Exploring Whole Genome Data: Insights and Implications
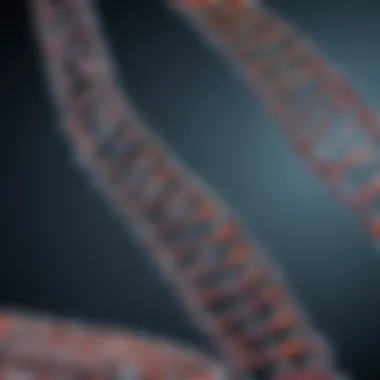
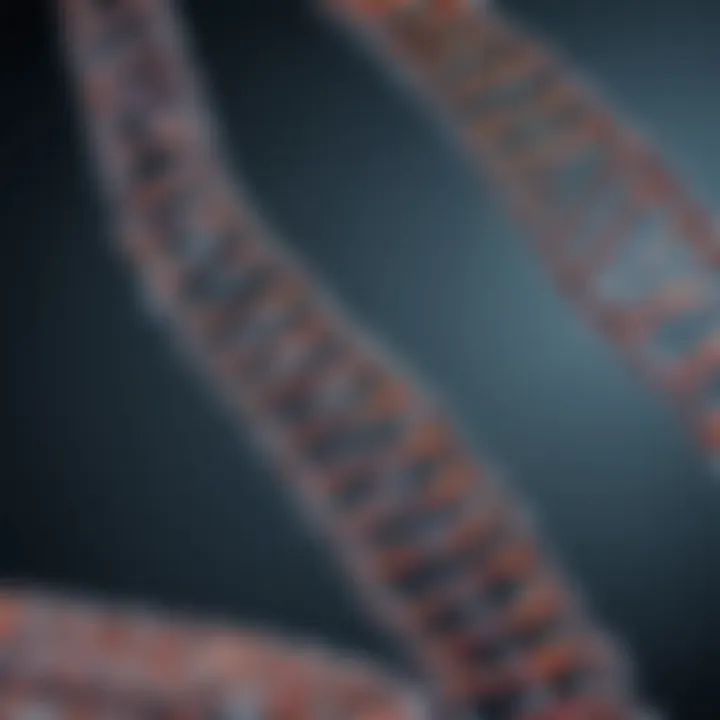
Intro
In today’s rapidly advancing scientific landscape, whole genome data has emerged as a cornerstone of research and application in genomics. By studying the complete set of genes and their variations within an organism, researchers can gain profound insights into areas such as disease susceptibility, evolutionary biology, and even population genetics. This exploration isn't just confined to academic realms; it carries significant implications for personalized medicine, public health initiatives, and ethical discussions that ripple through society.
The relevance of whole genome sequencing (WGS) extends beyond just numbers and sequences on a computer screen. It delves into the very fabric of life, unraveling how genetic material interacts with environmental factors. As the science scales new heights, understanding the implications of this data becomes crucial for students, researchers, educators, and professionals alike. This article aims to dissect these nuances, exploring methodological approaches, current trends, and the broader impacts of whole genome data.
Research Overview
Methodological Approaches
The landscape of genome research is influenced by numerous advanced methodologies. Techniques used for genome sequencing have evolved significantly—from the early days of Sanger sequencing to the more recent advancements in next-generation sequencing (NGS). For instance, NGS offers a much higher throughput, allowing for the simultaneous sequencing of millions of DNA fragments. This is akin to collecting a massive library of genetic information without the tedious effort of reading one book at a time.
Data storage and analysis represent another layer of complexity. With the increasing volume of whole genome data generated, researchers face significant challenges related to data management. Solutions like cloud computing and high-capacity storage systems have gained traction. Not just that, cutting-edge bioinformatics tools help researchers sift through this vast ocean of information, identifying patterns and associations essential for drawing meaningful conclusions.
Significance and Implications
Whole genome data holds enormous potential to transform healthcare. For example, understanding individual genetic variants helps tailor treatments specifically suited to a person’s genetic makeup—this approach is what many refer to as personalized medicine. Moreover, genomic data can enhance public health responses by identifying at-risk populations for certain diseases. As such, one could argue that genomic information is not merely data; it's a treasure chest of insights waiting to be explored.
Furthermore, there are ethical dimensions to consider. The implications of accessing and using such personal data stir up discussions about privacy and consent. Regulatory frameworks may need to adapt to safeguard individual rights while enabling scientific progress.
