insights can be gained that drive forward personalized medicine approaches.
"RNA sequencing paves the way for breakthroughs in understanding cellular processes and disease paths, showcasing the intricate dance of gene regulation and expression."
In summary, understanding RNA sequencing is not just about learning a technique. Itās fundamentally about appreciating how gene activity shapes life at the molecular level, a vital concept for students, researchers, and educators alike. As technology advances, the nuances behind RNA-seq will continue to evolve, further revolutionizing how we approach genomic inquiry.
The RNA Sequencing Workflow
The RNA sequencing workflow serves as the backbone of any RNA-seq analysis, drawing a direct line from sample collection to the generation of insights that help unravel biological mysteries. This process not only provides researchers with a roadmap but also ensures that the results they obtain are robust and reliable. Understanding each step within this workflow is crucial. It allows for effective planning and utilization of resources, ensuring that the data generated can withstand scrutiny. Each phase, from urine to insights, has specific elements that are carefully orchestrated to yield meaningful results.
Sample Collection and Preparation
Before diving into the intricate methodologies of RNA sequencing, one must consider how the journey begins: sample collection and preparation. This phase is vital because the integrity of the sample directly affects subsequent results. Inadequate handling can lead to RNA degradation or contamination, thereby skewing the data. Selecting the appropriate type of sampleābe it from blood, tissue, or even cell culturesārequires thoughtful consideration. Factors like the RNA extraction method or the storage conditions can play a significant role in maintaining RNA quality.
The process typically involves several key steps:
- Choosing the Right Sample: Ensuring that the sample is fresh and representative of the biological question at hand.
- Attention to Detail: Utilizing RNase-free equipment and reagents to prevent contamination.
- Storage Conditions: Samples often need to be frozen or stabilized immediately for later analysis.
In this initial phase, meticulous attention can spell the difference between a successful RNA sequencing endeavor and a muddled venture.
Library Construction
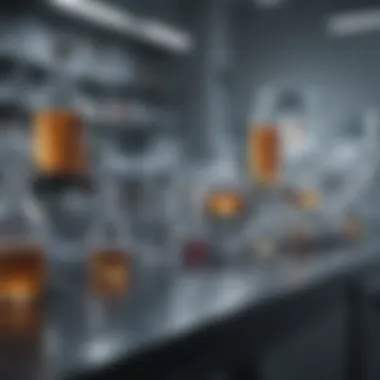
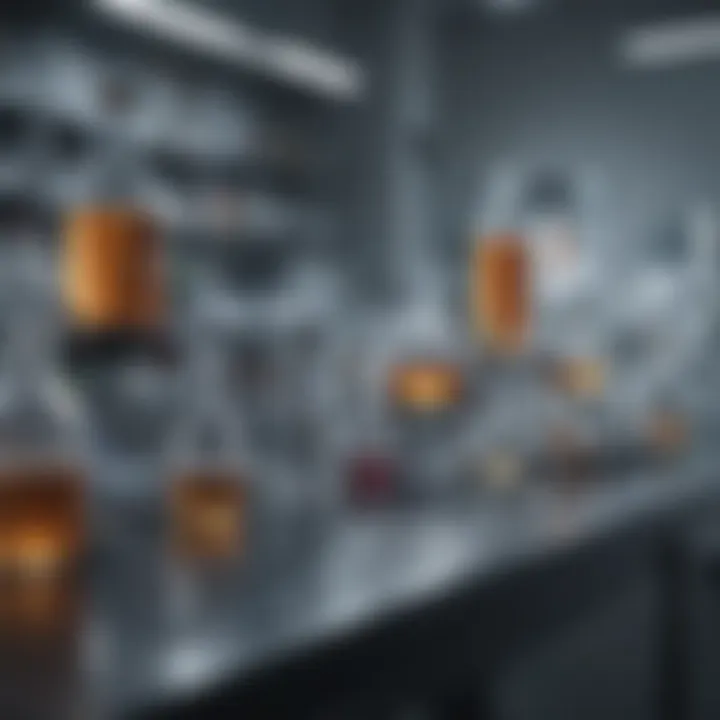
Once samples are procured and prepared with the utmost care, the next step is library construction. This is where the RNA is converted into a form suitable for sequencing. The library, essentially a collection of parts, is crucial because its quality will dictate the information gleaned from the sequencing process. This stage can be divided into several core procedures:
- RNA Fragmentation: This involves breaking down the RNA into smaller pieces that can be sequenced.
