Understanding RNA Sequencing Volcano Plots in Genomics
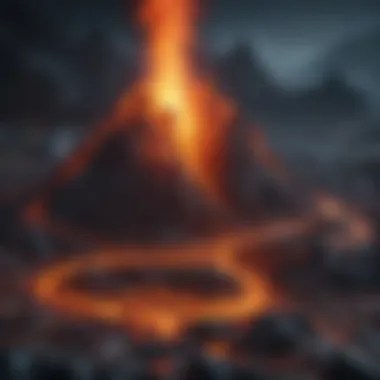
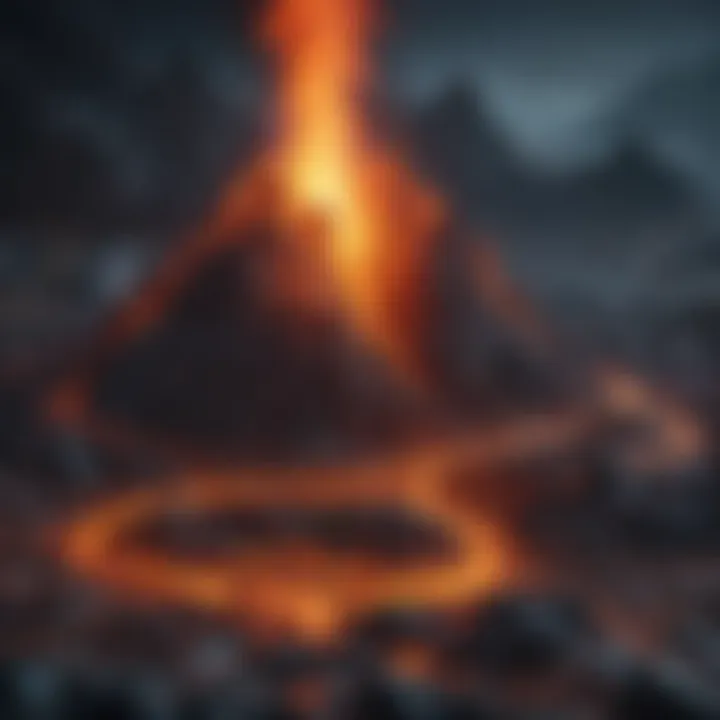
Intro
In the realm of genomics, the ability to comprehend the nuances of gene expression is like finding a needle in a haystack but with more advanced tools. RNA sequencing has kicked down the doors of traditional research methods, providing researchers with a fresh perspective on how genes interact and express themselves. Among the trove of tools at their disposal, the volcano plot stands out. It acts not just as a visual aid but a beacon, guiding scientists through the vast landscape of RNA-seq data. By illuminating differentially expressed genes in a visually arresting way, these plots have reshaped how we identify potential biomarkers and therapeutic targets.
Research Overview
Methodological Approaches
Digging into the intricacies of RNA sequencing reveals a mosaic of methodologies intertwined to capture the essence of gene expression. The process begins with the extraction of RNA, followed by conversion to complementary DNA (cDNA), and onto the sequencing stage. Each step is a piece of the puzzle that culminates in a treasure trove of data.
Once the raw data is gathered, it is transformed through a series of analytical steps including normalization, filtering, and statistical analysis. The volcano plot emerges as an essential component of this analytical framework. In essence, it plots statistical significance against the magnitude of change, making it easier to spot outliers—genes that are either up-regulated or down-regulated significantly in response to biological conditions. This twofold representation, often displayed in a scatter plot format, allows for immediate visual insights into the data.
Significance and Implications
Volcano plots do not simply serve as decorative pieces in research papers; they hold immense significance. By capturing the exponential changes in gene expression levels while considering the statistical robustness of those changes, they empower researchers. Precise identification of these differentially expressed genes provides pathways to understanding complex diseases. Take, for instance, cancer research. These plots can highlight key genes involved in tumor progression, offering targets for further investigation.
To summarize, the significance of volcano plots in RNA sequencing is unparalleled. They help to streamline the interpretation process, pointing researchers in the right direction. As a result, this methodology paves the way for more targeted research initiatives, ultimately improving our understanding of genetic underpinnings.