Current Trends in Science
Innovative Techniques and Tools
Current trends in genome research highlight the introduction of innovative tools that enhance the quality and efficiency of genomic studies. The advent of CRISPR technologies has revolutionized gene editing, allowing scientists to make precise alterations to DNA sequences. It's as if researchers were given a pair of scissors to cut and paste genetic material at will.
Additionally, artificial intelligence plays an increasingly pivotal role in analyzing whole genome data. Algorithms can predict which genetic variants contribute to diseases, providing researchers with remarkable predictive capabilities.
Interdisciplinary Connections
The implications of whole genome data extend far beyond biology. Cross-pollination of knowledge from disciplines such as data science, computer science, and ethics is crucial in fully understanding this data. This interdisciplinary approach fosters collaboration and innovative solutions for challenges encountered in genomics research. For example, partnerships between geneticists and data analysts lead to refinements in interpreting complex genomic datasets.
In summary, the depth and breadth of whole genome data unveil a myriad of insights and implications for science and society. By keeping an eye on advancements and ethical considerations, stakeholders can ensure that the pursuit of knowledge aligns with the common good.
Preamble to Whole Genome Data
The realm of genomics has undergone a significant transformation over recent years. One of the most groundbreaking aspects of genetic research is the rise of whole genome data. This innovation is not just about crunching numbers or knitting together sequences of DNA; it has profound implications that extend into various fields, ranging from personalized medicine to agricultural advancements. Whole genome data enables scientists and researchers to peel away layers of complexity, unlocking the secrets that lie within the DNA of various organisms. This section will lay the groundwork by discussing the definition and scope of this crucial subject and providing the historical context that has shaped its evolution.
Definition and Scope
Whole genome data refers to complete sequences of an organism's DNA, comprising all its genetic information. Unlike targeted sequencing methods that focus on particular genes or areas of the genome, whole genome sequencing captures the entirety of genetic material. This includes coding regions that correspond to proteins and non-coding regions that were once considered 'junk DNA' but have turned out to hold vital regulatory roles. The scope of whole genome data is vast; it encompasses a diverse array of applications, ranging from clinical diagnostics to evolutionary studies.
The importance of whole genome data lies in its potential to identify genetic variants linked to diseases, understand genetic diversity within populations, and facilitate the development of new therapeutics. Furthermore, it provides a rich dataset that can be harnessed for various analytical techniques, including machine learning and bioinformatics tools, allowing for nuanced interpretations of data.
Historical Context
The journey to whole genome sequencing has not been a straight path. It all began in the early 1990s with the Human Genome Project, an ambitious international effort aimed at mapping the human genome. This monumental project marked the starting point for researchers worldwide, as it not only provided a reference sequence but also pioneered numerous techniques in genomic analysis.
Advances in sequencing technologies have greatly reduced the cost and time required to sequence genomes. Early methods like Sanger sequencing were labor-intensive, often taking years to yield a small amount of data. In contrast, the introduction of next-generation sequencing technologies has enabled the rapid sequencing of entire genomes, even yielding millions of sequences in a single run. This leap forward has made it feasible for scientists to generate whole genome data at an unprecedented scale.
As whole genome data became more accessible, its implications for various fields began to unfold. In medicine, a better understanding of genetic diseases allowed for more targeted treatments, while in agriculture, the data has been used to enhance crop yields and resistance to diseases. The historical context showcases a landscape of innovation and expansion, laying a solid foundation for understanding the complexities and potential of whole genome sequencing.
The Technology Behind Genome Sequencing
In the rapidly evolving field of genomics, the technology behind genome sequencing stands as a cornerstone. Understanding these technologies is vital as they shape the landscape of genomic research and its applications across various sectors, whether in medicine, agriculture, or evolutionary studies. It's not just about reading DNA; it’s the means that enable researchers to translate complex genetic information into practical knowledge.
Sequencing Methods
Genome sequencing has moved beyond the days of Sanger sequencing into more advanced methods that accelerate the pace of research and clinical applications. The two main pillars of modern genome sequencing methods include Next-Generation Sequencing and Single-Cell Sequencing.