- cDNA Synthesis: Reverse transcriptase is used to generate complementary DNA (cDNA) from the RNA fragments, which is more stable and allows for better amplification.
- Adapter Ligation: Adapters are then added to cDNA to facilitate sequencing. These adapters not only allow binding to the sequencing platform but also contain indices that enable multiplexing.
A well-constructed library is akin to a well-planned itinerary; it sets up the entire workflow for success.
Sequencing Techniques
Sequencing techniques bring us to the heart of RNA sequencing. They define how effectively the RNA molecules can be read. Various platforms each come with their sets of advantages and weaknesses, making it essential for researchers to select the right method based on their specific needs. Among these, Illumina Sequencing, PacBio Sequencing, and Oxford Nanopore Technologies stand out for their distinct approaches.
Illumina Sequencing
Illumina sequencing has gained prominence due to its high throughput and accuracy. It uses a sequencing-by-synthesis approach that allows for millions of fragments to be sequenced simultaneously.
- Key Characteristic: The high throughput enables the generation of vast amounts of data quickly, making it suitable for large-scale projects.
- Benefits: Its accuracy and cost-effectiveness also contribute to its popularity among researchers, especially in extensive genomic studies.
- Unique Feature: The ability to capture paired-end sequences allows for improved alignment to reference genomes which is essential in complex analyses such as variant calling.
However, if thereās a drawback, it lies in its inability to handle long reads efficiently, which can be important in the study of certain genomic structures.
PacBio Sequencing
PacBio sequencing, or single-molecule real-time (SMRT) sequencing, is known for its long-read capabilities, significantly aiding in the assembly of challenging genomes.
- Key Characteristic: The ability to sequence longer DNA fragments ensures that complex genomic regions, such as repetitive areas, are captured in their entirety.
- Benefits: This can lead to more accurate annotations and improved insights into gene structures.
- Unique Feature: It also offers circular consensus sequencing, which allows for higher accuracy even in long reads.
Nonetheless, it often comes with a higher cost per base, making it less economical for projects that do not prioritize long reads.
Oxford Nanopore Technologies
Oxford Nanopore Technologies provides an innovative approach by enabling real-time sequencing of DNA or RNA, thereby offering unprecedented versatility in applications.
- Key Characteristic: The ability to sequence in real-time is a game changer. It allows researchers to make decisions based on data as it becomes available.
- Benefits: This can be particularly useful in fieldwork scenarios where timely data access is criticalāsuch as tracking outbreaks.
- Unique Feature: Its portability makes it appropriate for a variety of settings, including remote locations.
On the flip side, while it holds promise, its error rates can be higher than those of Illumina, which might necessitate additional validation steps for critical applications.
Data Generation
In this final stage of the RNA sequencing workflow, raw data is produced, ready to be analyzed. Successful sequencing means that the information is captured accurately, setting the stage for the next steps of quality control, mapping, and eventually unraveling the underlying biology of the sample.
To sum it up, the RNA sequencing workflow integrates various critical components, ensuring that accurate biological insights are generated. Each step, from careful sample collection through diverse sequencing techniques to data generation, plays its part in constructing a clear narrative of cellular activity. In genomic research, where precision is paramount, this workflow provides the structure on which discoveries are built.
Data Analysis in RNA Sequencing
Data analysis is the backbone of RNA sequencing, where raw data morphs into valuable insights about genomic expressions. Once samples have been sequenced, mountains of data are generated, and how researchers handle this data can dramatically influence the conclusions they draw. Understanding the nuances of data analysis is crucial, as it ensures that the findings are both reliable and relevant in the context of specific research objectives.
Quality Control of Data
Before diving into the actual analysis, the proverbial first step is ensuring the quality of the sequencing data. This process is akin to filtering the wheat from the chaff. Low-quality reads can skew results and lead to misinterpretations. Tools like FastQC are employed to gauge the quality of read sequences, looking for various metrics including the sequence length distribution, GC content, and the presence of adapters.