Current Trends in Science
Innovative Techniques and Tools
With the continuous evolution of genomic technologies, innovative techniques are continually augmenting the effectiveness of RNA-seq analysis. For instance, advancements in artificial intelligence are enabling more refined interpretations of RNA-seq data. Machine learning algorithms can enhance the analysis of volcano plots by predicting gene interactions and identifying subtypes of diseases based on expression patterns.
Furthermore, tools that provide interactive capabilities for plotting and visualizing data have gained traction. Platforms such as R’s ggplot2 and GraphPad Prism offer intuitive interfaces for generating volcano plots, making them accessible even to those who might not be statistically savvy.
Interdisciplinary Connections
The fields of bioinformatics, statistical genetics, and molecular biology are merging in exciting ways, and RNA sequencing volcano plots serve as a nexus point. Collaborative efforts across disciplines are driving the development of new analytical methods and software designed to improve data accuracy and interpretation. These interactions are helping to foster a more holistic view of genomic research where data analytics, applied biology, and clinical implications converge.
In closing, RNA sequencing volcano plots are more than just graphical representations; they serve as a vital link between raw data and impactful scientific findings. By evaluating their construction, interpretation, and potential applications, we can further appreciate their core role in modern genomic research.
Intro to RNA Sequencing
The realm of genomics has undergone significant transformations in recent years, with RNA sequencing playing a pivotal role in our comprehension of gene expression. As researchers dive into the molecular intricacies of living organisms, RNA sequencing emerges as a powerful tool, providing clarity and resolution that traditional techniques simply cannot match. The importance of RNA sequencing in this discussion cannot be overstated, as it forms the backbone for subsequent analysis, including the interpretation of differential gene expression through visual aids like volcano plots.
The advent of RNA sequencing technology has marked a considerable leap in research efficiency and accuracy. By allowing scientists to quantify RNA levels across various conditions, it enables them to discern not only how much of a specific RNA is present but also to contextualize that information against other genes. This holistic view of transcriptomes has opened the door to a myriad of applications, from biomarker discovery to drug development.
When we consider RNA sequencing, several key aspects demand our attention:
- Versatility: This technology isn't confined to a single application but spans various fields, including oncology, neurology, and infectious disease research.
- Precision: Unlike earlier techniques like microarrays, RNA-seq can detect subtle changes in gene expression, offering a finer lens into cellular processes.
- Scalability: As costs continue to drop, RNA-seq becomes increasingly accessible to labs around the world, democratizing research opportunities.
Consequently, how one interprets this data is equally vital. Volcano plots, which graphically represent differential expression data, play a significant role in making sense of the mountains of data generated by RNA-seq experiments. The statistical rigor combined with the visual insight these plots provide makes them indispensable in modern genomic research.
Ultimately, understanding RNA sequencing is not merely about the technology itself but about recognizing its implications for future research. As the science progresses, the role of RNA sequencing and toolsets like volcano plots will indelibly shape our insights into the fundamental mechanisms of life.
Understanding Volcano Plots
In the realm of genomic research, the ability to visualize complex data is like having a secret weapon. Volcano plots serve as a vital tool, providing insights into gene expression changes. Their design allows scientists to quickly assess the significance and magnitude of these changes. By illustrating the relationship between the statistical significance of results and the magnitude of change, these plots help researchers to make informed decisions. Whether for identifying potential biomarkers or understanding disease mechanisms, mastering volcano plots can be essential for any researcher in the field.
Fundamentals of Volcano Plot Construction
Diving into the basics of volcano plot construction reveals the heart of their utility.
Axes and Coordinates
To start, the axes play a crucial role. The x-axis typically shows the log2 fold change, while the y-axis represents the -log10 p-value. This format is a clever choice because it allows for a straightforward interpretation; larger fold changes appear farther on the x-axis, while more statistically significant results soar upward on the y-axis.
A key characteristic of this setup is its clarity; researchers can easily spot genes that exhibit both significant expression changes and strong statistical evidence. The unique feature here is that this dual representation enables an efficient overview of large datasets, filtering the noise from relevant data. Nonetheless, some researchers might face challenges if they do not appropriately scale the axes, potentially missing critical insights from the data.
Selection of Data Points
Another important facet of volcano plots is the selection of data points. Choosing which genes to highlight can significantly affect the plot's usefulness. Often, researchers will filter for genes that meet specific criteria, such as a p-value threshold or a fold change criterion. This selective filtering is pivotal to ensuring that only the most relevant data is presented.