Next-Generation Sequencing
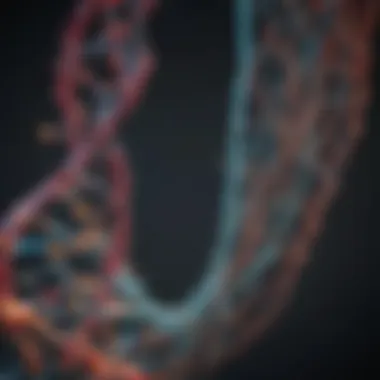
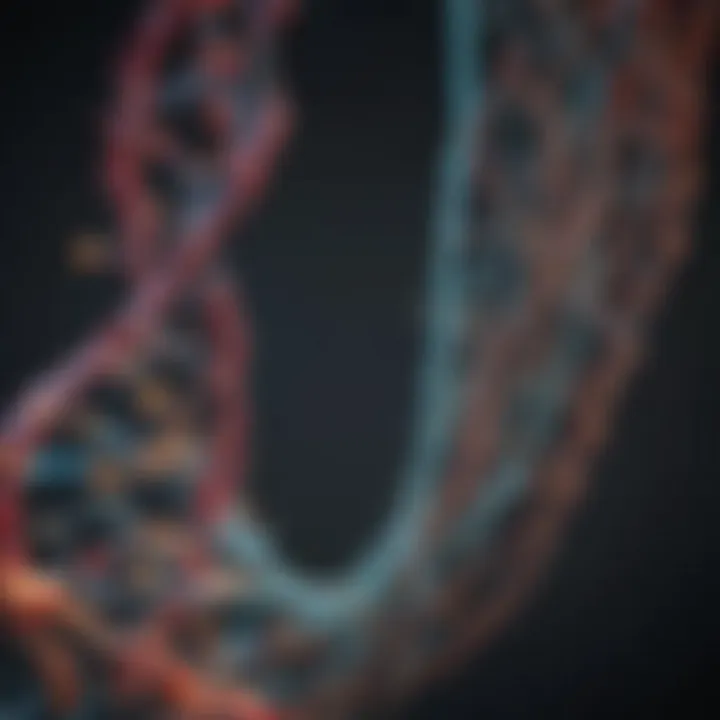
Next-Generation Sequencing (NGS) is a game changer in genomics. Its standout feature is the ability to sequence millions of fragments of DNA simultaneously. This parallel processing capability vastly reduces both time and cost, making it a preferred choice among researchers and clinicians alike.
One such benefit of NGS is its high throughput, enabling the generation of large amounts of genomic data in a fraction of the time compared to traditional methods. It could be said that NGS took the tedious out of sequencing, opening the doorway for more extensive genomic studies. However, a potential drawback is the complexity of data analysis, which necessitates robust bioinformatics tools to make sense of the vast data generated.
"The future of genomic research will heavily rely on the capabilities provided by NGS; it’s like switching from a horse and cart to a high-speed train."
Single-Cell Sequencing
Single-Cell Sequencing (SCS) has unveiled a new dimension in our understanding of genomics. Its key characteristic lies in its ability to analyze individual cells, unraveling the complexity of heterogeneous populations that traditional sequencing methods might overlook. This granularity is particularly important in understanding diseases at a cellular level, such as cancer, where the behavior and characteristics of each cell can differ.
The unique feature of SCS is its capacity to provide insights into the cellular diversity within a tissue or a tumor, shedding light on how different cells respond to treatments. Despite its advantages, SCS can be technically challenging and often requires more specialized equipment and expertise than NGS, making it less accessible for some laboratories.
Data Generation Processes
The processes underpinning data generation in genome sequencing are multifaceted. First, sample preparation, including DNA extraction and library preparation, is critical. This stage lays the groundwork for successful sequencing and subsequent analysis. Following this, the sequencing itself occurs, where the raw data is generated. Each method, whether NGS or SCS, follows its own specific protocols for data generation, each influencing the quality and type of information obtained.
Post-sequencing, the generated data must be processed, which involves aligning sequences to reference genomes and identifying genetic variants. This phase is crucial as it transforms raw data into meaningful insights that can drive research, inform clinical decisions, and contribute to advancements in personalized medicine and beyond.