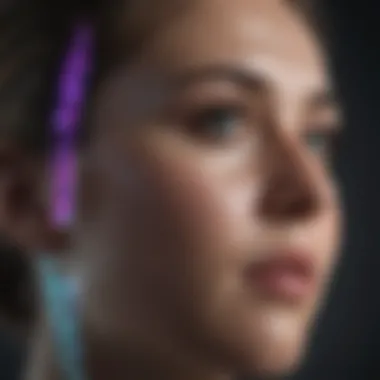
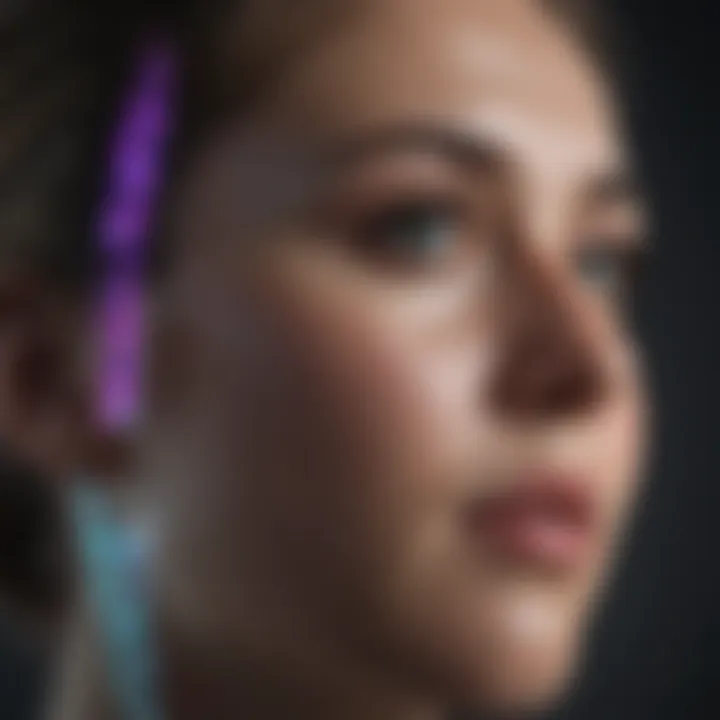
Using trimmers or filters can help eliminate poor-quality sequences, thus preserving the integrity of the data. The process may also involve re-sequencing if the quality does not meet established standards. Itās essential to remember that quality control is not a one-time effort but a repetitive cycle throughout the sequencing process. Regular checks help maintain high standards, which is a recipe for reliable outcomes.
Mapping Reads to Reference Genomes
Once the data quality checks are done, the next step is mapping the reads back to a reference genome. Think of this stage as trying to fit puzzle pieces into their correct locations in an image. Several software tools, like BWA (Burrows-Wheeler Aligner) and HISAT2, are typically used for this mapping task. The objective here is to align each read to its corresponding position in the reference genome. However, it's not always as straightforward as it seems.
Challeges, such as repetitive regions in the genome and variations in read lengths, can complicate the mapping process. A reasonable mapping rate is usually more than 70%, and that still leaves some room for interpretation and adjustment. Once the reads are mapped, discrepancies such as mismatches or gaps need to be scrutinized. This step is foundational, as it establishes the groundwork for further analyses.
Quantification of Gene Expression
With the reads now confidently aligned to a reference genome, researchers can quantify gene expression. This stage turns the focus on how loudly each gene is "shouting" in the resulting data. Utilizing methods like RPKM (Reads Per Kilobase of transcript per Million mapped reads) or TPM (Transcripts Per Million), researchers gain insight into the expression levels of individual genes across different conditions or treatments.
The quantification outcomes are critical for understanding not just which genes are being expressed, but also the extent of their activity. Such quantifications can reveal a wealth of information, including insights into cellular processes, responses to environmental changes, and disease mechanisms. Ensuring accuracy in these measurements is paramount, as statistically meaningful results can only arise from precise quantifications.
Differential Expression Analysis
The final piece of the analysis puzzle is the differential expression analysis, where the real comparisons begin. Here, researchers compare gene expression levels across various conditions, typically involving two or more samples. The aim is to uncover genes that behave differently under distinct conditionsābe it healthy vs. diseased tissue or treated vs. untreated samples.