The key characteristic of this selection process is that it prioritizes clarity and focuses on the most significant findings. The unique feature of targeted data selection lies in its potential to reduce clutter and enhance interpretability. However, it can also be a double-edged sword; stringent filtering may eliminate outliers or noteworthy genes, potentially causing researchers to overlook important discoveries.
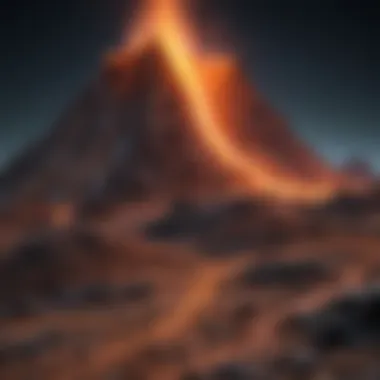
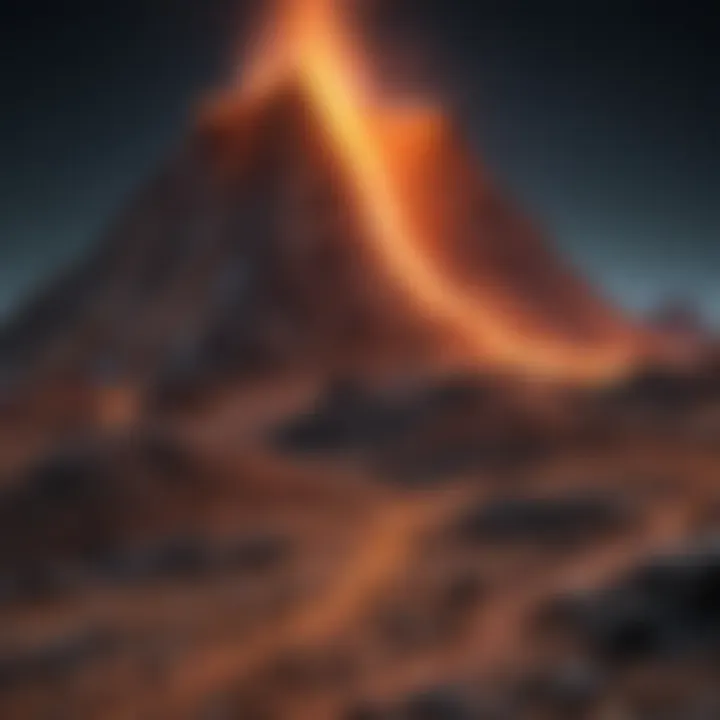
Statistical Significance in Volcano Plots
Statistical significance underpins the effectiveness of volcano plots, guiding researchers toward trustworthy results.
P-values
P-values are at the forefront of assessing statistical relevance. They measure the probability that the observed data could occur if the null hypothesis were true. In the context of volcano plots, a low p-value, typically below 0.05, is often regarded as indicative of significant differential expression. This characteristic, however, may lead researchers to a trap: over-reliance on these values can cause one to overlook the biological relevance of the findings.
The unique framework of presenting p-values in a logarithmic scale amplifies their distinctions on the plot, offering a visual cue to the most statistically significant genes. However, while they provide crucial insights, the over-simplification of this aspect could sometimes mislead interpretations if biological context is not examined concurrently.
False Discovery Rate
On the other hand, the false discovery rate (FDR) addresses the concern of multiple comparisons. It estimates the proportion of false positives among the declared significant results. This is a crucial aspect, especially in high-dimensional data where many tests can inflate the likelihood of finding false positives.
The key characteristic of FDR is its ability to adjust for the fact that multiple tests are being conducted simultaneously. Unique to this method is its application in prioritizing genes for further study based on their biological importance and not just their statistical significance. Despite its advantages, one must remember that a focus solely on FDR may lead to overlooking significant proteins that show compelling biological implications but do not pass the statistical bar.
Visual Elements and Design Considerations
The visual aspect of volcano plots is not merely decorative; it has substantial implications for data interpretation.
Color Coding
Color coding can dramatically improve readability. Assigning specific colors to genes based on their significance, such as highlighting upregulated genes in red and downregulated ones in blue, provides an immediate visual cue for researchers. This enhanced clarity can help in quickly identifying trends and patterns.