Challenges in Data Storage and Management
As we plunge deeper into the world of whole genome data, one can't help but notice the significant hurdles tied to data storage and management. With advancements in sequencing technologies, the volume of data generated has grown exponentially, leading to serious implications for how this information is handled, stored, and analyzed. It's not just about having more data; it's about making sense of it, retaining its accuracy, and ensuring it is accessible to those who need it. Beneath this layer of complexity lies the importance of adopting strategic and effective storage solutions to harness the true potential of genomic data.
Volume of Data Generated
The sheer amount of data produced during whole genome sequencing can be staggering. A single human genome can produce around 100 gigabytes of raw data, and when you consider that several genomes may be sequenced simultaneously, the figures quickly spiral out of control. Let's break it down:
- Sequence complexity: Each genome contains about 3 billion base pairs of DNA sequences. The intricacies within can lead to vast amounts of information that must be preserved.
- Research scale: Large-scale studies, such as those exploring population genomics or disease associations, can easily involve thousands of samples. Multiply that by the data size of each sample, and you have a mountain of information to navigate.
- File formats: Different sequencing technologies generate diverse file formats, translating into various storage requirements. Handling formats like FASTQ, BAM, or VCF necessitates organized systems for efficient data retrieval.
With this growth comes the responsibility to ensure that data remains useful for future research and clinical applications. The challenge is not merely one of size but also encompasses organization and retrieval, demanding sophisticated management approaches.
Data Compression Techniques
To combat the problem of swelling data volumes, researchers have turned to compression techniques that can help retain essential information while minimizing storage space. Here are some noteworthy methods:
- Lossless compression: This technique maintains all original data without loss, efficiently compressing files. Tools like Gzip or Bzip2 are frequently used to achieve reductions while ensuring data integrity.
- Genomic-specific algorithms: Some algorithms, such as CRAM, are explicitly designed for genomic data. They notably reduce the size of sequencing files based on the redundancy commonly present in genomic sequences.
- Hierarchical storage management (HSM): This approach helps to automate the movement of data files across various storage devices based on the frequency of access, ensuring that less frequently needed data does not occupy more immediate storage solutions.
Incorporating these compression strategies is essential in navigating the data explosion while still retaining the richness of genomic information. However, it's a balancing act since overly aggressive compression can lead to data loss, thus undermining the results of careful sequencing work.
Cloud Storage Solutions
Cloud storage has emerged as a powerful ally in the face of these data management challenges. The flexibility and scalability of cloud systems provide a robust framework for storing large volumes of genome data. Consider the following advantages:
- Scalability: As research demands grow, cloud solutions allow for dynamic increases in storage capacity to accommodate evolving data sets without the need for hefty infrastructure investments.
- Accessibility: Cloud storage can facilitate collaborative projects, enabling researchers from various locations to access and share data seamlessly. This improves the pace of research by fostering multi-institutional cooperation.
- Security and redundancy: Leading cloud providers implement high levels of security and create backups to protect sensitive genomic information. This is particularly important for compliance with ethical and legal requirements regarding personal data.
However, while cloud solutions offer many benefits, they come with considerations about data privacy and possible reliance on internet connectivity. Researchers must navigate these waters with caution, ensuring that ethics and security remain at the forefront of genomic data management.
"The flood of sequenced genomes is like a deluge; managing it well is key to unlocking their secrets and advancing science."
Interpreting Whole Genome Data
The realm of whole genome data is not just about gathering a slew of sequences or numbers; it’s about making sense of them. Interpretation of this data is pivotal, as it transforms raw genetic information into actionable insights that can greatly influence various fields, particularly genomics and personalized medicine. With the continuous rise in the scale of genomic databases, the challenge of interpreting this information only escalates. Sophisticated tools and methods are thus integral in navigating this ocean of data, ensuring researchers can draw meaningful conclusions that might influence treatment decisions, population studies, or agricultural advancements.
Bioinformatics Tools
In the digital age, bioinformatics tools serve as the bridge between biology and data science, playing an instrumental role in the interpretation of whole genome data. The ability to analyze and visualize data effectively falls on these tools, making them essential for researchers and clinicians alike.
Genomic Data Analysis Software
Genomic data analysis software is like a Swiss Army knife for geneticists. This software specializes in sifting through the complex datasets generated by genome sequencing, making it easier for researchers to identify trends, anomalies, and meaningful insights. One notable characteristic of such software is its user-friendly interface, which allows users—ranging from seasoned researchers to those new to genomics—to navigate it with relative ease.