Tools like DESeq2 and edgeR are designed to identify these differences, often employing statistical models to determine significance levels. A common threshold set at a false discovery rate (FDR) of less than 0.05 helps to pinpoint correctly those genes that show meaningful changes in expression. Itās fascinating how a simple shift in a geneās expression can play a pivotal role in understanding disease pathology or biological mechanisms.
In summary, data analysis in RNA sequencing is a multi-layered process that requires attention to detail at every stage. From quality control to mapping and quantifying gene expression, each phase builds upon the last to provide a cohesive understanding of genomic functions and dynamics. As researchers continue to explore these dynamics, they pave the way for innovations in genomics that hold potential for real-world applications.
Applications of RNA Sequencing
RNA sequencing has become a cornerstone in modern genomics. Its versatility allows researchers and clinicians alike to explore a myriad of applications. By providing an in-depth understanding of the transcriptome, RNA sequencing opens doors to new insights in various fields, from basic research to clinical applications. The implications of these applications stretch far and wide, offering substantial advantages in understanding complex biological systems.
Transcriptome Profiling
Transcriptome profiling is a core application of RNA sequencing. This process involves analyzing all RNA molecules present in a cell at a particular time. Think of it as taking a snapshot, not just of genes being expressed, but how those genes are contributing to cellular functions in real time. This profiling helps in the identification of different isoforms and alternatively spliced transcripts.
Through accurate quantification of various RNA levels, researchers can tailor study designs that delve deep into specific biological conditions. Imagine a scientist tracking how a muscle cell transforms in response to exercise, or how a neuron behaves under stress. By pinpointing gene expression patterns, novel therapeutic targets can emerge. This knowledge might sway the development of drugs that are finely tuned to circumvent disease mechanisms effectively.
Disease Research
One of the most impactful uses of RNA sequencing lies in disease research. It provides a clearer vision of how diseases like cancer or neurodegenerative disorders manifest at the molecular level. When you consider cancer, for instance, the ability to analyze tumor RNA enables researchers to uncover how mutations lead to uncontrolled cell growth.
Moreover, RNA sequencing helps differentiate between tumor types, allowing for better classification. Thereafter, understanding these patterns can aid in the development of diagnostics and prognostics tailored to specific cancer subtypes. Furthermore, RNA sequencing also plays a role in infectious disease research, offering real-time insights into pathogen-host interactions. By analyzing the host's transcriptome during an infection, we can reveal pathways critical for immune response.
"The more we understand RNA, the more we can tailor individual therapies to combat an array of diseases."
Personalized Medicine
Personalized medicine is gradually reshaping treatment modalities across various diseases, and RNA sequencing has become pivotal in this movement. By examining a patientās unique RNA expression profile, clinicians can make more informed decisions regarding therapies. For example, in oncology, within a single patientās tumor sample, RNA sequencing can uncover specific mutations or expression patterns that provide clues for targeted therapies.
In addition, RNA sequencing can assist in predicting therapy responses, greatly enhancing treatment efficacy. When you think about it, each patient is unique - their tumors differ, their responses to drugs vary, and their side effects can be unpredictable. Thus, using RNA sequencing to tailor treatments based on an individual's transcriptomic profile facilitates a more focused treatment approach.
In summary, the applications of RNA sequencing stretch far beyond mere academic interest. From transcriptome profiling to disease research and personalized medicine, this technology is harnessing the potential of our genetic information, thereby advancing our understanding and treatment of complex conditions. As the field continues to evolve, the benefits of these applications will only continue to grow.