The key characteristic of color coding hinges on its ability to facilitate instant comprehension of results. Each color serves a unique narrative, telling the story of gene expression changes at a glance. However, there is a caveat; poor color choices can sometimes lead to confusion or misinterpretation. Ensuring proper contrast and colorblind-friendly palettes is paramount to prevent any misunderstanding from arising.
Point Size and Shape
Lastly, point size and shape are also instrumental in conveying different data dimensions. By varying the size of point markers to indicate the magnitude of change or additional data, such as the number of biological replicates, researchers can create a richer visual experience. This approach allows an extra layer of depth to the presentation, making it more informative.
The key characteristic of manipulating point sizes is allowing for more nuanced comparisons among genes. It gives researchers the ability to emphasize particularly notable changes in expression without cluttering the visual space. However, if not balanced well, varying sizes may lead to oversaturation, detracting from the overall clarity of the plot.
Interpreting Volcano Plots
Interpreting volcano plots is a critical step in the analysis of RNA sequencing data. These plots serve as a visual representation of the complex relationships between gene expression levels and statistical significance, making it easier for researchers to discern important patterns in the data. To decode the information presented in these visuals, understanding several core components is essential.
Identifying Differentially Expressed Genes
Differential gene expression (DGE) analysis is the heart and soul of many studies in genomic research. Volcano plots enable scientists to pinpoint genes that exhibit significant changes in expression between experimental conditions. The classic way to observe this is by positioning genes on the plot based on their log-fold changes (x-axis) against their -log10 p-values (y-axis).
- Thresholds: Researchers typically set thresholds to highlight genes of interest. For example, a common practice is to consider genes above a certain log-fold change and below a specific p-value as differentially expressed. These thresholds help to filter out noise and focus on biologically relevant changes.
- Highlights: In a volcano plot, genes that are highly expressed in one condition relative to another will appear towards the right or left extremes. Genes that show notable significance will soar towards the top of the plot. By visually scanning these plots, researchers can quickly identify potential biomarkers or therapeutic targets.
Understanding the Biostatistical Significance
The quantification of gene expression changes brings statistical vigor to the interpretations derived from volcano plots. The concept of statistical significance is crucial here, providing a means to assess whether observed differences are genuine or merely artifacts of variability in the data.
- P-values: Each point in the volcano plot is often associated with a p-value which indicates the probability that the observed expression change happened by chance. Researchers usually follow a conventional threshold of 0.05, but depending on the study, more stringent criteria may be applied.
- False Discovery Rate (FDR): Beyond p-values, correction methods for multiple testing, such as the Benjamini-Hochberg procedure, help control the false discovery rate. This ensures that the list of differentially expressed genes reflects true expressions rather than random fluctuations.
Understanding these components allows researchers to make informed decisions about which genes warrant further exploration, profoundly influencing the direction of subsequent experiments.
Potential Limitations and Biases
While volcano plots are powerful tools, they come with limitations that can obscure the clarity of the data. Recognizing these potential pitfalls is crucial for any researcher looking to utilize this visualization method effectively.
- Data Quality: Poor quality RNA-seq data can skew results. Artifacts from sample preparation, sequencing errors, and batch effects can introduce biases that misrepresent gene expression levels.
- Interpretation Challenges: Not all visually striking genes warrant biological relevance. Some genes may pass the statistical thresholds without meaningful biological implications. Researchers must resist the temptation to place undue emphasis on outliers without further validation.
- Overlapping Factors: External factors like environmental changes or co-regulatory mechanisms can influence gene expression, complicating the interpretation of results.
"Presenting your findings accurately can be the difference between a breakthrough discovery and a pathway leading nowhere."
In summary, interpreting volcano plots requires a careful balance of insight and skepticism. Identifying differentially expressed genes, understanding the statistical significance of findings, and acknowledging limitations are integral to drawing meaningful conclusions from RNA-seq datasets. This layered interpretation equips researchers to navigate the intricate landscape of gene regulation and expression.
Practical Applications of Volcano Plots
Volcano plots serve as a vital tool in the realm of genomic research, especially in presenting complex RNA sequencing data in a way that is both interpretable and actionable. The necessity of efficient visualization of differentially expressed genes cannot be overstated, particularly as the volume of transcriptomic data generated continues to escalate. The following sections delve into three key areas where volcano plots find practical application: biomarker discovery, drug development, and disease mechanism research.