Moreover, a standout example is Galaxy, which provides a web-based interface for exploring bioinformatics tools without heavy programming expertise. While its accessibility is a significant plus, it indeed requires a stable internet connection and can sometimes slow down with large datasets.
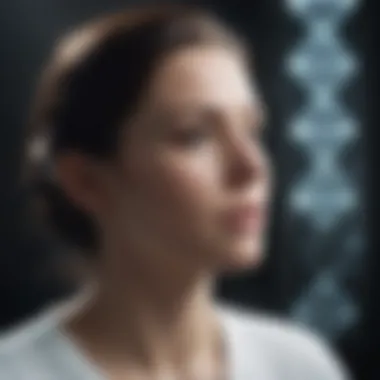
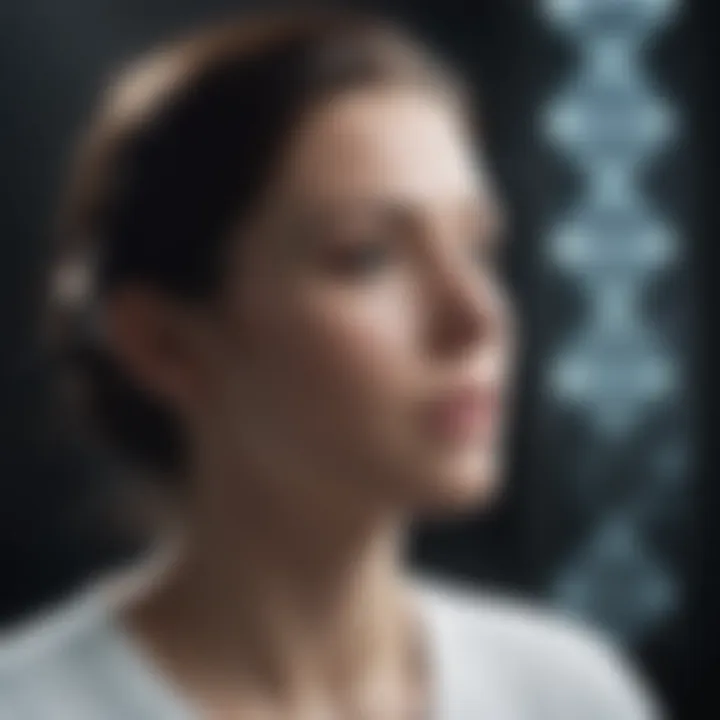
- Key Features:
- Advantages and Disadvantages:
- Intuitive design for ease of use.
- Broad selection of analytic tools tailored for various genomic tasks.
- Advantages: Great for group work and teaching. Accessible to non-specialists.
- Disadvantages: Performance can lag with high data loads.
Machine Learning Approaches
Turning the lens toward machine learning approaches, these techniques represent the cutting edge of analyzing whole genome data. They leverage algorithms to detect patterns and predict outcomes, which significantly enhances the interpretation process. One major characteristic is their adaptability; such approaches can evolve as more data becomes available, improving their accuracy over time, which is why they are increasingly popular in this scientific domain.
An intriguing aspect of machine learning is its capacity for variant prioritization. It's not just about identifying variations but understanding their relevance in a clinical context. However, using machine learning is not without its hurdles. Although it offers precise and complex analyses, there can be a steep learning curve for researchers unfamiliar with programming and statistical methods.
- Unique Features:
- Advantages and Disadvantages:
- Ability to handle vast datasets effectively and derive insights that might be missed through traditional methods.
- Advantages: Can automate time-consuming tasks and improve prediction models.
- Disadvantages: Requires substantial computational resources and expertise in interpreting the model outputs.
Variant Calling and Annotation
When it comes to variant calling and annotation, the subtleties in genomic data interpretation come to the fore. This process involves identifying differences between the sequenced genomes and a reference genome. Each identified variant can hold the key to understanding a range of genetic disorders, hence accuracy in this phase is paramount.
Each variant must then be annotated, which effectively tags it with relevant information, such as potential impacts on proteins or associations with diseases. This detailed tagging becomes invaluable in the clinical sphere, where precise knowledge about genetic variants can significantly inform treatment strategies.
"Variant calling isn’t just about finding differences; it’s about understanding their implications."