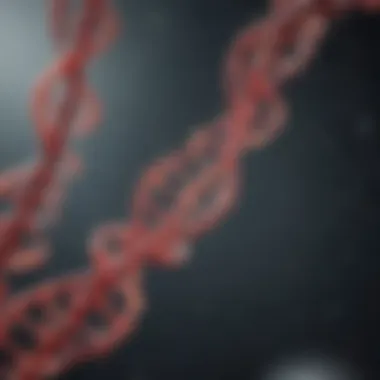
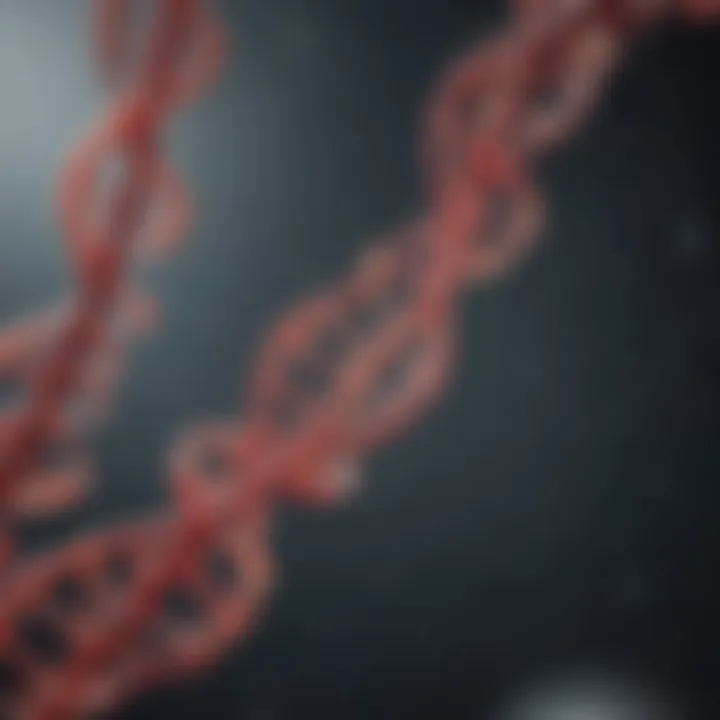
Challenges in RNA Sequencing
RNA sequencing (RNA-seq) has transformed our approach to understanding gene expression and complexity of transcriptomes. However, the path to clarity is not without stumbles. Significant challenges can muddle the insights we seek, rendering interpretation difficult if not impossible. Identifying these hurdles becomes crucial for researchers and practitioners who aspire to harness RNA-seq's power.
Technical Limitations
Navigating the technical landscape of RNA sequencing requires an understanding that not everything is as straightforward as it appears. Here, we dive into some specific hurdles that practitioners face:
- Sample Degradation: RNA is a notoriously unstable molecule. Though it is possible to isolate RNA from various sample types, these molecules degrade quickly under suboptimal conditions. Handling samples with care and implementing rapid processing protocols is essential, yet it remains a technical challenge.
- Bias in Library Preparation: Different protocols for library preparation can introduce biases into the data. Whether itās due to RNA fragmentation, amplification methods, or PCR cycles, these biases might lead to inaccurate representation of low-abundance transcripts. This phenomenon can skew results and mislead conclusions.
- Sequencing Errors: Each sequencing platform has its own error profiles. For instance, somatic mutations might go undetected in the presence of these errors. As discernible as the data might seem, the reliability of results hinges on understanding and mitigating sequencing errors.
This technical landscape may seem daunting, yet overseeing these limitations can lead to more reliable and reproducible results. Paying careful attention to effective sample handling and choosing the right sequencing technology could significantly improve overall output.
Data Interpretation Complexities
After the dust settles from data generation, the next hurdle emerges in the form of data interpretation. Delving into RNA-seq data isnāt just about crunching numbersāthere's a complex web of factors that can distort findings. Consider the following:
- Bioinformatics Requirements: The analysis of RNA-seq data involves substantial bioinformatics expertise. Researchers must navigate intricate tools and software to yield meaningful information from the raw data. This requires an understanding of statistical methods and programming, which can be overwhelming for those who are not specialists in these areas.
- Reference Genome Dependence: The accuracy of mapping reads back to reference genomes can affect quantification. If the reference genome is incomplete or of poor quality, the data gathered could be misleading. For example, annotations might lack crucial functional details, leading to significant oversights in understanding gene roles.
- Variability in Expression: Biological variability between samples can confuse interpretations. A supposed similarity in expression levels may not accurately represent biological differences. Hence, a sharp focus on statistical rigor during differential expression analysis becomes vital to avoid murky conclusions.
"[ā¦] careful statistical modelling is paramount. Overinterpretation or overlooking variability could lead researchers to make assumptions that misinform clinical or further biological decisions."
While the promise of RNA-seq is immense, success in this field rests on the shoulders of a sound understanding of these challenges. Only when technical limitations and data interpretation complexities are thoughtfully addressed, can the true potential of RNA sequencing be realized.