In Biomarker Discovery

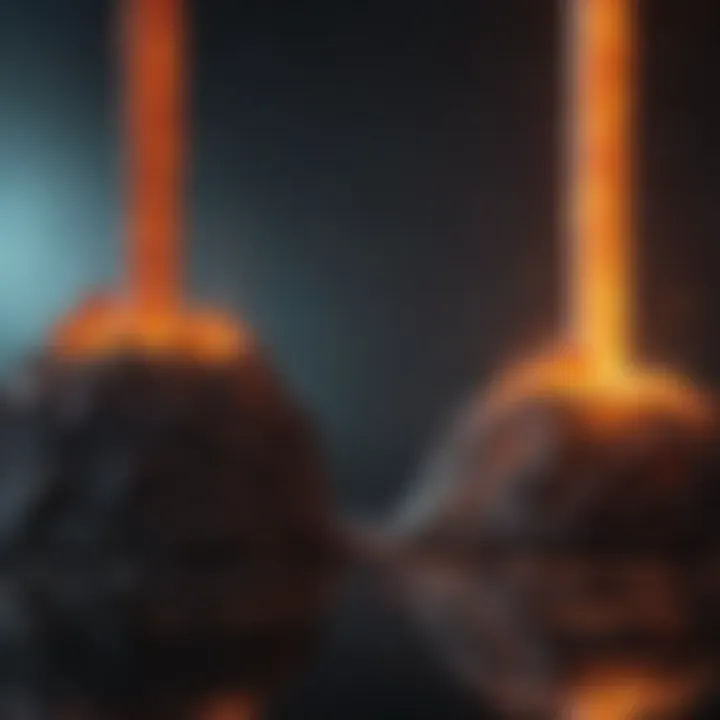
Identifying biomarkers is central to the advancement of personalized medicine. Volcano plots streamline this process by highlighting genes that exhibit statistically significant differences in expression levels between groups. This capability is crucial for researchers seeking to pinpoint potential biomarkers that can serve as indicators for disease presence or progression.
- Focus on Key Genes: The visual nature of volcano plots allows researchers to swiftly identify genes of interest that lie outside predefined thresholds. This aids in narrowing down the list of candidates for further validation.
- Comparison Across Conditions: By visualizing gene expression profiles across different conditions (e.g., healthy vs. diseased), researchers can also ascertain whether the identified biomarkers are consistent and reliable across varying contexts.
In summary, volcano plots enhance the biomarker discovery process, allowing researchers to filter vast datasets and identify the most promising candidates for clinical application.
In Drug Development
In the pharmaceutical industry, the path from concept to market is fraught with challenges. Volcano plots play a significant role in drug development, particularly during the screening of therapeutic targets.
- Target Identification: By comparing the gene expression levels in treated versus untreated samples, researchers can identify potential targets for new drugs. The plots clearly delineate which genes are up-regulated or down-regulated in response to treatment, directing focus toward the most relevant pathways.
- Dose-Response Analysis: Volcano plots can also illustrate the effects of different drug concentrations on gene expression. This helps in understanding dose-response relationships and further refining targeted therapies.
With their ability to visually summarize complex data, volcano plots reduce the likelihood of missing critical insights during drug discovery and streamline the subsequent stages of drug development.
In Disease Mechanism Research
Understanding the underlying mechanisms of diseases requires comprehensive investigation into the various molecular pathways involved. Volcano plots contribute significantly by enabling researchers to visualize the wide-ranging effects of pathological conditions on gene expression profiles.
- Pathway Analysis: Researchers can utilize the plots to explore how specific genes are involved in known or novel pathways related to diseases. This can lead to the discovery of new pathogenic mechanisms and may even highlight unexpected targets for intervention.
- Longitudinal Studies: In studies assessing the progression of diseases, volcano plots can be employed to track gene expression changes over time, providing insights into how diseases evolve and informing treatment strategies accordingly.
In essence, volcano plots not only facilitate the identification of the gene expression alterations linked to various diseases but also foster a deeper understanding of their biological mechanisms, thus catalyzing breakthroughs in the field.