Applications of Whole Genome Data
The growing field of whole genome data has far-reaching applications that stretch across various domains, particularly in medicine, research, and agriculture. Understanding these applications is critical, as they reveal how genomic insights are revolutionizing traditional practices and leveraging data for innovation and advancements in health and science. Whole genome data can be likened to a treasure map; each data point offers clues related to genetic predispositions, disease markers, and potential therapeutic targets. Let's delve deeper into some of the distinctive applications that highlight the significance of this vast reservoir of genomic information.
Personalized Medicine
In the realm of personalized medicine, whole genome data has become indispensable. By sequencing and analyzing an individual's genome, healthcare providers can tailor treatments based on a patient's unique genetic makeup. This approach marks a shift from the traditional one-size-fits-all model, allowing for more specific interventions. For example, patients with specific cancer mutations might respond better to specific therapies, such as targeted treatments like imatinib for chronic myeloid leukemia.
Furthermore, the probe into pharmacogenomics—the study illustrating how genes affect a person's response to drugs—illustrates the practical utility of this data. Here are a few key benefits of personalized medicine through genome data:
- Tailored Treatments: Reduces trial-and-error prescribing.
- Predictive Capability: Identifies individuals at risk for specific diseases.
- Enhanced Drug Development: Facilitates faster and more efficient trials for new therapies.
"The key to unlocking the benefits of personalized medicine lies in recognizing the unique genetic code of each patient."
This shift is not without its challenges, though. Ethical concerns regarding privacy and potential discrimination based on genetic information loom large, necessitating a careful balance in the integration of whole genome data into everyday clinical practice.
Population Genomics Studies
Whole genome data also plays a pivotal role in population genomics studies. Researchers utilize this information to explore genetic variations across populations, which can help elucidate how certain traits or diseases are distributed geographically or culturally. The insights derived can lead to better healthcare strategies that are more effective on a population level.
Key considerations include:
- Tracking Disease Outbreaks: Genomic data can help trace how diseases spread within and between populations.
- Understanding Evolutionary Trends: By comparing genomes, scientists can gain insights into how populations have evolved over time. For example, studies on human adaptations to high altitudes in regions like the Himalayas can reveal important genetic variations relevant to survival.
- Public Health Planning: The data can guide the allocation of resources and the design of targeted health interventions.
Agricultural Biotechnology
Finally, in the realm of agricultural biotechnology, whole genome data is revolutionizing crop and livestock development. By understanding the genetic underpinnings of desired traits such as yield, pest resistance, or drought tolerance, scientists can engineer better-performing crops and livestock.
Important aspects include:
- Genetic Modification: Whole genome information aids in the development of genetically modified organisms (GMOs) that can thrive in diverse conditions and resist disease.
- Marker-Assisted Selection: This technique accelerates the breeding process by selecting plants or animals with desired traits based on their genomic data, rather than solely relying on physical traits.
- Sustainability Practices: Improved crop varieties can help to minimize the environmental impact, ensuring food security amid changing climate conditions.
In summary, whole genome data engenders radical transformations in diverse arenas, from personal health optimization to meticulous ecological management. As we continue to unpack the segmental layers of genomic information, its relevance only intensifies, expanding the horizon of what is possible in bioscience.