Through education, careful methodology, and collaborative efforts across disciplines, overcoming these challenges is within reach.
Future Directions in RNA Sequencing
The field of RNA sequencing continues to evolve at breakneck speed, and staying abreast of future directions is crucial for researchers and practitioners alike. With advancements bubbling on the horizon, RNA sequencing is poised to become more precise, efficient, and relevant across various domains of biomedicine and genomics.
Advancements in Technology
Advancements in sequencing technology hold the promise of not only enhancing the accuracy of RNA sequencing but also broadening its applications. The innovation in platforms like Illumina, which are already pivotal, has led to improvements in read lengths and sequencing accuracy. Consider the rise of single-cell RNA sequencing; it allows for a granular understanding of gene expression at the individual cell level, which is revolutionary. This advancement makes it possible to discern cellular heterogeneity in tissues, potentially leading to breakthroughs in understanding complex diseases.
Furthermore, technologies like Oxford Nanopore have shifted the game by enabling real-time sequencing and portability, which can particularly benefit field studies where traditional lab setups are impractical. The adoption of artificial intelligence in processing sequencing data is also gaining ground, making analysis faster and reducing the cognitive load on researchers. These enhancements collectively underscore a pressing need for stakeholders in genomics to remain agile and adaptable, ready to integrate new technologies as they emerge.
Interdisciplinary Approaches
Emphasizing interdisciplinary approaches in RNA sequencing is vital for fostering collaborations that could lead to impressive breakthroughs. The integration of computational biology, genomics, and bioinformatics creates a rich tapestry for exploring RNA data. For instance, partnerships between computational scientists and molecular biologists can accelerate the development of novel algorithms for data interpretation, thus enhancing the utility of RNA-seq in terms of insights into gene regulation and expression patterns.
Moreover, a strong collaboration with fields like biomedical engineering or pharmacology can tailor RNA sequencing applications to address specific therapeutic challenges. For example, understanding the RNA profiles of tumors can guide personalized treatments and improve patient outcomes. Bringing together diverse expertise not only enriches the research portfolio but also empowers innovation, enabling more robust solutions to pressing biological questions.
"Integrating multiple disciplines is not just beneficialāit is essential for maximizing the potential of RNA sequencing."
The End
As we bring the discussion on RNA sequencing to a close, it's crucial to reflect on the multifaceted nature of this cutting-edge technique. RNA sequencing is not just about gathering data; itās a profound way to explore the biology of cells at a level that traditional methods simply canāt reach. The insights gained from RNA sequencing have profound implications, particularly in elucidating gene expression patterns, understanding disease mechanisms, and driving personalized medicine.
The key elements to consider when summarizing our journey through RNA sequencing include:
- The Complexity of the Workflow: Each phase, from sample collection to data analysis, is ripe with technical considerations. Mistakes or oversights in any step can compromise the integrity of the results. It shows how important a meticulous approach is in experimental design.
- The Importance of Technology: Advancements in sequencing technologies have dramatically expanded the capabilities of genomic research. For instance, while Illumina is widely known for its high throughput, technologies like PacBio and Oxford Nanopore offer unique advantages such as longer reads that help in resolving complex genomic regions.
- Interpreting the Data: The sheer volume of data generated poses its own challenges. As we've touched upon earlier, quality control and data interpretation can be labyrinthine. Often, complex algorithms and tools are needed to make sense of the noise and extract meaningful conclusions.
- Applications that Matter: From transcriptome profiling to disease research, the applications of RNA sequencing are substantial. Tailoring treatments based on individual expression profiles can revolutionize healthcare strategies, shifting focus from one-size-fits-all approaches to more personalized options.
"The future of medicine lies not in treating conditions, but in understanding individual biology through innovative technologies like RNA sequencing."
In consideration of all these facets, it is evident that RNA sequencing is more than a simple procedure; itās a game-changing tool that continues to shape the landscape of genomic research. As the field evolves, we must remain vigilant about the ethical implications, especially regarding data privacy and potential misuse in healthcare. This consideration will ensure that the benefits of RNA sequencing can truly reach their potential without compromising the trust of those who contribute to this vital research and data collection.