Tools and Software for Volcano Plot Visualization
Navigating the world of RNA sequencing and volcano plots requires an understanding of the essential tools and software that can make this complex data more approachable. These tools not only facilitate the creation of volcano plots but also enhance the clarity and comprehension of the underlying data. Their importance cannot be overstated, especially when dealing with large datasets that require efficient processing and vivid visualization. Researchers need reliable software that caters to their specific needs, allowing for insightful interpretation and application. Here are the key areas concerning tools and software for volcano plot visualization:
- Accessibility: The software must be user-friendly, enabling researchers with varying levels of expertise to visualize their data effectively.
- Integration: The ability to integrate results from RNA-seq analysis seamlessly with visual tools is crucial, ensuring that the depicted results are accurate and informative.
- Customization: Tools that allow for tailored visual design can communicate results more compellingly, attracting attention to significant findings.
Review of Popular Software Packages
R and Bioconductor
R and its associated ecosystem, Bioconductor, play a pivotal role in gene expression analysis, including the construction and visualization of volcano plots. One of the most compelling aspects of R is its flexibility; R can accommodate packages specifically designed for RNA-seq data. Bioconductor provides a plethora of packages that ease the complexity of sequences analyzing.
A standout feature of R is its extensive statistical capabilities combined with powerful visualization tools. R enables researchers to create high-quality graphics using ggplot2, for instance—an industry favorite.
Advantages of R and Bioconductor:
- Robust statistical analysis capabilities tailored for genomic data.
- Extensive resources and tutorials available through the Bioconductor community.
- Highly customizable visual outputs.
Disadvantages:
- Steeper learning curve for those new to programming.
- Potential overhead in installation and package updates.
Python Libraries
Python has gained traction as an alternative to R, particularly because of its straightforward syntax and vast libraries tailored for scientific analysis. Libraries like Matplotlib and Seaborn are instrumental in creating volcano plots. Python promotes ease of use and offers excellent support for integrating machine learning, which can be advantageous for more intricate analyses in genomic research.
Key characteristics include its versatility, allowing for easier debugging and extension of functionalities through multiple libraries tailored for bioinformatics.
Advantages of Python Libraries:
- A gentler learning curve for entry-level coders, making it accessible.
- Strong compatibility with other scientific libraries and machine learning frameworks.
- Excellent documentation and community support.
Disadvantages:
- May not for specialized genomic analyses to the depth that R provides.
- The need to combine multiple libraries, which might complicate some workflows.
Customization Options for Enhanced Visuals
Customization plays a significant role in enhancing the effectiveness of volcano plots. A well-tailored volcano plot can draw attention to specific genes that exhibit differential expression and can illustrate significant trends within the data.
Key aspects of customization include:
- Color Schemes: Utilizing distinct color palettes helps in demarcating key data points, particularly when highlighting up-regulated and down-regulated genes. This makes it easier for audiences to comprehend the results quickly.
- Labeling: Adding clear labels to significant points can enhance the plot's clarity. Reporters might note the notable genes that catch the eye in publications or presentations.
- Sizing: Varying the size of the points in the plot based on the statistical significance (like p-values) or expression levels can visually convey the impact easily.