Ethical Considerations in Whole Genome Research
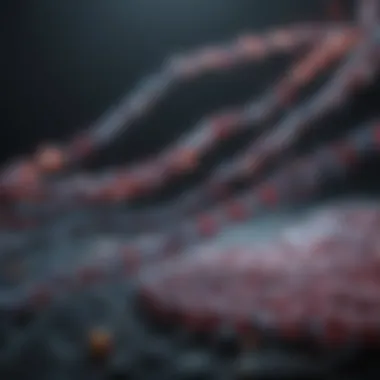
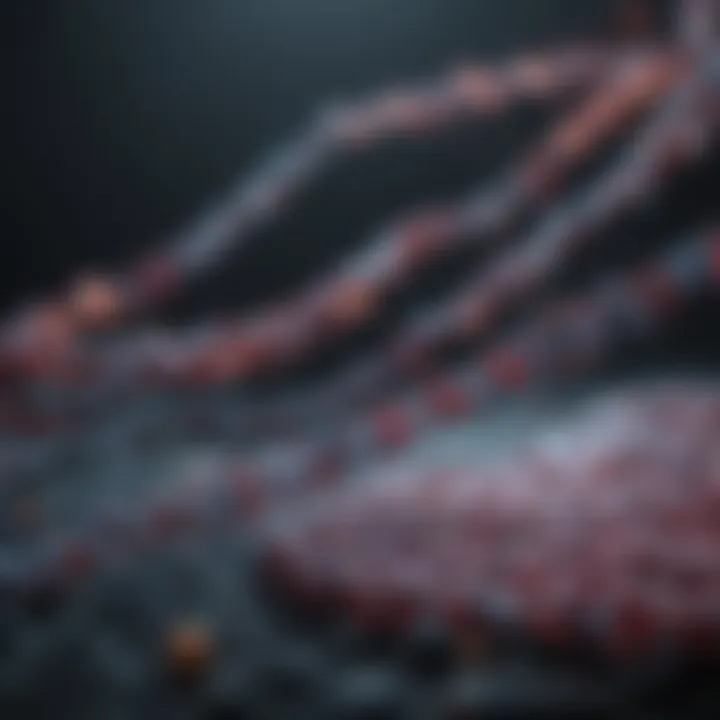
Exploring the ethical landscape of whole genome sequencing is paramount, especially as we navigate 21st-century advancements in genomics. As these technologies leap forward, they pose unique benefits, notably in health and medicine. However, they also bring about complexities that need thorough examination. The topic doesn't just brush the surface but digs deep into the implications for individuals, societies, and policy-makers.
Privacy and Consent
At the heart of ethical considerations in genetic research lies the issue of privacy. Given that whole genome sequencing reveals intricate details about an individual's genetic makeup, the potential for misuse of this information is a serious concern. Patients and research subjects stand to relinquish sensitive data, ranging from predispositions to specific diseases to familial lineage.
Consent is another critical pillar in the discussion. It is not enough for individuals to sign their names on a document; they must fully grasp what they are agreeing to. Informed consent should mean providing clear, accessible information on how their genomic data will be used, who it may be shared with, and the risks involved. Insufficient understanding may lead individuals to consent to data usage that could have unforeseen consequences later on. Therefore, researchers and institutions must invest in tailored communication strategies that demystify genetic research to foster trust.
"In a world where data is king, consent cannot be viewed as a mere box to be checked; it must be an ongoing dialogue."
Ensuring that privacy measures are in place is also crucial. Researchers and organizations responsible for genomic data must implement robust security protocols. This involves using advanced encryption methods and ensuring that only authorized individuals have access to sensitive information. Failure to protect this data can have devastating impacts, not only for individuals but also for the credibility of the research field.
Data Ownership Issues
The notion of ownership in genomic data is an enigma. Does the individual whose DNA is being sequenced maintain ownership of that data once it is processed? This question could lead to intricate disputes that echo throughout ethical, legal, and social domains.
Educators and legal professionals are becoming increasingly involved in navigating these murky waters. Some argue that individuals should have full ownership and control of their genomic information, including the right to dispose of it or limit its access. On the flip side, institutions may claim rights over this data based on their investment in research.
The balance between individual rights and institutional needs is delicate. Clear guidelines or policies must be established to prevent exploitation or data commodification. Furthermore, transparency is key; individuals should be informed whether their genomic data could contribute to commercial products or further research without their explicit consent.
In summary, as we tread towards a future dominated by genomic advancements, ethical considerations are not just an afterthought; they are central to the integrity and future of genomic research. Balancing privacy, consent, and ownership while encouraging innovation will ensure that the journey forward respects individual rights and societal norms.
Future Directions for Whole Genome Data
The exploration of whole genome data has reached a pivotal point, with implications that go far beyond what was previously imaginable. As we step into a new era of genomics, several exciting trends and innovations shape the landscape of genetic research and its applications. These future directions, particularly in emerging technologies and clinical implementations, are crucial to understanding how whole genome data can drive advancements in personalized medicine, agricultural biotechnology, and even public health initiatives.
Emerging Technologies
Third-Generation Sequencing
Third-generation sequencing technology is making waves in the field of genomics. It focuses on real-time sequencing, allowing researchers to gather data directly from the biological sample without the need for amplification. This characteristic significantly shortens the time required for sequencing and enhances throughput. One of the most notable aspects of third-generation sequencing is its ability to produce long reads. This longevity allows researchers to assemble genomes more accurately, as they can span across complex regions that shorter reads might misinterpret.
However, while this technology is a game changer, it comes with its own set of challenges. The error rates in these long reads can be higher compared to other sequencing methods. Despite this drawback, the potential for high-quality genomic data makes third-generation sequencing a strong contender for future research endeavors, especially when optimal accuracy is vital.
Improvements in Data Processing
As genomic data continues to grow exponentially, improvements in data processing are becoming paramount. Algorithms and computational methods have advanced, enabling researchers to analyze and interpret vast datasets more efficiently. One key characteristic of data processing advancements is parallel computing, which allows simultaneous analysis of multiple datasets. This is particularly beneficial in large genomics studies, where speed is crucial.
The unique feature of these improvements lies in the integration of machine learning approaches. By training algorithms on existing data, researchers can develop models that predict variants and assess their implications quickly. However, reliance on such methods comes with caution, as misinterpretation due to algorithmic bias can lead to misleading conclusions. Yet, when applied correctly, improved data processing facilitates a more nuanced understanding of genomic data, ideally enhancing the predictive capabilities in clinical settings.
Implementation in Clinical Settings
The transition of whole genome data from research laboratories to clinical applications marks a significant turning point. As healthcare systems begin to integrate genomic data into routine practice, several benefits and considerations arise. One of the notable advantages is the potential for tailored treatment strategies. By analyzing a patient’s unique genomic profile, healthcare providers can select therapies that are more likely to be effective based on individual genetic makeup. This shift toward personalized medicine not only enhances treatment efficacy but also minimizes adverse effects.
Yet, the implementation of whole genome data in clinical settings raises important questions surrounding regulation and data privacy. As we embrace these advancements, it's essential to ensure that patient consent, data protection, and ethical guidelines are robustly established. The future of whole genome data hinges on striking a balance between technological innovation and ethical responsibility.
"The promise of whole genome data comes with the challenge of ensuring that its use aligns with the highest standards of ethics and patient care."
In summary, the future directions for whole genome data present both opportunities and challenges. Emerging technologies like third-generation sequencing and improvements in data processing will continue to push the boundaries of what’s possible in genomics. However, it’s the implementation in clinical settings that will truly determine how effectively this knowledge translates into tangible benefits for patients and society as a whole.
The End
The conclusion serves as the anchor point of this article, emphasizing the importance of whole genome data in shaping the current landscape of genomics. As we dissect the ramifications of the insights and implications surrounding this topic, one can't help but recognize the transformative potential that whole genome data holds in various fields, from medicine to public health policies. It is not merely a collection of sequences; it is a repository of information with the power to redefine treatment protocols and improve health outcomes through personalized approaches.
Summation of Key Insights
The discussion throughout this article has brought to light several pivotal insights:
- Technological Advances: The rapid evolution of sequencing technologies has made genome data more accessible, enabling researchers to harness this information for groundbreaking studies.
- Data Challenges: Significant challenges lie in the management and storage of the vast amounts of data generated, pushing the envelope on existing data compression techniques and cloud storage solutions.
- Ethical Implications: The ethical dimensions surrounding privacy and data ownership cannot be overlooked. As data-sharing practices evolve, legal frameworks must also adapt to protect individual rights while fostering innovation in research.
- Applications in Health: The potential applications in personalized medicine and public health illustrate a broader implication on how whole genome data can inform and enhance preventative and therapeutic strategies.
These key points underscore the necessity for continued exploration and dialogue about whole genome data, highlighting both its opportunities and responsibilities stakeholders must address.
Final Thoughts on Whole Genome Data
In closing, whole genome data encapsulates a wealth of knowledge that synthesizes biological complexity into usable information for real-world applications. As various sectors, including healthcare, agriculture, and legal domains, begin to understand and implement these advancements, the overarching goal should remain clear: to use this data to benefit society while ensuring ethical standards are met.
The conversation surrounding whole genome data is still growing. It invites a collaborative effort amongst researchers, policymakers, and the public to navigate this multifaceted landscape. As we stand on this precipice of innovation, the path forward looks promising, but not without challenges. It's crucial that as we push the boundaries of science and technology, we maintain a balanced approach that honors the ethical considerations that ought to underpin our ambitions. Embracing whole genome data is less about harnessing power and more about enhancing human health and understanding our shared biology in a responsible manner.