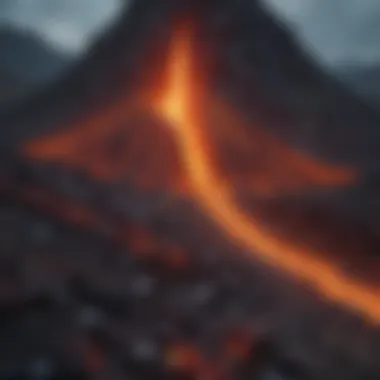
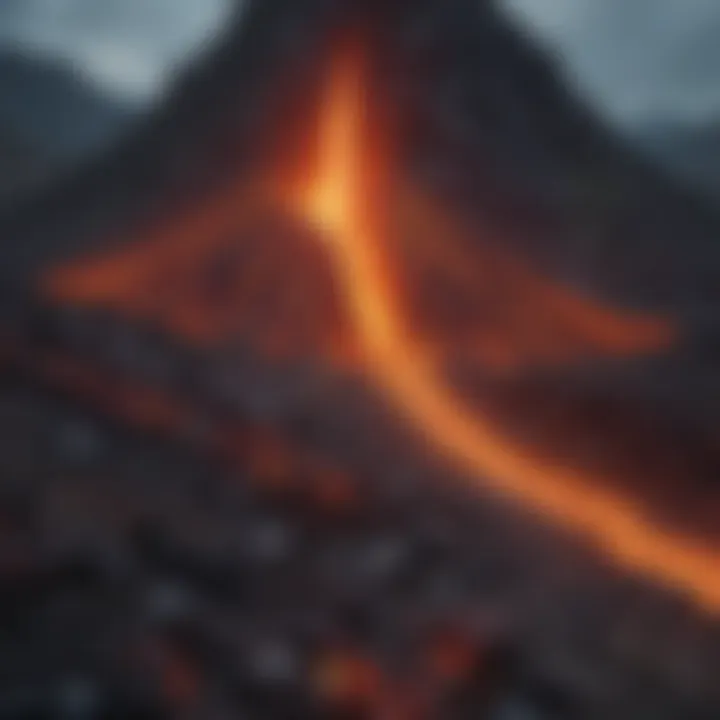
Integration with Other Data Visualization Tools
Integration with other data visualization tools maximizes the use of volcano plots in presenting RNA-seq data. Tools such as Tableau or even web-based platforms like Plotly can complement volcano plots by offering advanced features for interactive dashboards.
When volcano plots are integrated with other graphics, the interplay can lead to richer stories about the data. This synergistic approach benefits researchers, allowing them to highlight correlations and trends that might not be evident in standalone plots.
"In the world of genomic data, the right visualization tools can transform complex datasets into comprehensible insights."
By understanding the landscape of software available and leveraging their unique features, researchers can pave the path towards more effective analysis and interpretation in their studies.
Case Studies Involving Volcano Plots
In the realm of genomic research, volcano plots provide pivotal insights that can shape the direction of numerous studies. These plots are not just a collection of points on a graph; they serve as a bridge between raw data and meaningful biological interpretations. Case studies illustrate their significance and demonstrate the practical applications and outcomes of this visualization tool across various research domains.
Cancer Research Examples
Cancer genomics has embraced volcano plots as a standard tool for making sense of complex RNA-seq data. For instance, a study investigating breast cancer highlighted differentially expressed genes using a volcano plot that effectively showcased the dramatic fold changes in expression levels between tumor and normal tissues. The researchers were able to visually identify potential oncogenes and tumor suppressor genes, thereby steering them toward further validation.
This plotted representation allows researchers to observe both statistical significance and biological relevance at a glance. A key takeaway from such studies is that volcano plots can highlight outliers and patterns indicating specific gene involvement in cancer pathways, enhancing the potential for personalized therapy.
Neurological Disorder Studies
When examining neurological disorders such as Alzheimer’s disease, researchers sometimes grapple with the intricate interplay between genetics and environmental factors. A recent application of volcano plots in analyzing gene expression data from patient-derived neurons has shed light on distinguishing markers associated with disease progression. The plot visually separated genes with significant changes, directing attention to neuroinflammatory processes that could be central to therapeutic intervention.
In this context, volcano plots not only support identification of potential biomarkers but also provide a framework for understanding underlying mechanisms. By presenting complex data in a digestible format, these plots enable researchers to communicate their findings with greater clarity, which is essential when pursuing collaborations or presenting experiments at conferences.
Infectious Disease Approaches
In the world of infectious diseases, understanding host response can be crucial. Studies on viral infections have effectively utilized volcano plots to decipher the host transcriptomic response to pathogens. For example, a recent investigation into the response of human cells to the Zika virus employed volcano plots to reveal subsets of genes that were significantly up- or down-regulated post-infection.
The observations gleaned from these plots often lead to essential questions about potential therapeutic targets or pathways involved in infection. The clarity of visualizing large amounts of data fosters a deeper understanding of complex interactions and guides the development of vaccines or antiviral drugs.
The systematic use of volcano plots in research empowers scientists to pinpoint critical genes and pathways, which can ultimately accelerate the development of innovative treatments.
Future Directions in RNA-seq Analysis
As we look to the horizon of genomic research, the implications of RNA sequencing (RNA-seq) continue to reveal themselves in ever-expanding ways. The significance of understanding future directions in RNA-seq analysis cannot be overstated; it offers the lenses through which scientists can envision and shape the next phases of research. While current methodologies yield rich data, embracing novel trends and technologies stands as a critical step towards deeper comprehension of transcriptomics and associated biological processes.
Emerging Trends in Transcriptomics
The landscape of transcriptomics is shifting, fueled by advancements in technology and a growing recognition of its potential. Single-cell RNA sequencing is paving the way for dissecting cellular heterogeneity, allowing researchers to study gene expression at the individual cell level. This granularity unveils heterogeneity in cellular responses that bulk RNA-seq might obscure. As data resolution matures, so does the potential for personalized medicine, where treatments can be tailored based on the specific transcriptomic profiles of patients.
Moreover, long-read sequencing technologies such as those from Pacific Biosciences and Oxford Nanopore are disrupting traditional short-read methods. These platforms can capture full-length transcripts, providing a more complete representation of gene expression and isoform diversity. The implications here are profound – we are not only looking at more accurate sequencing of known genes but also uncovering novel transcripts, which can have significant biological insights.
"Embracing new technology allows us expand our understanding manifold - like opening a hidden door to a new room full of possibilities."
Innovative Visualization Techniques
Visualization remains a cornerstone of RNA-seq analysis, especially as datasets grow in complexity. Emerging visualization techniques are enhancing the way we interpret and present RNA-seq data. For example, tools that allow for interactive exploration of volcano plots or heat maps enable researchers to tailor their views to highlight specific patterns or disparities in gene expression across different experimental conditions.
Interactive visualizations, like those offered by tools such as Plotly or Shiny in R, empower researchers to engage with data more dynamically. This interactivity fosters better understanding and communication of complex data, which is crucial for collaborative efforts among multidisciplinary teams. Enhanced dimensionality reduction techniques, such as t-SNE or UMAP, are also gaining traction, simplifying high-dimensional data into visual formats that are easier to digest.
Integrating Methods for Comprehensive Analysis
The future of RNA-seq analysis will not be defined solely by new technology, but also by the integration of various data types. By combining RNA-seq data with other omics—like proteomics or metabolomics—researchers can achieve a more holistic bioinformatics approach. This integrative perspective can improve our understanding of cellular activities and how they change in response to environmental shifts or treatments.
Furthermore, the application of machine learning techniques stands to revolutionize the analysis of RNA-seq data. By leveraging algorithms capable of identifying patterns in complex datasets, researchers can refine their predictive modeling for gene expression under different conditions. The synergy of RNA-seq data with AI could potentially lead to breakthroughs in biomarker identification and therapeutic discoveries, providing new avenues for precision medicine.
Culmination
In essence, volcano plots allow for a clearer understanding of differential gene expression. They help scientists pinpoint genes that may serve as biomarkers or therapeutic targets, driving the research forward in critical areas like cancer or neurological disorders. This visual representation aids in overcoming the challenges posed by large datasets, making it simpler for researchers to interpret results accurately and efficiently.
Summary of Key Insights
In summary, RNA sequencing volcano plots hold substantial value across various fields of genomic research. Here are some concise insights that encapsulate the discussions:
- Enhancement of Data Interpretation: Volcano plots facilitate the quick identification of significantly expressed genes by showing the relationship between fold change and statistical significance.
- Statistical Rigor: These plots incorporate statistical measures such as P-values and False Discovery Rates to ensure robust conclusions regarding gene expression changes.
- Applications Across Disciplines: Their versatility is evident in applications ranging from biomarker discovery to insights into complex diseases, including multifactorial conditions.
The Role of Volcano Plots in Future Research
Looking ahead, volcano plots will continue to play an essential role in guiding genomic studies. With advancements in RNA sequencing technology and burgeoning datasets, the demand for effective visualization methods will grow. These plots can adapt to emerging trends in transcriptomics and multi-omics, providing crucial insights into gene interactions and regulatory mechanisms.
Moreover, incorporating innovative visualization techniques can provide even more nuanced insights. As researchers aspire to uncover the detailed biology behind diseases and therapeutic responses, volcano plots will undoubtedly evolve, enhancing their functionality and applicability. The integration of these methods into comprehensive analysis strategies will pave the way for breakthroughs that enhance our understanding of biology at a genetic level.